01 Beginner
02 intermediate, 03 advanced, 04 training programs, knowledge representation in ai - types, issues, & techniques, artificial intelligence certification course, knowledge representation in ai - overview, what is knowledge representation in ai, types of knowledge in ai, 1.) declarative knowledge, 2.) procedural knowledge, 3.) meta knowledge, the knowledge cycle in ai, approaches to knowledge representation in ai, techniques of knowledge representation in ai, advantages and limitations of different techniques of knowledge representation, logic-based methods, semantic networks, scripts and frames, real-world applications of knowledge representation in ai, challenges and future directions in knowledge representation in ai, best practices of knowledge representation in ai, resources for further learning and practice, live classes schedule.
Filling Fast | |
Filling Fast | |
Filling Fast | |
Filling Fast | |
Filling Fast | |
Filling Fast | |
Filling Fast | |
Filling Fast | |
Filling Fast | |
Filling Fast |

About Author
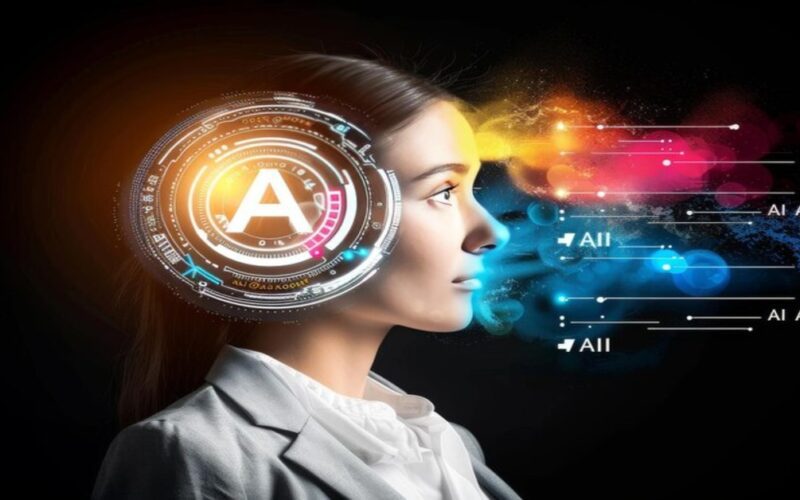
What is Knowledge Representation in AI?
- 7 minute read
- August 27, 2024
Written by:

I am Julie Bowie a data scientist with a specialization in machine learning. I have conducted research in the field of language processing and has published several papers in reputable journals.
Summary: Knowledge representation is a core concept in AI, focusing on structuring information for machines to reason and learn. This blog covers common representation methods, their importance, implications, and recent advancements. It also provides practical tips for choosing the right representation and maintaining knowledge effectively in AI systems.
Introduction
Knowledge representation is a fundamental concept in Artificial Intelligence (AI) that focuses on how information and knowledge can be structured so that machines can understand and use it effectively. Just as humans use language and symbols to convey ideas, AI systems need a way to represent knowledge in a format that they can process.
This blog will explore what knowledge representation is, its importance in AI, various approaches, and the implications of these methods.
What is Knowledge Representation?
Knowledge representation involves creating models and structures to represent information in a way that intelligent systems can use. The primary goal is to enable machines to reason about the world like humans, capturing and encoding knowledge in a format that can be easily processed and utilized by AI systems.
In simpler terms, knowledge representation is about translating human knowledge into a form that computers can understand. This allows AI systems to perform tasks such as problem-solving, decision-making, and learning from experiences.
Types of Knowledge
Before diving deeper into the methods of knowledge representation, it’s essential to understand the different types of knowledge that AI systems need to represent:
Declarative Knowledge: This includes facts and concepts that can be stated explicitly, such as “Paris is the capital of France.”
Procedural Knowledge: This refers to knowledge about how to perform tasks, such as “how to bake a cake.”
Meta-Knowledge: This is knowledge about knowledge, such as understanding the limitations of certain information or knowing how to acquire new knowledge.
Heuristic Knowledge: This involves rules of thumb or strategies that guide decision-making based on experience.
Structural Knowledge: This describes the relationships between different concepts and objects.
Understanding these types of knowledge helps in designing effective representation systems.
How Knowledge Representation Works
Knowledge representation works by structuring information in a way that allows AI systems to reason and draw conclusions. There are various approaches to achieving this, each with its strengths and weaknesses. Here are some common methods:
Logical Representation
Logical representation uses formal languages to express knowledge. It involves creating statements that can be true or false. For example, using propositional logic, we can represent facts like “If it rains, then the ground will be wet.” This method allows AI systems to make inferences based on logical rules.
Semantic Networks
Semantic networks represent knowledge through a graph structure, where nodes represent concepts or objects, and links represent relationships between them.
For example, a semantic network might show that a “dog” is a type of “animal,” and “barks” is an action associated with “dog.” This approach helps in visualizing relationships and can be useful for natural language processing.
Frames are data structures that hold knowledge about a particular concept or object. They include attributes and values, allowing for the organization of information in a structured way.
For example, a frame for a “car” might include attributes like “make,” “model,” “year,” and “color.” Frames help in capturing complex information about objects and their properties.
Ontologies provide a formal specification of concepts, properties, and relationships within a specific domain. They define a shared vocabulary and allow for consistent communication between systems.
For instance, an ontology for the medical domain might include concepts like “disease,” “symptom,” and “treatment,” along with their relationships. Ontologies are essential for knowledge sharing and interoperability among AI systems.
Neural Networks
Neural networks represent knowledge through patterns and connections between nodes. They are particularly useful for learning from data and making predictions.
For example, in image recognition, a neural network can learn to identify objects by adjusting the weights of connections based on training data. This approach is powerful for tasks that involve large amounts of unstructured data.
The Importance of Knowledge Representation in AI
In this section, we will explore the significance of knowledge representation in Artificial Intelligence, highlighting how it enables reasoning, enhances learning, facilitates communication, and supports informed decision-making in AI systems. Knowledge representation is crucial for several reasons:
Enabling Reasoning
One of the main purposes of knowledge representation is to enable reasoning. By structuring knowledge in a way that AI systems can understand, we allow them to draw conclusions, make predictions, and solve problems based on the information they have.
Facilitating Learning
Effective knowledge representation supports Machine Learning by providing a framework for AI systems to learn from data. When knowledge is represented clearly, AI systems can identify patterns, make inferences, and adapt to new information.
Enhancing Communication
Knowledge representation allows AI systems to communicate with humans and other systems. By using a common representation, AI can share information more effectively, leading to better collaboration and understanding.
Supporting Decision-Making
In complex scenarios, knowledge representation helps AI systems make informed decisions . By representing relevant knowledge, AI can evaluate options, weigh risks, and choose the best course of action.
Implications of Knowledge Representation
This section will examine the implications of knowledge representation in Artificial Intelligence, focusing on the trade-offs between expressiveness and efficiency, the challenges of handling uncertainty, and the importance of scalability in knowledge systems. The way knowledge is represented in AI has significant implications for its performance and capabilities:
Expressiveness vs. Efficiency
One of the key trade-offs in knowledge representation is between expressiveness and efficiency. Highly expressive representations can capture complex relationships and nuances but may be computationally expensive to process. Conversely, simpler representations may be more efficient but might not capture all necessary details.
Handling Uncertainty
Real-world knowledge often involves uncertainty and ambiguity. Effective knowledge representation must account for this uncertainty, allowing AI systems to make decisions even when information is incomplete or imprecise. Techniques like fuzzy logic and probabilistic reasoning are often employed to handle uncertainty.
Scalability
As the amount of knowledge grows, the representation system must be scalable. It should be able to accommodate new information without becoming overly complex or difficult to manage. This is particularly important in dynamic environments where knowledge is constantly changing.
Workarounds and Alternatives
Given the challenges associated with knowledge representation, researchers and practitioners have developed various workarounds and alternatives:
Hybrid Approaches
Many AI systems use hybrid approaches that combine different methods of knowledge representation. For example, a system might use semantic networks for representing relationships and logical representation for reasoning. This allows for greater flexibility and effectiveness in handling diverse types of knowledge.
Knowledge Graphs
Knowledge graphs are a powerful way to represent knowledge in a structured format. They consist of nodes (entities) and edges (relationships), enabling complex queries and reasoning. Knowledge graphs have become popular in applications like search engines and recommendation systems, where understanding relationships is crucial.
Reinforcement Learning
In scenarios where traditional knowledge representation may struggle, reinforcement learning offers an alternative. This approach allows AI systems to learn from interactions with their environment, making decisions based on rewards and penalties rather than predefined knowledge structures.
Recent Developments and Future Directions
This section will discuss recent advancements in knowledge representation within AI, exploring emerging techniques, integration with Machine Learning, and future directions that promise to enhance AI capabilities and applications.
Advances in Natural Language Processing
Natural Language Processing (NLP) has made significant strides in representing knowledge from unstructured text. Techniques like transformer models enable AI systems to understand and generate human language, allowing for more intuitive interactions and knowledge extraction.
Improved Ontology Development
The development of ontologies has become more streamlined with tools and frameworks that facilitate the creation and maintenance of formal knowledge structures. This has enhanced interoperability and knowledge sharing across different AI systems.
Integration with Machine Learning
Knowledge representation is increasingly being integrated with Machine Learning techniques. This allows AI systems to leverage structured knowledge while also learning from data, leading to more robust and adaptable models.
Practical Tips and Best Practices
In this section, we will provide practical tips and best practices for effective knowledge representation in AI, including choosing the right methods, maintaining consistency, and ensuring adaptability to enhance system performance and usability. When working with knowledge representation in AI, consider the following tips:
Choose the Right Representation
Select the most appropriate representation method based on the type of knowledge and the intended application. Consider factors like expressiveness, efficiency, and ease of use.
Keep It Simple
Avoid unnecessary complexity in your knowledge representation. A simpler representation is often easier to understand and manage, making it more effective for reasoning and decision-making.
Ensure Consistency
Maintain consistency in your knowledge representation to avoid confusion and errors. Use standardized formats and terminologies to ensure that all stakeholders can understand and use the knowledge effectively.
Regularly Update Knowledge
Knowledge representation should be dynamic and adaptable. Regularly review and update your knowledge structures to reflect new information and changes in the domain.
Collaborate with Experts
Engage with domain experts when developing knowledge representations. Their insights can help ensure that the representation accurately reflects the complexities of the knowledge domain.
Knowledge representation is a vital aspect of Artificial Intelligence, enabling machines to understand, reason, and learn from information in a human-like manner. By structuring knowledge effectively, AI systems can perform various tasks, from problem-solving to decision-making, and interact with humans more intuitively.
Understanding the different approaches to knowledge representation, including logical representation, semantic networks, frames, ontologies, and neural networks, is essential for developing effective AI systems. As the field continues to evolve, advancements in natural language processing, ontology development, and integration with Machine Learning will further enhance the capabilities of knowledge representation in AI.
By following best practices and staying informed about recent developments, developers and researchers can create AI systems that leverage knowledge representation to achieve intelligent behaviour and solve complex real-world problems.
Frequently Asked Questions
What is the main purpose of knowledge representation in ai.
The main purpose of knowledge representation in AI is to structure information in a way that machines can understand and use it effectively for reasoning, decision-making, and learning.
What Are Some Common Methods of Knowledge Representation?
Common methods of knowledge representation include logical representation, semantic networks, frames, ontologies, and neural networks. Each method has its strengths and is suited for different types of knowledge.
How Does Knowledge Representation Impact AI Performance?
Knowledge representation impacts AI performance by determining how effectively a system can reason about information, make decisions, and learn from data. A well-designed representation can enhance an AI system’s capabilities and efficiency.
Reviewed by:
Post written by: Julie Bowie
Data Science Data Collection: A Comprehensive Guide
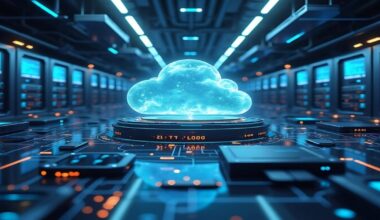
Cloud Computing Serverless Computing: An In-depth Analysis
You may also like.
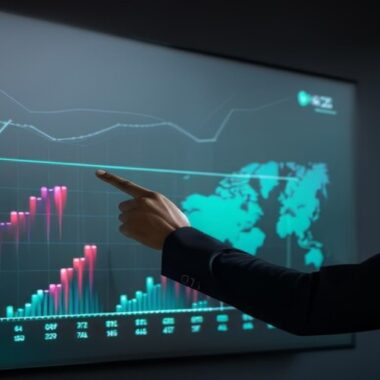
- Artificial Intelligence
Artificial Intelligence Courses in India after 12th
- Aashi Verma
- June 20, 2023

AI in the Next 5 Years: A Glimpse into the Future
- July 14, 2023
- 6 minute read
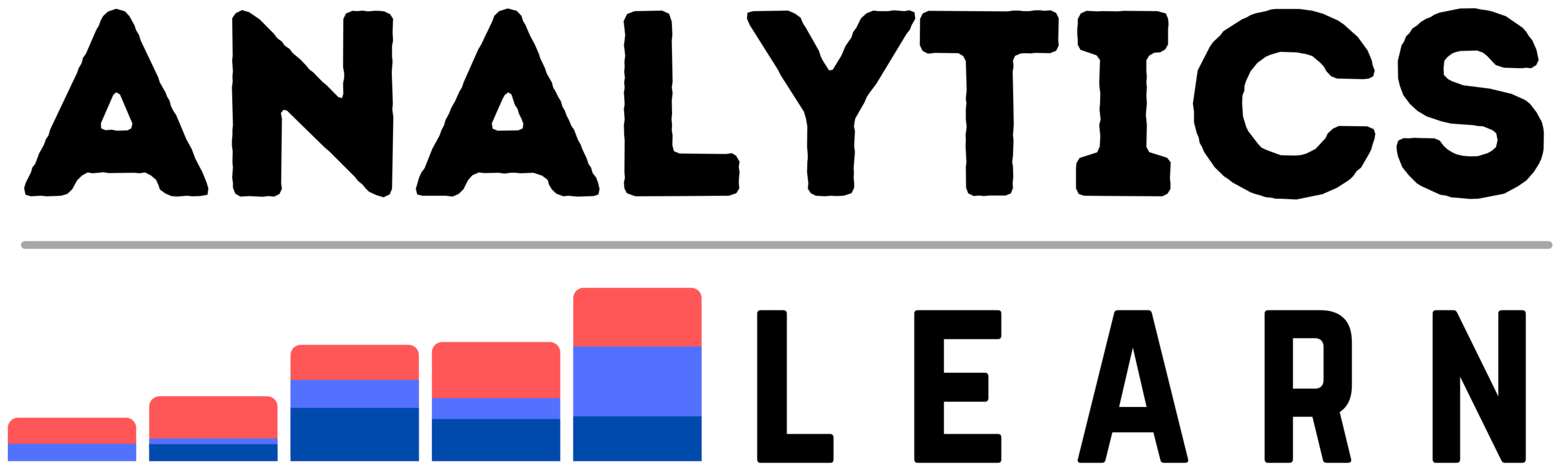
Knowledge Representation in AI: Ultimate Guide
In this guide, we will explore Knowledge Representation in AI with the Concepts, Methods, and Applications in detail.
Knowledge representation is a critical aspect of artificial intelligence (AI) that involves the way in which information and rules are structured so that machines can understand, reason, and make decisions.
It bridges the gap between human cognitive processes and computer algorithms, enabling machines to mimic human-like understanding and problem-solving abilities.
This article explores the various techniques and methods used in knowledge representation, their applications, and provides illustrative examples to highlight their importance.
What is Knowledge Representation in AI?
Knowledge representation is the method by which information is formalized for AI systems to use.
It encompasses a variety of techniques designed to represent facts, concepts, and relationships within a domain, allowing machines to process and utilize this information effectively.
The primary goals of knowledge representation include:
- Expressiveness: The ability to represent a wide variety of knowledge.
- Efficiency: The capability to manipulate and reason with knowledge quickly.
- Understandability: The ease with which humans can comprehend the represented knowledge.
- Scalability: The ability to handle increasing amounts of knowledge without significant performance degradation.
Types of Knowledge
Knowledge in AI can be categorized into several types:
- Declarative Knowledge: Facts and information about objects, events, and their relationships. For example, “Paris is the capital of France.”
- Procedural Knowledge: Knowledge of how to perform tasks. For example, “How to ride a bicycle.”
- Meta-Knowledge: Knowledge about other knowledge. For example, “The reliability of a source.”
- Heuristic Knowledge: Rules of thumb or best practices. For example, “If the weather is cloudy, it might rain.”
Methods of Knowledge Representation
Several methods are employed to represent knowledge in AI, each with its strengths and weaknesses. These methods include:
1. Semantic Networks
Semantic networks are graph structures consisting of nodes (representing concepts) and edges (representing relationships). They are useful for representing hierarchical and associative knowledge.
Example: Consider a semantic network for animal classification:
- Nodes: “Animal,” “Mammal,” “Bird,” “Dog,” “Cat,” “Sparrow”
- Edges: “is-a” (Dog is a Mammal), “has-a” (Dog has a Tail)
This network helps in understanding relationships and inheritance of properties (e.g., if “Dog” is a “Mammal” and “Mammals” have “Warm-blood,” then “Dog” is warm-blooded).
Frames are data structures for dividing knowledge into substructures by representing stereotyped situations.
They consist of slots (attributes) and values.
Example: A frame for a “Car” might include:
- Make: Toyota
- Model: Corolla
Frames allow AI systems to organize knowledge into recognizable patterns, making it easier to retrieve and use.
3. Rule-Based Systems
Rule-based systems use a set of if-then rules to represent knowledge.
These systems are particularly effective in decision-making and problem-solving applications.
Example: A medical diagnosis system might use rules like:
- IF the patient has a fever AND a cough, THEN diagnose as flu.
- IF the patient has a rash AND itching, THEN diagnose as allergy.
Rule-based systems are straightforward to implement and interpret, making them widely used in expert systems.
4. Ontologies
Ontologies define a set of representational terms and the relationships among them, often using languages like OWL (Web Ontology Language).
They provide a more rigorous and standardized way to represent knowledge.
Example: An ontology for a university might include classes such as “Student,” “Professor,” “Course,” with properties like “teaches,” “enrolled-in,” and relationships such as “Student is-enrolled-in Course.”
Ontologies are particularly useful in ensuring interoperability and sharing of knowledge across different systems and domains.
5. Logic-Based Representations
Logic-based representations use formal logic to encode knowledge.
Propositional logic and first-order predicate logic are common types.
Example: In propositional logic, knowledge might be represented as:
- P: “It is raining.”
- Q: “The ground is wet.”
- Rule: P → Q (If it is raining, then the ground is wet.)
These representations are powerful for performing automated reasoning, as they allow for precise and unambiguous expression of knowledge.
6. Probabilistic Representations
Probabilistic representations incorporate uncertainty into knowledge representation, allowing AI systems to make decisions under uncertainty.
Bayesian networks are a common form of probabilistic representation.
Example: A Bayesian network for a medical diagnosis might include variables such as “Fever,” “Cough,” “Flu,” with conditional probabilities indicating the likelihood of having the flu given the presence of symptoms.
Probabilistic representations are essential for real-world applications where uncertainty is a significant factor.
Applications of Knowledge Representation
Knowledge representation techniques are applied in various AI applications to enhance their functionality and effectiveness.
1. Natural Language Processing (NLP)
In NLP, knowledge representation helps in understanding and generating human language.
Semantic networks and ontologies, for example, enable machines to grasp the meanings of words and their relationships, facilitating tasks like machine translation and sentiment analysis.
Example: Google’s Knowledge Graph uses a vast ontology to understand search queries better and provide more relevant results by connecting facts about people, places, and things.
2. Expert Systems
Expert systems use rule-based knowledge representation to emulate the decision-making abilities of human experts.
They are widely used in domains like medical diagnosis, financial forecasting, and technical support.
Example: MYCIN, an early expert system for diagnosing bacterial infections and recommending antibiotics, used over 450 rules to make its recommendations based on symptoms and test results.
3. Robotics
In robotics, knowledge representation is crucial for understanding and navigating the environment.
Frames and semantic networks help robots recognize objects, understand spatial relationships, and plan actions.
Example: A robot vacuum cleaner uses a map of the house (a form of knowledge representation) to navigate and clean efficiently, avoiding obstacles and covering all areas.
4. Autonomous Vehicles
Autonomous vehicles rely on knowledge representation to interpret sensor data, make driving decisions, and navigate safely.
Probabilistic representations and logic-based systems are used to model uncertainties and ensure robust decision-making.
Example: Waymo’s autonomous vehicles use a combination of sensors, machine learning, and knowledge representation techniques to understand the driving environment and make real-time decisions.
In video games, knowledge representation allows non-player characters (NPCs) to exhibit intelligent behavior, enhancing the gaming experience.
Rule-based systems and state machines are commonly used for this purpose.
Example: In strategy games like “StarCraft,” AI opponents use knowledge representation to plan and execute strategies, adapting to the player’s actions and the game’s evolving state.
Additional Applications of Knowledge Representation
Here are the few Additional Applications of Knowledge Representation in AI:
1. Cognitive Computing
Cognitive computing systems, such as IBM’s Watson, use knowledge representation to process and analyze vast amounts of unstructured data, such as medical records, research papers, and news articles.
By integrating multiple forms of knowledge representation, these systems can understand context, derive insights, and assist in decision-making.
Example: Watson for Oncology uses natural language processing (NLP) and ontologies to help oncologists by providing evidence-based treatment options. It analyzes patient records and medical literature to suggest personalized treatment plans.
2. Personal Assistants
Digital personal assistants like Siri, Alexa, and Google Assistant rely heavily on knowledge representation to understand user queries and provide relevant responses.
They use ontologies and semantic networks to interpret the context and relationships between entities.
Example: When a user asks, “What’s the weather like in New York?” the assistant interprets “weather” and “New York” through a knowledge base that includes geographic and meteorological data, providing accurate weather information.
3. Fraud Detection
In financial services, AI systems use rule-based systems and probabilistic models to detect fraudulent activities.
These systems analyze transaction patterns, user behavior, and historical data to identify anomalies and potential fraud.
Example: A fraud detection system might use rules like:
- IF a transaction exceeds $10,000 AND the account is flagged for unusual activity, THEN alert for potential fraud.
- IF transactions occur from multiple locations in a short time span, THEN flag for review.
4. Knowledge Graphs
Knowledge graphs, which are a form of semantic network, are used by search engines and recommendation systems to improve information retrieval and personalization.
Example: Google’s Knowledge Graph enhances search results by connecting related concepts and providing comprehensive information about a topic. If you search for “Leonardo da Vinci,” the knowledge graph provides details about his biography, works, and related historical figures.
Latest Advancements and Future Trends
Here are the few Latest Advancements and Future Trends in AI:
1. Hybrid Approaches
Combining symbolic AI (logic-based, rule-based systems) with sub-symbolic AI (neural networks, machine learning) is a promising trend.
These hybrid approaches aim to leverage the strengths of both paradigms: the interpretability and structured knowledge of symbolic AI with the learning capabilities and pattern recognition of sub-symbolic AI.
Example: Neuro-symbolic AI systems use neural networks to process raw data and extract features, which are then used by symbolic reasoning engines to make decisions or generate explanations. This integration can enhance the robustness and explainability of AI systems.
2. Transfer Learning
Transfer learning allows AI models to transfer knowledge gained from one domain to another, improving learning efficiency and performance.
In knowledge representation, this involves reusing ontologies, semantic networks, or trained models across different applications.
Example: A model trained to recognize objects in images can transfer its knowledge to a new task, such as identifying objects in videos, reducing the amount of training data required.
3. Explainable AI (XAI)
Explainable AI focuses on making AI systems’ decision-making processes transparent and understandable to humans.
This is crucial for building trust and ensuring ethical AI deployment. Knowledge representation techniques play a key role in achieving explainability.
Example: An explainable AI system might use a combination of rule-based reasoning and visualizations to show how it arrived at a particular decision, such as a loan approval or medical diagnosis.
4. Knowledge Graph Embeddings
Knowledge graph embeddings are a technique to represent entities and relationships in a knowledge graph as vectors in a continuous vector space.
This facilitates the application of machine learning techniques to knowledge graphs, enabling tasks like link prediction, entity classification, and knowledge graph completion.
Example: Embedding techniques like TransE, RotatE, and ComplEx have been developed to improve the representation and reasoning capabilities of knowledge graphs, leading to more accurate and scalable AI systems.
5. Integration with IoT
The integration of knowledge representation with the Internet of Things (IoT) enables more intelligent and context-aware IoT systems.
These systems can reason about the data collected from various sensors and devices, providing more meaningful insights and actions.
Example: In smart homes, a knowledge representation system can combine data from temperature sensors, motion detectors, and user preferences to optimize heating and lighting, improving energy efficiency and user comfort.
Challenges and Future Directions
Despite its successes, knowledge representation in AI faces several challenges:
- Scalability: As the amount of knowledge grows, efficiently storing, retrieving, and reasoning becomes challenging.
- Ambiguity and Uncertainty: Representing and reasoning with ambiguous or uncertain information remains a difficult problem.
- Integration: Combining different representation methods and integrating them into a cohesive system can be complex.
- Explainability: Ensuring that AI systems can explain their decisions and reasoning processes is crucial for trust and transparency.
Future directions in knowledge representation research aim to address these challenges by developing more scalable, robust, and interpretable methods.
Advances in areas like deep learning, hybrid systems, and neurosymbolic AI (which combines neural networks with symbolic reasoning) hold promise for more sophisticated and effective knowledge representation.
Knowledge representation is a cornerstone of artificial intelligence, enabling machines to process, reason, and act upon information in ways that mimic human intelligence.
From semantic networks to probabilistic models, the variety of techniques available provides powerful tools for different applications, from natural language processing to autonomous vehicles.
As AI continues to evolve, advances in knowledge representation will play a crucial role in building more intelligent, capable, and trustworthy systems.
Understanding and leveraging these methods will be essential for anyone involved in the development and application of AI technologies.
Related Article: Top 15 Generative AI Projects: Ultimate Guide
Meet Nitin, a seasoned professional in the field of data engineering. With a Post Graduation in Data Science and Analytics, Nitin is a key contributor to the healthcare sector, specializing in data analysis, machine learning, AI, blockchain, and various data-related tools and technologies. As the Co-founder and editor of analyticslearn.com, Nitin brings a wealth of knowledge and experience to the realm of analytics. Join us in exploring the exciting intersection of healthcare and data science with Nitin as your guide.
Related Posts
Which case would benefit from explainable ai principles, image recognition in artificial intelligence: complete guide, top 20 application of artificial intelligence in healthcare., top 10 ai tools for content writing, top 15 artificial intelligence courses: ai courses, open ai playground: ultimate guide, top 15 generative ai projects: ultimate guide, artificial intelligence movies | top 10 ai movies, where is artificial intelligence taking the future.

Knowledge Representation in AI Explained in Simple Terms

Artificial intelligence (AI) is a popular and innovative technology that takes human intelligence to the next level. It offers the power of accurate intelligence integrated with machines.
Humans are bestowed with high-level thinking, reasoning, interpreting, and understanding of knowledge. The knowledge we gain helps us perform different activities in the real world.
Nowadays, even machines are becoming capable of doing so many things, thanks to technology.
Recently, the usage of AI-powered systems and devices is rising due to their efficiency and accuracy in performing complex tasks.
Now, the problem is, while humans have acquired many levels and types of knowledge in their lives, machines face difficulty in interpreting the same knowledge.
Hence, knowledge representation is used. This will solve complex issues in our world that are hard and time-consuming for humans to tackle.
In this article, I’ll explain knowledge representation in AI, how it works, its types and techniques, and more.
Let’s begin!
What Is Knowledge Representation and Reasoning?
Knowledge representation and reasoning (KR&R) is a part of artificial intelligence that is solely dedicated to representing information about the real world in such a form that a computer can understand and take action accordingly. This leads to solving complex problems, such as computation, having a dialog in natural language, diagnosing a critical medical condition, etc.
Knowledge representation finds its way from psychology about how a human is capable of solving problems and representing knowledge to design formalisms. This will let AI understand how a human makes complex systems simpler while building and designing.

The earliest work was focused on general issue-solvers, which was developed by Herbert A. Simon and Allen Newell in 1959. These systems used data structure for decomposition and planning. The system first starts with a goal and then decomposes the goal into sub-goals. Afterward, the system sets out some construct strategies that can attend to each subgoal.
These efforts then led to a cognitive revolution in human psychology and a phase of AI that focused on knowledge representation. This resulted in expert systems in the 1970s and 1980s, frame languages, production systems, and more. Later, AI changed its primary focus to expert systems that could possibly match human competence, such as medical diagnosis.
Moreover, knowledge representation allows computer systems to understand and utilize the knowledge to solve real-world problems. It also defines a way through which you can represent knowledge and reasoning in AI.
Knowledge representation is not just about storing data in databases; rather, it enables intelligent machines to learn from human knowledge and experience the same so that a machine can behave and act like a human.

Humans have knowledge that is alien to machines, including feelings, intentions, beliefs, common sense, judgments, prejudices, intuition, and more. Some knowledge is also straightforward, like knowing certain facts, general knowledge of events, people, objects, language, academic disciplines, etc.
With KR&R, you can represent the concepts of humans in an understandable format for machines and make the AI-powered systems truly intelligent. Here, knowledge means providing information regarding the ecosystem and storing them, whereas reasoning means taking decisions and actions from the stored information based on the knowledge.
What Knowledge Is to Be Represented in AI Systems?
The knowledge that needs to be presented in artificial intelligence systems can include:
- Object: Objects surround humans constantly. Hence, the information regarding those objects is essential and must be considered a knowledge type. For example, pianos have white and black keys, cars have wheels, buses need drivers, planes need pilots, etc.
- Events: Numerous events are constantly taking place in the real world. And human perception is based on events. AI needs to have events knowledge to take action. Some events are famines, the advancement of societies, wars, disasters, achievements, and more.
- Performance: This knowledge deals with humans’ certain actions in various situations. It represents the behavior side of knowledge which is quite essential for AI to understand.

- What we already know
- What we know is basically things we do not know completely
- What we do not know yet
- Meta knowledge deals with the first one, i.e, what we know and lets AI perceive the same.
- Facts: This knowledge is based on the factual description of our world. For example, the earth is not flat but also not round; our sun has a voracious appetite, and more.
- Knowledge-base: The knowledge base is the main component of human intelligence. This refers to a group of relevant data or information on any field, description, and more. For example, a knowledge base on designing a car model.
How Does Knowledge Representation Work?
Typically, a task to carry out, a problem to solve, and getting a solution, is given informally, like delivering parcels when they arrive or fixing electrical issues in the house.

To solve a real problem, the system designer must:
- Carry out the task to determine what better solution it can provide
- Represent the issue in a language so a computer can reason it
- Use the system to computer a final output, which is the solution for users or a sequence of activities needed to be done in the ecosystem.
- Interpret the final result as a solution to the primary issue
Knowledge is the information that a human already has, but machines need to learn. Since there are a lot of problems, the machine needs knowledge. As a part of the design system, you can define what knowledge is to be represented.
Connection Between Knowledge Representation and AI
Knowledge plays an essential role in intelligence. It is also responsible for the creation of artificial intelligence. When it is needed to express intelligent behavior in the AI agents, it plays a necessary role. An agent is unable to function accurately when it lacks experience or knowledge of certain inputs.

For example, if you want to interact with a person but are unable to understand the language, it is obvious that you can’t respond well and deliver any action. This works the same for agents’ intelligent behavior. AI needs to have enough knowledge to carry out the functionality as a decision-maker discovers the environment and applies the required knowledge.
However, AI can’t exhibit intellectual behavior without the components of knowledge.
Types of Knowledge Represented in AI
Now that we are clear about why we need knowledge representation in AI, let’s find out the types the knowledge represented in an AI system.
- Declarative knowledge: It represents the objects, concepts, and facts that help you describe the whole world around you. Thus, it shares the description of something and expresses declarative sentences.
- Procedural Knowledge: Procedural knowledge is less compared to declarative knowledge. It is also known as imperative knowledge, which is used by mobile robots. It’s for declaring the accomplishment of something. For example, with just a map of a building, mobile robots can make their own plan. Mobile robots can plan to attack or perform navigation.

Moreover, procedural knowledge is directly applied to the task that, includes rules, procedures, agendas, strategies, and more.
- Meta Knowledge: In the field of artificial intelligence, pre-defined knowledge is known as meta-knowledge. For example, the study of tagging, learning, planning, etc., falls under this type of knowledge. This model changes its behavior with time and utilizes other specifications. A system engineer or knowledge engineer utilizes various forms of meta-knowledge, such as accuracy, assessment, purpose, source, life span, reliability, justification, completeness, consistency, applicability, and disambiguation.
- Heuristic Knowledge: This knowledge, which is also known as shallow knowledge, follows the thumb rule principle. Hence, it is highly efficient in the process of reasoning as it can solve issues based on past records or problems that are compiled by experts. However, it gathers experiences of past problems and provides a better knowledge-based approach to specify problems and take action.
- Structural Knowledge: Structural knowledge is the most simple and basic knowledge that is used and applied in solving complex problems. It tries to find an effective solution by finding the relationship between objects and concepts. In addition, it describes the relationship between multiple concepts, like part of, kind of, or grouping of something.
Declarative knowledge can be represented as the describing one, whereas procedural knowledge is the doing one. Additionally, declarative knowledge is defined as explicit, whereas procedural knowledge is tacit or implicit. It is declarative knowledge if you can articulate the knowledge and procedural knowledge if you can’t articulate it.
Techniques of Knowledge Representation in AI

There are four major techniques out there that represent the knowledge in AI:
- Logical representation
- Semantic networks
- Production rules
- Frame representation
Logical Representation
Logical representation is the basic form of knowledge representation to the machines where a defined syntax with basic rules is used. This syntax has no ambiguity in the meaning and deals with prepositions. However, the logical form of knowledge representation acts as the communication rules. This is the reason it can be used to represent facts to the machines.
Logical representation is of two types:
- Propositional Logic: Propositional logic is also known as statement logic or propositional calculus that works in a Boolean, which means a method of True or False.
- First-order Logic: First-order logic is a type of logical knowledge representation that you can also term First Order Predicate Calculus Logic (FOPL). This representation of logical knowledge represents the predicates and objects in quantifiers. It is an advanced model of propositional logic.
This form of knowledge representation looks like most of the programming languages where you use semantics to forward information. It is a highly logical way of solving problems. However, the main drawback of this method is the strict nature of the representation. In general, it is tough to execute and not very efficient sometimes.
Semantic Networks

A graphical representation, in this type of knowledge representation, carries the connected objects which are used with the data network. The semantic networks include arcs/edges (connections) and nodes/blocks (objects) that describe the connection between the objects.
This is an alternative to the First Order Predicate Calculus Logic (FOPL) form of representation. The relationships in the semantic networks are of two types:
It is a more natural form of representation than logical due to its simplicity of understanding. The main downside of this form of representation is that it is computationally expensive and doesn’t include equivalent quantifiers that you can find in logical representation.
Production Rules
Production rules are the most common form of knowledge representation in AI systems. It is the simplest form of representing if-else rule-based systems and hence, can be understood easily. It represents a way of combining FOPL and propositional logic.
In order to technically understand the production rules, you need to first understand the constituents of the representation system. This system includes a set of rules, working memory, rule applier, and a recognized act cycle.
For every input, AI checks the conditions from the production rules, and after finding a better rule, it takes the needed action immediately. The cycle of selecting rules based on the conditions and acting to solve the issue is known as the recognition and act cycle that takes place in every input.
However, this method has some problems, such as inefficient execution due to the active rules and lack of gaining experience due to no storage of past results. Since the rules are expressed in natural language, the cost of the disadvantages can be redeemed. Here, rules can be changed and dropped easily if required.
Frame Representation

To understand the frame representation at a fundamental level, imagine a table consisting of names in columns and values in rows; the needed information is passed in this complete structure. In simple words, frame representation is a collection of values and attributes.
This is an AI-specific data structure that uses fillers (slot values that can be of any data type and shape) and slots. The process is quite similar to the typical Database Management System (DBMS). These fillers and slots form a structure called a frame.
The slots, in this form of knowledge representation, have names or attributes, and the knowledge related to the attributes is stored in fillers. The main advantage of this type of representation is that similar data can be merged into groups to divide the knowledge into structures. Further, it is divided into sub-structures.
Being like a typical data structure, this type can be understood, manipulated, and visualized easily. Typical concepts, including removing, deleting, and adding slots, can be carried out effortlessly.
Requirements for Knowledge Representation in AI system
A good knowledge representation contains some properties:
- Representational accuracy: Knowledge representation needs to represent each kind of required knowledge accurately.
- Inferential efficiency: It is the ability to handle inferential knowledge mechanisms easily in productive directions using appropriate guides.
- Inferential adequacy: Knowledge representation should have the ability to manipulate some representational structures to represent new knowledge based on the existing structures.
- Acquisitional efficiency: The ability to gain new knowledge using automatic methods.
AI Knowledge Cycle

AI systems include some major components to show intelligent behavior that make representing knowledge possible.
- Perception: It helps the AI-based system collect information about the environment using different sensors and makes it familiar with the ecosystem to efficiently interact with the problems.
- Learning: It is used to allow AI systems to run deep learning algorithms that are already written to make AI systems deliver the needed information from the perception component to the learning component for better learning and understanding.
- Knowledge representation and reasoning: Humans use knowledge to make decisions. Hence, this block is responsible for serving humans through the knowledge data of AI systems and using relevant knowledge whenever required.
- Planning and execution: This block is independent. It is used to take data from knowledge and reasoning blocks and execute relevant actions.
Humans can gain knowledge in different ways, and so do AI-based machines. As AI is evolving, representing knowledge to machines in a better way helps you solve complex problems with minimal error. So, knowledge representation is an essential attribute for AI machines to work intelligently and smartly.
You may also look at the difference between Artificial Intelligence, Machine Learning, and Deep Learning .
Knowledge Representation in AI
Humans are great at tasks that require creativity , critical thinking , and empathy . They can learn from experience and adapt to new situations, and they possess emotional intelligence that allows them to understand and connect with other people on a deep level.
On the other hand, Artificial Intelligence or AI is excellent at tasks that require speed, accuracy, and scalability. It can quickly process vast amounts of data and perform complex calculations and analyses far beyond human capabilities.
But how does AI understand knowledge or data for its benefits? This article will give you the perfect answer to this question.
Introduction
Knowledge representation is a crucial element of Artificial Intelligence. It is believed that an intelligent system needs to have an explicit representation of its knowledge to reason and make decisions.
Knowledge representation provides a framework for representing, organizing, and manipulating knowledge that can be used to solve complex problems, make decisions, and learn from data.
For example, when you see a hot tea cup, a signal immediately comes from your brain cautioning you against picking it up. If we were to make AI more sophisticated(or humanist), we would be required to feed them with more and often complex information about our world to perform the complex task, which leads to the concept of Knowledge Representation in Artificial Intelligence.
What is Knowledge Representation in AI?
Knowledge representation is a fundamental concept in artificial intelligence (AI) that involves creating models and structures to represent information and knowledge in a way that intelligent systems can use. The goal of knowledge representation is to enable machines to reason about the world like humans, by capturing and encoding knowledge in a format that can be easily processed and utilized by AI systems.
There are various approaches to knowledge representation in AI, including:
Logical representation: This involves representing knowledge in a symbolic logic or rule-based system , which uses formal languages to express and infer new knowledge.
Semantic networks: This involves representing knowledge through nodes and links, where nodes represent concepts or objects, and links represent their relationships .
Frames: This approach involves representing knowledge in the form of structures called frames , which capture the properties and attributes of objects or concepts and the relationships between them.
Ontologies: This involves representing knowledge in the form of a formal, explicit specification of the concepts, properties, and relationships between them within a particular domain.
Neural networks: This involves representing knowledge in the form of patterns or connections between nodes in a network, which can be used to learn and infer new knowledge from data.
The Different Kinds of Knowledge: What to Represent
- Object: The AI needs to know all the facts about the objects in our world domain. E.g., A keyboard has keys, a guitar has strings, etc.
- Events: The actions which occur in our world are called events.
- Performance: It describes a behavior involving knowledge about how to do things.
- Meta-knowledge: The knowledge about what we know is called meta-knowledge.
- Facts: The things in the real world that are known and proven true.
- Knowledge Base: A knowledge base in artificial intelligence aims to capture human expert knowledge to support decision-making, problem-solving , and more.
Types of Knowledge in AI
In AI, various types of knowledge` are used for different purposes. Here are some of the main types of knowledge in AI:
Declarative Knowledge: This knowledge can be expressed in a declarative form, such as facts, rules, or propositions. It is also called descriptive knowledge and is expressed in declarative sentences. It is often represented using logic-based representations such as knowledge graphs or ontologies. Example: The capital of France is Paris. This statement represents declarative knowledge because it is a fact that can be explicitly stated and written down. It is not based on personal experience or practical skills, but rather on an established piece of information that can be easily communicated to others.
Procedural Knowledge: This knowledge is used to perform specific tasks or actions and is often represented using algorithms or programming languages . It is responsible for knowing how to do something. It includes rules, strategies, procedures, agendas, etc. Example: How to change a flat tire on a car, including the steps of loosening the lug nuts, jacking up the car, removing the tire, and replacing it with a spare. This is a practical skill that involves specific techniques and steps that must be followed to successfully change a tire.
Meta-knowledge: This is knowledge about knowledge and is often used to reason about and improve the performance of AI systems. Example: To remember new information, it is helpful to use strategies such as repetition, visualization, and elaboration. This statement represents metaknowledge because it is knowledge about how to learn and remember new information, rather than knowledge about a specific fact or concept. It acknowledges that some specific techniques and strategies can be used to enhance memory and learning, and encourages the use of these techniques to improve learning outcomes.
Heuristic Knowledge: Heuristics are based on past experiences or domain knowledge and are often used in decision-making processes to guide an AI system toward a solution. Heuristic knowledge is a type of knowledge in AI that refers to rules of thumb or strategies that are used to solve problems quickly and efficiently, but only sometimes optimally. Heuristics are often used when there is too much complexity or uncertainty in a problem to use an exact algorithm or solution. Example: When packing for a trip, it is helpful to make a list of essential items, pack versatile clothing items that can be mixed and matched, and leave room in the suitcase for any souvenirs or purchases. This statement represents heuristic knowledge because it is a practical set of rules of thumb that can be used to guide decision-making in a specific situation (packing for a trip).
Structural Knowledge: This is knowledge about the structure of a problem or system and is often used to help AI systems decompose complex problems into simpler sub-problems that can be solved more easily. It is the basic knowledge of problem-solving. It also describes relationships between concepts such as kind of, part of, and grouping of something. Example: In the field of biology, living organisms can be classified into different taxonomic groups based on shared characteristics. These taxonomic groups include domains, kingdoms, phyla, classes, orders, families, genera, and species. This statement represents structural knowledge because it describes the hierarchical structure of the taxonomic classification system used in biology. It acknowledges that there are specific levels of organization within this system and that each level has its unique characteristics and relationships to other levels.
The Relation Between Knowledge and Intelligence
Knowledge and intelligence are related but distinct concepts. Knowledge refers to the information, skills, and understanding that an individual has acquired through learning and experience. In contrast, intelligence refers to the ability to think abstractly, reason, learn quickly, solve problems, and adapt to new situations.
In the context of AI, knowledge, and intelligence are also distinct but interrelated concepts. AI systems can be designed to acquire knowledge through machine learning or expert systems. Still, the ability to reason, learn, and adapt to new situations requires a more general intelligence that is beyond most AI systems' capabilities.
An agent can only act accurately on some input when it has some knowledge or experience about that input.
Nonetheless, using knowledge-based systems and other AI techniques can help enhance the intelligence of machines and enable them to perform a wide range of tasks.
AI Knowledge Cycle
The AI knowledge cycle is a process that involves the acquisition, representation, and utilization of knowledge by AI systems. It consists of several stages, including:
Data collection: This stage involves gathering relevant data from various sources such as sensors, databases, or the internet.
Data preprocessing: The collected data is then cleaned, filtered, and transformed into a suitable format for analysis.
Knowledge representation: This stage involves encoding the data into a format that an AI system can use. This can include symbolic representations, such as knowledge graphs or ontologies, or numerical representations, such as feature vectors.
Knowledge inference: Once the data has been represented, an AI system can use this knowledge to make predictions or decisions. This involves applying machine learning algorithms or other inference techniques to the data.
Knowledge evaluation: This stage involves evaluating the accuracy and effectiveness of the knowledge that has been inferred. This can involve testing the AI system on known examples or other evaluation metrics.
Knowledge refinement: Based on the evaluation results, the knowledge representation and inference algorithms can be refined or updated to improve the accuracy and effectiveness of the AI system.
Knowledge utilization: Finally, the knowledge acquired and inferred can be used to perform various tasks, such as natural language processing , image recognition , or decision-making .
The AI knowledge cycle is a continuous process, as new data is constantly being generated, and the AI system can learn and adapt based on this new information. By following this cycle, AI systems can continuously improve their performance and perform a wide range of tasks more effectively.
Approaches to Knowledge Representation
Simple relational knowledge.
- This type of knowledge uses relational methods to store facts.
- It is one of the simplest types of knowledge representation.
- The facts are systematically set out in terms of rows and columns.
- This type of knowledge representation is used in database systems where the relationship between different entities is represented.
- There is a low opportunity for inference.
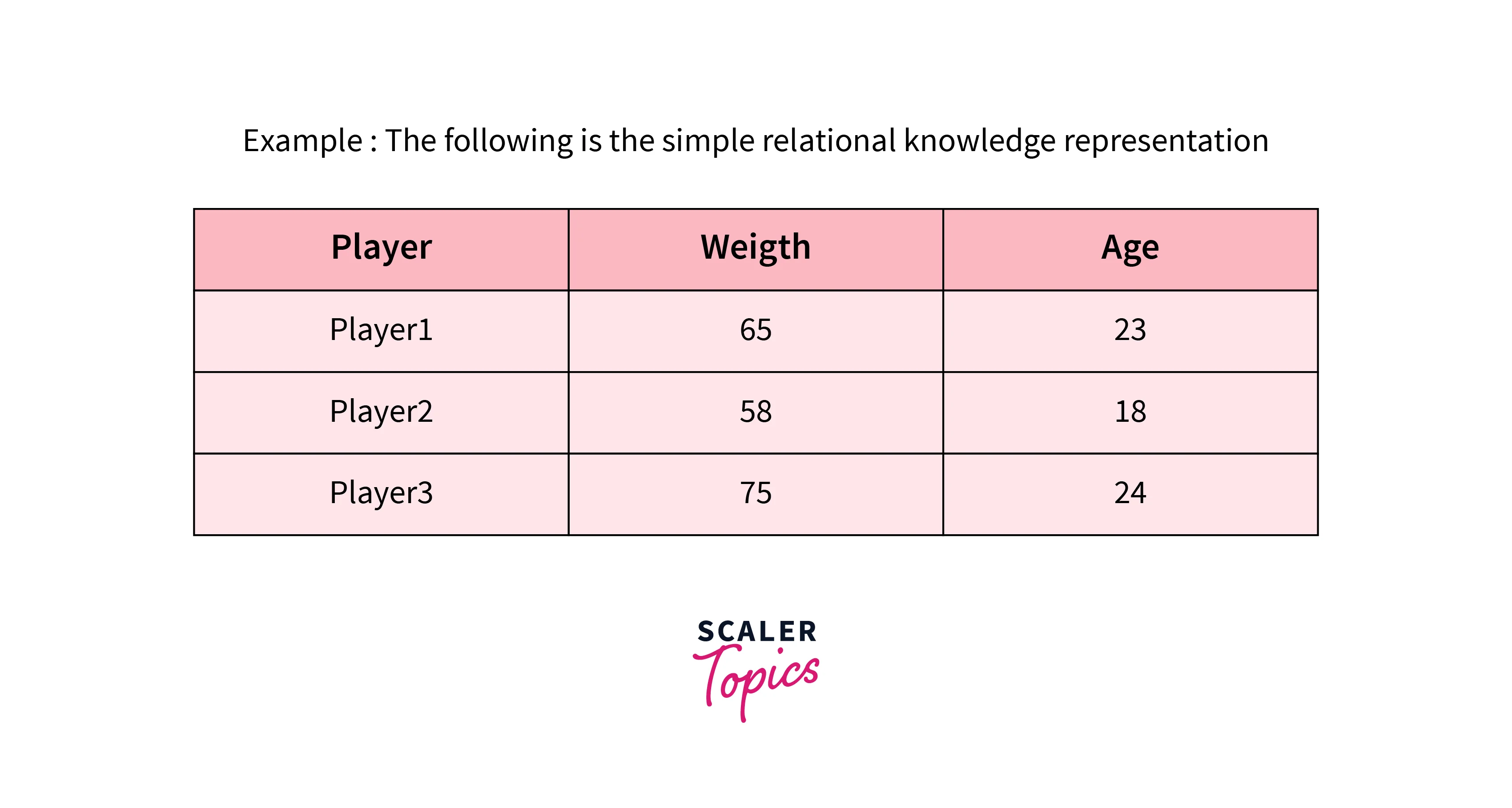
Inheritable Knowledge
- Inheritable knowledge in AI refers to knowledge acquired by an AI system through learning and can be transferred or inherited by other AI systems.
- This knowledge can include models, rules, or other forms of knowledge that an AI system learns through training or experience.
- In this approach, all data must be stored in a hierarchy of classes.
- Boxed nodes are used to represent objects and their values.
- We use Arrows that point from objects to their values.
- Rather than starting from scratch , an AI system can inherit knowledge from other systems, allowing it to learn faster and avoid repeating mistakes that have already been made. Inheritable knowledge also allows for knowledge transfer across domains, allowing an AI system to apply knowledge learned in one domain to another.
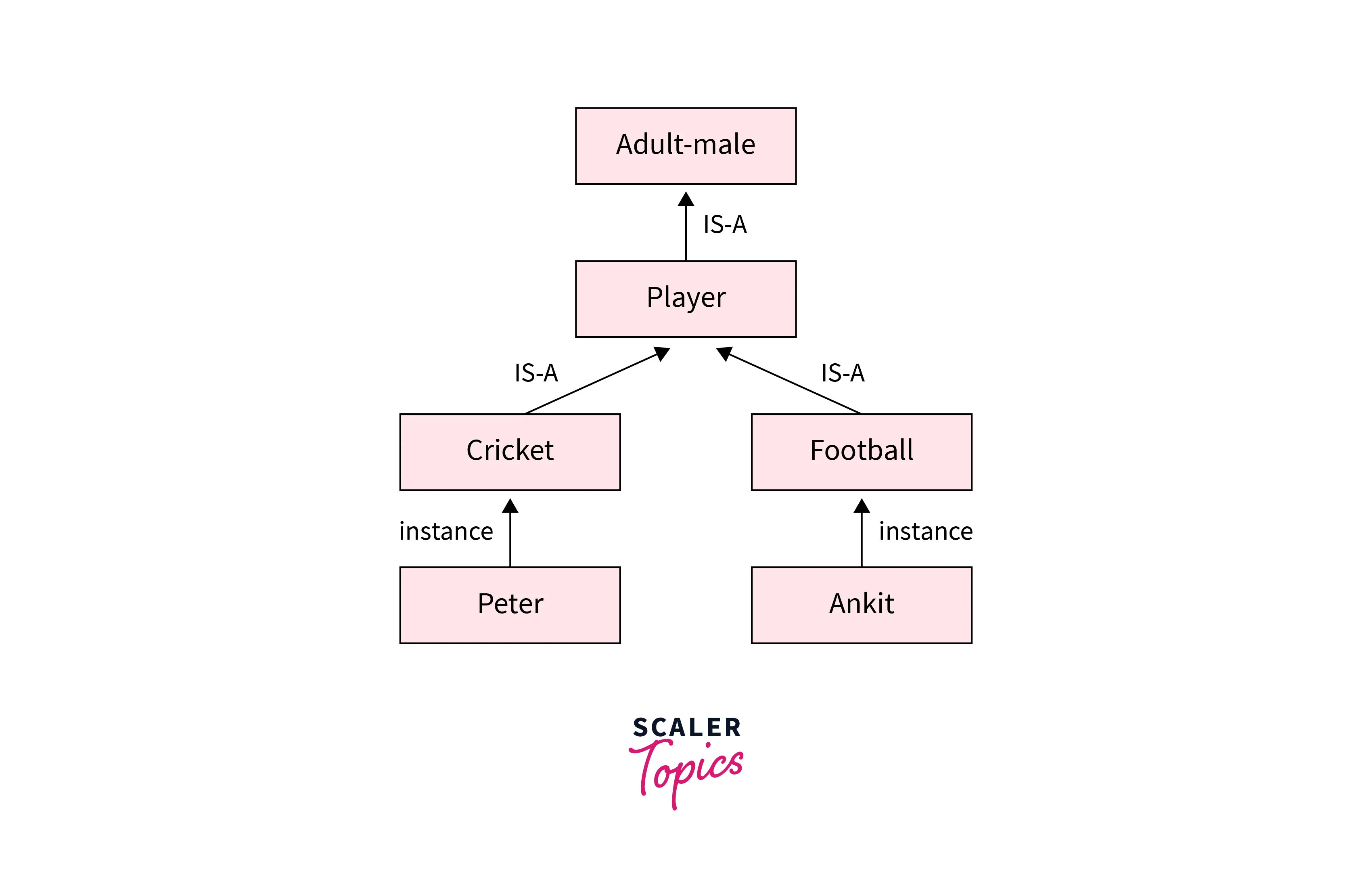
Inferential Knowledge
- Inferential knowledge refers to the ability to draw logical conclusions or make predictions based on available data or information
- In artificial intelligence , inferential knowledge is often used in machine learning algorithms, where models are trained on large amounts of data and then used to make predictions or decisions about new data.
- For example, in image recognition, a machine learning model can be trained on a large dataset of labeled images and then used to predict the contents of new images that it has never seen before. The model can draw inferences based on the patterns it has learned from the training data.
- It represents knowledge in the form of formal logic.
Example: Statement 1: Alex is a footballer. Statement 2: All footballers are athletes. Then it can be represented as; Footballer(Alex) ∀x = Footballer (x) ———-> Athelete (x)s
Procedural Knowledge:
- In artificial intelligence , procedural knowledge refers to the knowledge or instructions required to perform a specific task or solve a problem.
- This knowledge is often represented in algorithms or rules dictating how a machine processes data or performs tasks.
- For example, in natural language processing, procedural knowledge might involve the steps required to analyze and understand the meaning of a sentence. This could include tasks such as identifying the parts of speech in the sentence, identifying relationships between different words, and determining the overall structure and meaning of the sentence.
- One of the most important rules used is the If-then rule.
- This knowledge allows us to use various coding languages such as LISP and Prolog .
- Procedural knowledge is an important aspect of artificial intelligence, as it allows machines to perform complex tasks and make decisions based on specific instructions.
Requirements For Knowledge Representation System
Representational accuracy.
Representational accuracy refers to the degree to which a knowledge representation system accurately captures and reflects the real-world concepts, relationships, and constraints it intends to represent. In artificial intelligence, representational accuracy is important because it directly affects the ability of a system to reason and make decisions based on the knowledge stored within it.
A knowledge representation system that accurately reflects the real-world concepts and relationships that it is intended to represent is more likely to produce accurate results and make correct predictions. Conversely, a system that inaccurately represents these concepts and relationships is more likely to produce errors and incorrect predictions.
Inferential Adequacy:
Inferential adequacy refers to the ability of a knowledge representation system or artificial intelligence model to make accurate inferences and predictions based on the knowledge that is represented within it. In other words, an inferentially adequate system can reason and draw logical conclusions based on its available information.
Achieving inferential adequacy requires a knowledge representation system or AI model to be designed with a well-defined reasoning mechanism that can use the knowledge stored within it. In addition, this mechanism should be able to apply rules and principles to the available data to make accurate inferences and predictions .
Inferential Efficiency
Inferential efficiency in artificial intelligence refers to the ability of a knowledge representation system or AI model to perform reasoning and inference operations in a timely and efficient manner. In other words, an inferentially efficient system should be able to make accurate predictions and draw logical conclusions quickly and with minimal computational resources .
Achieving inferential efficiency requires several factors, including the complexity of the reasoning mechanism, the amount and structure of the data that needs to be processed, and the computational resources available to the system. As a result, AI researchers and developers often employ various techniques and strategies to improve inferential efficiency, including optimizing the algorithms used for inference, improving the data processing pipeline, and utilizing specialized hardware or software architectures designed for efficient inferencing.
Acquisitional efficiency
Acquisitional efficiency in artificial intelligence refers to the ability of a knowledge representation system or AI model to effectively and efficiently acquire new knowledge or information. In other words, an acquisitionally efficient system should be able to rapidly and accurately learn from new data or experience.
Achieving acquisitional efficiency requires several factors, including the ability to recognize patterns and relationships in the data, the ability to generalize from examples to new situations, and the ability to adapt to changing circumstances or contexts. AI researchers and developers often employ various techniques and strategies to improve acquisitional efficiency, including active learning, transfer learning, and reinforcement learning.
The key takeaways from this article are:-
- Knowledge representation is a fundamental concept in artificial intelligence (AI) that involves creating models and structures to represent information and knowledge in a way that intelligent systems can use.
- Objects, events, performance, meta-knowledge , facts, and knowledge-base are the different kinds of knowledge.
- The AI knowledge cycle is a process that involves the acquisition, representation, and utilization of knowledge by AI systems.
- Relational, inferential, procedural , and inheritable are four approaches to knowledge representation.
Q. How is AI used in cybersecurity?
A. AI (Artificial Intelligence) is increasingly used in cybersecurity to improve the efficiency and effectiveness of various security measures. Here are some ways in which AI is used in cybersecurity:
Threat detection: AI algorithms can be trained to identify patterns and anomalies in network traffic, which can help detect potential threats and attacks. These algorithms can monitor network activity, log files, and other data sources to identify unusual behavior and respond to potential threats.
Malware detection: AI can identify and classify different types of malware. AI-powered antivirus software can use machine learning algorithms to learn from past malware behavior and detect new variants.
Fraud detection: AI can detect fraudulent activity in financial transactions, such as credit card fraud or money laundering. AI algorithms can analyze large amounts of data and identify patterns that may indicate fraudulent activity.
Vulnerability assessment: AI can scan systems and networks for vulnerabilities that attackers could exploit. AI-powered vulnerability scanners can analyze system configurations and identify potential security weaknesses.
Incident response: AI can automate incident response processes, such as isolating infected systems, blocking malicious traffic, and restoring compromised data.
User authentication: AI can be used to analyze user behavior patterns to detect anomalies and prevent unauthorized access. For example, AI-powered systems can learn how users typically access a system and identify if a user's unusual behavior indicates a potential security threat.
Q. Will AI take over cybersecurity?
A. No, AI will not take over cybersecurity entirely. While AI can potentially improve the efficiency and effectiveness of various security measures, it is not a substitute for human expertise in cybersecurity.
AI can help automate routine tasks such as malware detection, but it still requires human oversight and intervention to ensure the accuracy of the results. Moreover, AI is not infallible and can make mistakes or be vulnerable to attacks. Therefore, human cybersecurity experts are still needed to evaluate and interpret the results generated by AI-powered systems and to make decisions based on their expertise and experience.
Q. What is AI in cybersecurity?
A. In cybersecurity, AI (Artificial Intelligence) refers to using machine learning algorithms and other AI techniques to enhance various security measures. AI-powered cybersecurity systems can analyze large amounts of data, detect patterns, and make decisions based on that analysis without requiring human intervention .
Knowledge Representation in AI: The Foundation of Intelligent Systems

Knowledge representation plays a crucial role in the field of artificial intelligence (AI), serving as the bedrock for the development of intelligent systems. By allowing AI systems to organize and process information in a way that imitates human cognition, knowledge representation bridges the gap between raw data and meaningful insights. This article explores the various techniques and approaches used in AI knowledge representation , highlighting its importance and applications in different domains.
Key Takeaways:
- AI knowledge representation is essential for organizing and processing information in a way that mimics human cognition.
- Techniques like semantic networks , frames , and ontologies enable AI systems to capture and reason with hierarchical, associative, and formal relationships.
- Knowledge representation is crucial for AI reasoning, decision-making, and providing valuable recommendations in various domains.
- Challenges in knowledge representation include ambiguity and vagueness in natural language and handling complex and scalable data.
- Applications of knowledge representation span expert systems , natural language processing , and robotics , enhancing capabilities in decision-making, language understanding, and physical interaction.
Understanding Knowledge Representation

Knowledge representation is a fundamental process in artificial intelligence (AI) that enables AI systems to comprehend and manipulate information. By structuring and organizing data in a way that mirrors human understanding, knowledge representation facilitates the reasoning and decision-making capabilities of AI systems. Various techniques, such as semantic networks , frames , and ontologies , are employed to capture the hierarchical, associative, and formal relationships between concepts, enhancing the AI system’s ability to generate intelligent responses.
One common technique used in knowledge representation is semantic networks . These networks utilize interconnected nodes and edges to represent concepts and the relationships between them. This approach allows for the capture of hierarchical knowledge structures, enabling AI systems to understand the context and associations between different concepts. Frames , on the other hand, involve organizing knowledge into predefined categories known as frames, which contain attributes, properties, and relationships. This technique allows for the structured representation of knowledge, making it easier for AI systems to process and reason with information.
“Knowledge representation enables AI systems to capture the essence of human cognition, allowing them to mimic our thought processes and generate intelligent responses.” – AI Expert
Ontologies provide a formal structure for knowledge representation and are often used in domains where precise classification and categorization are required. These hierarchical representations allow for efficient retrieval and inference by AI systems, enabling them to navigate complex knowledge domains effectively. By employing these techniques, AI systems can understand and interpret information in a manner similar to human cognition, facilitating problem-solving, decision-making, and generating valuable insights.
Table: Comparison of Knowledge Representation Techniques
Technique | Description | Advantages | Disadvantages |
---|---|---|---|
Semantic Networks | Use interconnected nodes and edges to represent concepts and relationships. | Facilitates efficient retrieval of hierarchical knowledge structures. | May become complex and difficult to manage as networks grow in size. |
Frames | Organize knowledge into predefined categories containing attributes and relationships. | Provides a structured representation of knowledge. | Requires upfront definition of frames and may not handle ambiguous data well. |
Ontologies | Establish a formal structure for representing knowledge using a taxonomy-like hierarchy. | Enables precise classification and categorization of knowledge. | Creating and maintaining ontologies can be time-consuming and resource-intensive. |
The choice of knowledge representation technique depends on the specific requirements and characteristics of the AI application. Each technique has its strengths and limitations, and the selection should be based on the nature of the knowledge and the intended use of the AI system.
Importance of Knowledge Representation in AI
Knowledge representation plays a crucial role in the field of artificial intelligence (AI) by enabling reasoning and decision-making. AI systems need a structured format to process and understand information, allowing them to derive valuable insights and make informed choices. By representing knowledge in a way that mirrors human cognition, AI systems can effectively solve complex problems and provide relevant recommendations in various domains.
AI reasoning and decision-making heavily rely on the structured representation of knowledge. Without a well-organized framework, AI systems would struggle to comprehend and analyze vast amounts of information. Knowledge representation allows AI systems to capture the hierarchical, associative, and formal relationships among concepts, providing a foundation for intelligent reasoning.
Additionally, knowledge representation empowers AI systems to handle uncertainty and ambiguity inherent in human language and real-world data. By encapsulating knowledge in structured formats such as semantic networks, frames, and ontologies, AI systems can better understand context, disambiguate meaning, and make accurate interpretations. This enhanced comprehension enables AI systems to generate intelligent responses and contribute to effective decision-making processes.
The importance of knowledge representation in AI cannot be overstated. It forms the basis for AI systems to reason, learn, and make informed decisions. By representing knowledge in a structured and meaningful way, AI systems can bridge the gap between raw data and valuable insights, leading to advancements in various domains and contributing to the development of more intelligent systems.
Techniques of Knowledge Representation
In the field of artificial intelligence (AI), several techniques are commonly used for knowledge representation. These techniques play a vital role in enabling AI systems to understand and process information effectively. Some of the prominent techniques are semantic networks, frames, and ontologies.
1. Semantic Networks:
Semantic networks are graphical representations that depict the relationships between different concepts. In a semantic network, nodes represent individual concepts, while edges illustrate the connections or associations between these concepts. This technique allows AI systems to capture hierarchical and associative knowledge, enabling them to understand complex relationships and make informed decisions. Semantic networks provide a structured framework for organizing information, making it easier for AI systems to retrieve relevant data and draw meaningful insights.
Frames involve the organization of knowledge into predefined categories known as frames. Each frame consists of attributes, properties, and relationships that define a particular concept or object. By using frames, AI systems can represent knowledge in a structured manner, allowing for efficient reasoning and decision-making. Frames provide a way to capture important characteristics and associations related to specific concepts, enhancing the AI system’s understanding and ability to generate intelligent responses.
3. Ontologies:
Ontologies provide a formal and explicit representation of knowledge. They establish a hierarchy of concepts and their relationships, similar to a taxonomy. Ontologies not only define the concepts but also specify the properties, attributes, and constraints associated with each concept. This technique enables AI systems to reason and infer based on the defined rules and relationships within the ontology. Ontologies offer a systematic approach to knowledge representation, facilitating efficient knowledge acquisition, storage, and retrieval.
Technique | Description | Advantages |
---|---|---|
Semantic Networks | Graphical representations depicting relationships between concepts | Allows for capturing hierarchical and associative knowledge |
Frames | Organizing knowledge into predefined categories with attributes and relationships | Provides a structured framework for efficient reasoning and decision-making |
Ontologies | Formal representation of knowledge with a defined hierarchy of concepts | Enables systematic knowledge acquisition, storage, and retrieval |
These techniques of knowledge representation, namely semantic networks, frames, and ontologies, provide AI systems with the necessary tools to organize, understand, and reason with information effectively. Each technique has its unique advantages and applications, allowing AI systems to tackle complex problems and generate intelligent responses. The choice of technique depends on the specific requirements and characteristics of the AI system and the domain in which it operates.
Challenges in Knowledge Representation
Knowledge representation in AI faces various challenges that impact its effectiveness and efficiency. Two major challenges in knowledge representation are Ambiguity and Vagueness , and Scalability and Complexity .
Ambiguity and Vagueness
Ambiguity refers to the presence of multiple interpretations or meanings for a given piece of information. In natural language, words and phrases may have different meanings depending on the context, making it challenging to represent knowledge accurately. Vagueness, on the other hand, refers to the lack of clarity or precision in defining concepts or boundaries.
The representation of ambiguous and vague information requires AI systems to handle uncertainty and make probabilistic inferences. Techniques such as fuzzy logic and probabilistic reasoning can be used to manage ambiguity and vagueness , allowing AI systems to make informed decisions even when dealing with imprecise or uncertain knowledge.
Scalability and Complexity
As knowledge bases grow in size and complexity, maintaining a coherent and efficient representation becomes a significant challenge. The scalability challenge arises due to the need to handle vast amounts of data and ensure quick and accurate retrieval. AI systems must efficiently organize and index knowledge for efficient search and retrieval, which becomes increasingly difficult as the volume of data increases.
Furthermore, the complexity of knowledge representation stems from the need to capture diverse and intricate relationships between concepts and entities. Representing highly interconnected knowledge requires sophisticated techniques such as ontologies and semantic networks to maintain the integrity and richness of the information.
Challenges | Description |
---|---|
Ambiguity and Vagueness | Presence of multiple interpretations and lack of clarity in defining concepts and boundaries. |
Managing large and complex knowledge bases while ensuring efficient retrieval and representation of interconnected relationships. |
By addressing these challenges, AI researchers and developers can enhance the quality and effectiveness of knowledge representation, thereby improving the overall performance of AI systems in reasoning, decision-making, and problem-solving tasks.

Applications of Knowledge Representation

Knowledge representation plays a vital role in various domains, including expert systems , natural language processing , and robotics . These applications leverage the power of knowledge representation techniques to enhance AI capabilities and enable intelligent interactions with humans and the physical world.
Expert Systems
In the field of expert systems , knowledge representation is instrumental in replicating the decision-making abilities of human experts. By utilizing techniques such as semantic networks, frames, and ontologies, AI systems can capture and organize domain-specific knowledge. This structured representation allows expert systems to provide valuable advice, recommendations, and solutions in various industries such as healthcare, finance, and engineering.
Natural Language Processing
Natural language processing (NLP) involves the understanding and generation of human language by AI systems. Knowledge representation plays a crucial role in NLP, enabling machines to comprehend the context of a conversation, identify relationships between words and phrases, and generate coherent responses. By representing knowledge in a structured format, AI systems can analyze and interpret language more effectively, leading to improved communication and interaction with humans.
Knowledge representation is essential in the field of robotics as it enables machines to navigate and interact with the physical world. By representing knowledge about the environment, objects, and actions, AI systems can understand their surroundings and make informed decisions. This allows robots to perform tasks efficiently, ensure safe movements, and adapt to dynamic situations. Knowledge representation empowers robots to learn from previous experiences and apply that knowledge in real-time, enhancing their overall functionality and autonomy.
As AI continues to advance, knowledge representation will play an even more significant role in shaping intelligent systems. Its applications in expert systems, natural language processing, and robotics demonstrate the wide-reaching impact of structured knowledge on enhancing AI capabilities. By refining knowledge representation techniques , AI researchers and practitioners are working towards developing more intelligent, adaptable, and autonomous systems that can revolutionize various industries and improve the lives of individuals worldwide.
The Future of Knowledge Representation
As artificial intelligence (AI) continues to advance and transform various industries, the field of knowledge representation is also evolving to keep pace with the growing complexity of data. Advancements in knowledge representation are crucial for AI systems to effectively handle dynamic and unstructured data, enabling them to make more intelligent decisions and generate meaningful insights.
One of the key areas of focus for future advancements in knowledge representation is the handling of dynamic data. Traditional knowledge representation techniques are often based on static knowledge bases, which can limit the ability of AI systems to adapt and learn from real-time data. By developing techniques that can handle dynamic data, AI systems will be able to continuously update and refine their knowledge, leading to more accurate and up-to-date decision-making.
Another important aspect of future knowledge representation is the handling of unstructured data. Unstructured data, such as text, images, and videos, poses a significant challenge for AI systems, as it lacks a predefined format and structure. Advancements in natural language processing and computer vision are enabling AI systems to better understand and interpret unstructured data, allowing for more effective knowledge representation and analysis.
In summary, the future of knowledge representation in AI holds immense potential for advancements in handling dynamic and unstructured data. By developing techniques that can adapt to real-time data and effectively interpret unstructured information, AI systems will be able to achieve greater levels of understanding and cognition. These advancements will pave the way for more intelligent AI systems that can make informed decisions, provide valuable insights, and revolutionize various industries.
Knowledge representation forms the very essence of AI intelligence . With a structured framework to organize and process information, AI systems gain the ability to think, learn, and reason – mirroring human cognition. The techniques and approaches employed in knowledge representation enable AI systems to decipher intricate data, solve complex problems, and make informed decisions.
As technology continues to advance, optimizing knowledge representation techniques will unlock new frontiers for AI systems to achieve unprecedented levels of understanding and cognition. These advancements empower AI to handle dynamic and unstructured data, paving the way for even smarter systems.
The future of knowledge representation holds immense potential, propelling AI intelligence to greater heights. By continuously refining these techniques, AI systems will transcend their current capabilities, bridging the gap between raw data and meaningful insights. This progress will steer AI towards enhanced cognitive abilities and pave the path for a future where intelligent systems become an indispensable part of our lives.
What is knowledge representation in AI?
Knowledge representation is the process of structuring and organizing information in a format that AI systems can comprehend and manipulate.
Why is knowledge representation important in AI?
Knowledge representation is crucial in AI because it enables reasoning, decision-making, and the generation of meaningful insights from data.
What are the techniques used in knowledge representation?
The techniques used in knowledge representation include semantic networks, frames, and ontologies.
What challenges does knowledge representation face?
Knowledge representation faces challenges related to ambiguity and vagueness in natural language, as well as scalability and complexity as knowledge bases grow in size.
Where is knowledge representation applied?
Knowledge representation finds applications in expert systems, natural language processing, and robotics, among other domains.
How is knowledge representation advancing in the future?
Efforts are being made to develop more sophisticated approaches to handle dynamic and unstructured data, allowing for even smarter AI systems.

The Quest for Transparency in NLP Systems: Understanding the Black Box

Ensuring Fairness in Language Models: Overcoming Bias and Inequality

Protecting User Privacy: Essential Strategies in NLP Applications

Navigating the Ethical Landscape of AI and NLP: Challenges and Solutions

Unveiling Bias in NLP Algorithms: A Path Towards Fairer AI

The Future is Now: Real-time NLP Analysis in Big Data Environments
- Free Onboarding
- Privacy Policy
- Terms and Conditions
- Affiliate Program 💵
- Why Libraria AI?
- Documentation
- API Playground
- Ecommerce AI
- Preprocessing Content with AI
- Libraria AI Internal Chat
- Chatbase vs Libraria AI
- AI Preprocessing
- Images in your AI Chatbot
- Chat History
What Is a Knowledge Representation?
- Randall Davis
- Howard Shrobe
- Peter Szolovits
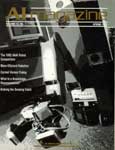
How to Cite
- Endnote/Zotero/Mendeley (RIS)
- The author(s) warrants that they are the sole author and owner of the copyright in the above article/paper, except for those portions shown to be in quotations; that the article/paper is original throughout; and that the undersigned right to make the grants set forth above is complete and unencumbered.
- The author(s) agree that if anyone brings any claim or action alleging facts that, if true, constitute a breach of any of the foregoing warranties, the author(s) will hold harmless and indemnify AAAI, their grantees, their licensees, and their distributors against any liability, whether under judgment, decree, or compromise, and any legal fees and expenses arising out of that claim or actions, and the undersigned will cooperate fully in any defense AAAI may make to such claim or action. Moreover, the undersigned agrees to cooperate in any claim or other action seeking to protect or enforce any right the undersigned has granted to AAAI in the article/paper. If any such claim or action fails because of facts that constitute a breach of any of the foregoing warranties, the undersigned agrees to reimburse whomever brings such claim or action for expenses and attorneys’ fees incurred therein.
- Author(s) retain all proprietary rights other than copyright (such as patent rights).
- Author(s) may make personal reuse of all or portions of the above article/paper in other works of their own authorship.
- Author(s) may reproduce, or have reproduced, their article/paper for the author’s personal use, or for company use provided that original work is property cited, and that the copies are not used in a way that implies AAAI endorsement of a product or service of an employer, and that the copies per se are not offered for sale. The foregoing right shall not permit the posting of the article/paper in electronic or digital form on any computer network, except by the author or the author’s employer, and then only on the author’s or the employer’s own web page or ftp site. Such web page or ftp site, in addition to the aforementioned requirements of this Paragraph, must provide an electronic reference or link back to the AAAI electronic server, and shall not post other AAAI copyrighted materials not of the author’s or the employer’s creation (including tables of contents with links to other papers) without AAAI’s written permission.
- Author(s) may make limited distribution of all or portions of their article/paper prior to publication.
- In the case of work performed under U.S. Government contract, AAAI grants the U.S. Government royalty-free permission to reproduce all or portions of the above article/paper, and to authorize others to do so, for U.S. Government purposes.
- In the event the above article/paper is not accepted and published by AAAI, or is withdrawn by the author(s) before acceptance by AAAI, this agreement becomes null and void.
Information
- For Readers
- For Authors
Developed By
Part of the PKP Publishing Services Network
Copyright © 2021, Association for the Advancement of Artificial Intelligence. All rights reserved.

Data Science Introduction
- What Is Data Science? A Beginner's Guide To Data Science
- Data Science Tutorial – Learn Data Science from Scratch!
- What are the Best Books for Data Science?
- Top 15 Hot Artificial Intelligence Technologies
- Top 8 Data Science Tools Everyone Should Know
- Top 10 Data Analytics Tools You Need To Know In 2024
- 5 Data Science Projects – Data Science Projects For Practice
- Top 10 Data Science Applications with Real Life Examples in 2024
- Who is a Data Scientist?
- SQL For Data Science: One stop Solution for Beginners
Statistical Inference
- All You Need To Know About Statistics And Probability
- A Complete Guide To Math And Statistics For Data Science
- Introduction To Markov Chains With Examples – Markov Chains With Python
- What is Fuzzy Logic in AI and What are its Applications?
- How To Implement Bayesian Networks In Python? – Bayesian Networks Explained With Examples
- All You Need To Know About Principal Component Analysis (PCA)
- Python for Data Science – How to Implement Python Libraries
Machine Learning
- What is Machine Learning? Machine Learning For Beginners
- Which is the Best Book for Machine Learning?
- Mathematics for Machine Learning: All You Need to Know
- Top 10 Machine Learning Frameworks You Need to Know
- Predicting the Outbreak of COVID-19 Pandemic using Machine Learning
- Introduction To Machine Learning: All You Need To Know About Machine Learning
- Machine Learning Tutorial for Beginners
- Top 10 Applications of Machine Learning in Daily Life
- Machine Learning Algorithms
- How To Implement Find-S Algorithm In Machine Learning?
What is Cross-Validation in Machine Learning and how to implement it?
- All You Need To Know About The Breadth First Search Algorithm
Supervised Learning
- What is Supervised Learning and its different types?
- Linear Regression Algorithm from Scratch
How To Implement Linear Regression for Machine Learning?
- Introduction to Classification Algorithms
- How To Implement Classification In Machine Learning?
- Naive Bayes Classifier: Learning Naive Bayes with Python
- A Comprehensive Guide To Naive Bayes In R
- A Complete Guide On Decision Tree Algorithm
- Decision Tree: How To Create A Perfect Decision Tree?
- What is Overfitting In Machine Learning And How To Avoid It?
- How To Use Regularization in Machine Learning?
Unsupervised Learning
- What is Unsupervised Learning and How does it Work?
- K-means Clustering Algorithm: Know How It Works
- KNN Algorithm: A Practical Implementation Of KNN Algorithm In R
- Implementing K-means Clustering on the Crime Dataset
- K-Nearest Neighbors Algorithm Using Python
- Apriori Algorithm : Know How to Find Frequent Itemsets
- What Are GANs? How and why you should use them!
Q Learning: All you need to know about Reinforcement Learning
Miscellaneous.
- Data Science vs Machine Learning - What's The Difference?
AI vs Machine Learning vs Deep Learning
- Data Analyst vs Data Engineer vs Data Scientist: Salary, Skills, Responsibilities
- Data Science vs Big Data vs Data Analytics
Career Opportunities
- Data Science Career Opportunities: Your Guide To Unlocking Top Data Scientist Jobs
- Data Scientist Skills – What Does It Take To Become A Data Scientist?
- 10 Skills To Master For Becoming A Data Scientist
- Data Scientist Resume Sample – How To Build An Impressive Data Scientist Resume
- Data Scientist Salary – How Much Does A Data Scientist Earn?
- Machine Learning Engineer vs Data Scientist : Career Comparision
- How To Become A Machine Learning Engineer? – Learning Path
Interview Questions
- Top Machine Learning Interview Questions You Must Prepare In 2024
- Top Data Science Interview Questions For Budding Data Scientists In 2024
- 120+ Data Science Interview Questions And Answers for 2024
Artificial Intelligence
What is knowledge representation in ai techniques you need to know.
Human beings are good at understanding, reasoning and interpreting knowledge. And using this knowledge, they are able to perform various actions in the real world. But how do machines perform the same? In this article, we will learn about Knowledge Representation in AI and how it helps the machines perform reasoning and interpretation using Artificial Intelligence in the following sequence:
What is Knowledge Representation?
Different types of knowledge.
- Cycle of Knowledge Representation
- What is the relation between Knowledge & Intelligence?
- Techniques of Knowledge Representation
Representation Requirements
- Approaches to Knowledge Representation with Example
Knowledge Representation in AI describes the representation of knowledge. Basically, it is a study of how the beliefs, intentions , and judgments of an intelligent agent can be expressed suitably for automated reasoning. One of the primary purposes of Knowledge Representation includes modeling intelligent behavior for an agent.
Knowledge Representation and Reasoning ( KR, KRR ) represents information from the real world for a computer to understand and then utilize this knowledge to solve complex real-life problems like communicating with human beings in natural language. Knowledge representation in AI is not just about storing data in a database, it allows a machine to learn from that knowledge and behave intelligently like a human being.
The different kinds of knowledge that need to be represented in AI include:
- Performance
- Meta-Knowledge
- Knowledge-base
Now that you know about Knowledge representation in AI, let’s move on and know about the different types of Knowledge.
There are 5 types of Knowledge such as:
Declarative Knowledge – It includes concepts, facts, and objects and expressed in a declarative sentence.
Structural Knowledge – It is a basic problem-solving knowledge that describes the relationship between concepts and objects.
Procedural Knowledge – This is responsible for knowing how to do something and includes rules, strategies, procedures, etc.
Meta Knowledge – Meta Knowledge defines knowledge about other types of Knowledge.
Heuristic Knowledge – This represents some expert knowledge in the field or subject.
These are the important types of Knowledge Representation in AI. Now, let’s have a look at the cycle of knowledge representation and how it works.
Cycle of Knowledge Representation in AI
Artificial Intelligent Systems usually consist of various components to display their intelligent behavior. Some of these components include:
- Knowledge Representation & Reasoning
Here is an example to show the different components of the system and how it works:
The above diagram shows the interaction of an AI system with the real world and the components involved in showing intelligence.
- The Perception component retrieves data or information from the environment. with the help of this component, you can retrieve data from the environment, find out the source of noises and check if the AI was damaged by anything. Also, it defines how to respond when any sense has been detected.
- Then, there is the Learning Component that learns from the captured data by the perception component. The goal is to build computers that can be taught instead of programming them. Learning focuses on the process of self-improvement. In order to learn new things, the system requires knowledge acquisition, inference, acquisition of heuristics, faster searches, etc.
- The main component in the cycle is Knowledge Representation and Reasoning which shows the human-like intelligence in the machines. Knowledge representation is all about understanding intelligence. Instead of trying to understand or build brains from the bottom up, its goal is to understand and build intelligent behavior from the top-down and focus on what an agent needs to know in order to behave intelligently. Also, it defines how automated reasoning procedures can make this knowledge available as needed.
- The Planning and Execution components depend on the analysis of knowledge representation and reasoning. Here, planning includes giving an initial state, finding their preconditions and effects, and a sequence of actions to achieve a state in which a particular goal holds. Now once the planning is completed, the final stage is the execution of the entire process.
So, these are the different components of the cycle of Knowledge Representation in AI. Now, let’s understand the relationship between knowledge and intelligence.
Top 10 Trending Technologies to Learn in 2024 | Edureka
What is the relation between knowledge & intelligence.
In the real world, knowledge plays a vital role in intelligence as well as creating artificial intelligence . It demonstrates the intelligent behavior in AI agents or systems . It is possible for an agent or system to act accurately on some input only when it has the knowledge or experience about the input.
Let’s take an example to understand the relationship:
In this example, there is one decision-maker whose actions are justified by sensing the environment and using knowledge. But, if we remove the knowledge part here, it will not be able to display any intelligent behavior.
Now that you know the relationship between knowledge and intelligence, let’s move on to the techniques of Knowledge Representation in AI.
Techniques of Knowledge Representation in AI
There are four techniques of representing knowledge such as:
Now, let’s discuss these techniques in detail.
Logical Representation
Logical representation is a language with some definite rules which deal with propositions and has no ambiguity in representation. It represents a conclusion based on various conditions and lays down some important communication rules . Also, it consists of precisely defined syntax and semantics which supports the sound inference. Each sentence can be translated into logics using syntax and semantics.
Advantages:
- Logical representation helps to perform logical reasoning.
- This representation is the basis for the programming languages.
Disadvantages:
- Logical representations have some restrictions and are challenging to work with.
- This technique may not be very natural, and inference may not be very efficient.
Semantic Network Representation
Semantic networks work as an alternative of predicate logic for knowledge representation. In Semantic networks, you can represent your knowledge in the form of graphical networks. This network consists of nodes representing objects and arcs which describe the relationship between those objects. Also, it categorizes the object in different forms and links those objects.
This representation consist of two types of relations:
- IS-A relation (Inheritance)
- Kind-of-relation
- Semantic networks are a natural representation of knowledge.
- Also, it conveys meaning in a transparent manner.
- These networks are simple and easy to understand.
- Semantic networks take more computational time at runtime.
- Also, these are inadequate as they do not have any equivalent quantifiers.
- These networks are not intelligent and depend on the creator of the system.
Frame Representation
A frame is a record like structure that consists of a collection of attributes and values to describe an entity in the world. These are the AI data structure that divides knowledge into substructures by representing stereotypes situations. Basically, it consists of a collection of slots and slot values of any type and size. Slots have names and values which are called facets.
- It makes the programming easier by grouping the related data.
- Frame representation is easy to understand and visualize.
- It is very easy to add slots for new attributes and relations.
- Also, it is easy to include default data and search for missing values.
- In frame system inference, the mechanism cannot be easily processed.
- The inference mechanism cannot be smoothly proceeded by frame representation.
- It has a very generalized approach.
Production Rules
In production rules, agent checks for the condition and if the condition exists then production rule fires and corresponding action is carried out. The condition part of the rule determines which rule may be applied to a problem. Whereas, the action part carries out the associated problem-solving steps. This complete process is called a recognize-act cycle.
The production rules system consists of three main parts:
- The set of production rules
- Working Memory
- The recognize-act-cycle
The production rules are expressed in natural language.
The production rules are highly modular and can be easily removed or modified.
It does not exhibit any learning capabilities and does not store the result of the problem for future uses.
During the execution of the program, many rules may be active. Thus, rule-based production systems are inefficient.
So, these were the important techniques for Knowledge Representation in AI. Now, let’s have a look at the requirements for these representations.
A good knowledge representation system must have properties such as:
Representational Accuracy: It should represent all kinds of required knowledge.
Inferential Adequacy : It should be able to manipulate the representational structures to produce new knowledge corresponding to the existing structure.
Inferential Efficiency : The ability to direct the inferential knowledge mechanism into the most productive directions by storing appropriate guides.
Acquisitional efficiency : The ability to acquire new knowledge easily using automatic methods.
Now, let’s have a look at some of the approaches to Knowledge Representation in AI along with different examples.
Approaches to Knowledge Representation in AI
There are different approaches to knowledge representation such as:
1. Simple Relational Knowledge
It is the simplest way of storing facts which uses the relational method. Here, all the facts about a set of the object are set out systematically in columns. Also, this approach of knowledge representation is famous in database systems where the relationship between different entities is represented. Thus, there is little opportunity for inference.
John | 25 | 100071 |
Amanda | 23 | 100056 |
Sam | 27 | 100042 |
This is an example of representing simple relational knowledge.
2. Inheritable Knowledge
In the inheritable knowledge approach, all data must be stored into a hierarchy of classes and should be arranged in a generalized form or a hierarchal manner. Also, this approach contains inheritable knowledge which shows a relation between instance and class, and it is called instance relation. In this approach, objects and values are represented in Boxed nodes.
3. Inferential Knowledge
The inferential knowledge approach represents knowledge in the form of formal logic . Thus, it can be used to derive more facts. Also, it guarantees correctness.
Statement 1 : John is a cricketer.
Statement 2 : All cricketers are athletes.
Then it can be represented as;
Cricketer(John) ∀x = Cricketer (x) ———-> Athelete (x)s
These were some of the approaches to knowledge representation in AI along with examples. With this, we have come to the end of our article. I hope you understood what is Knowledge Representation in AI and its different types.
Learn more about this trending OpenAI chatbot concept and implementation join the ChatGPT training .
Also, check out the AI Course is curated by industry professionals as per the industry requirements & demands. Thus, You will master the concepts such as SoftMax function, Autoencoder Neural Networks, Restricted Boltzmann Machine (RBM) and work with libraries like Keras & TFLearn. The course has been specially curated by industry experts with real-time case studies.
Got a question for us? Please mention it in the comments section of “Knowledge Representation in AI” and we will get back to you.
Course Name | Date | Details |
---|---|---|
Class Starts on 31st August,2024 31st August SAT&SUN (Weekend Batch) |
Recommended videos for you
Introduction to mahout, recommended blogs for you, tensorflow tutorial – deep learning using tensorflow, what is em algorithm in machine learning, top 12 artificial intelligence (ai) tools you need to know, artificial intelligence algorithms: all you need to know, what is cognitive ai is it the future, introduction to myrrix and oryx, a comprehensive guide to boosting machine learning algorithms, best generative ai learning path in 2024, top 10 skills to become a machine learning engineer, what are the prerequisites for machine learning, how to get started with chatgpt, artificial intelligence with python: a comprehensive guide, introduction to clustering in mahout, a step by step guide to install tensorflow, 10 practical generative ai examples to be more productive, how to use chatgpt for interview preparation in 2024, join the discussion cancel reply, trending courses in artificial intelligence, human-computer interaction (hci) for ai syste ....
- 2k Enrolled Learners
- Weekend/Weekday
ChatGPT Training Course: Beginners to Advance ...
- 15k Enrolled Learners
Generative AI in Business: University of Camb ...
- 1k Enrolled Learners
Prompt Engineering Course
- 5k Enrolled Learners
Artificial Intelligence Certification Course
- 16k Enrolled Learners
MLOps Certification Course Online
- 6k Enrolled Learners
Large Language Models (LLMs) Certification Co ...
- 3k Enrolled Learners
Reinforcement Learning
Graphical models certification training, generative ai in hr certification course, browse categories, subscribe to our newsletter, and get personalized recommendations..
Already have an account? Sign in .
20,00,000 learners love us! Get personalised resources in your inbox.
At least 1 upper-case and 1 lower-case letter
Minimum 8 characters and Maximum 50 characters
We have recieved your contact details.
You will recieve an email from us shortly.
Breadcrumbs Section. Click here to navigate to respective pages.

Knowledge Representation in Artificial Intelligence: An Overview
DOI link for Knowledge Representation in Artificial Intelligence: An Overview
Click here to navigate to parent product.
In real world, knowledge plays a significant role in exhibiting intelligent behavior by artificial intelligence (AI) agents. In AI, an agent can act accurately if it has past experience related to input or have some knowledge of input. In terms of machines, all the things done by humans are termed as knowledge representation and reasoning (KRR). KRR is a segment of AI that deals with thinking of AI agents and how it contributes to agents’ intelligent behavior. This chapter undertakes a basic overview of knowledge representation and how it is helpful in demonstrating intelligent behavior in AI agents, different types of knowledge, and a relation between intelligence and knowledge. This chapter also provides a detailed overview of a life cycle of knowledge in AI, basic requirements for a knowledge representation (KR) system, and different techniques and challenging issues of knowledge representation.
- Privacy Policy
- Terms & Conditions
- Cookie Policy
- Taylor & Francis Online
- Taylor & Francis Group
- Students/Researchers
- Librarians/Institutions
Connect with us
Registered in England & Wales No. 3099067 5 Howick Place | London | SW1P 1WG © 2024 Informa UK Limited
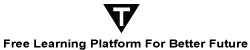
Artificial Intelligence
Control System
- Interview Q
Intelligent Agent
Problem-solving, adversarial search, knowledge represent, uncertain knowledge r., subsets of ai, artificial intelligence mcq, related tutorials.
COMMENTS
What is Knowledge Representation in AI? Knowledge Representation in AI refers to the way in which artificial intelligence systems store, organize, and utilize knowledge to solve complex problems. It is a crucial aspect of AI, enabling machines to mimic human understanding and reasoning.
Artificial intelligence (AI) is based on the core idea of knowledge representation, which tries to capture and organize knowledge in a meaningful and structured fashion. It entails archiving data and making it available to AI systems so they may learn, reason, and make decisions based on knowledge.
Knowledge representation is a vital aspect of Artificial Intelligence, enabling machines to understand, reason, and learn from information in a human-like manner. By structuring knowledge effectively, AI systems can perform various tasks, from problem-solving to decision-making, and interact with humans more intuitively.
Knowledge representation and reasoning (KRR, KR&R, KR²) is the field of artificial intelligence (AI) dedicated to representing information about the world in a form that a computer system can use to solve complex tasks such as diagnosing a medical condition or having a dialog in a natural language.Knowledge representation incorporates findings from psychology [1] about how humans solve ...
Knowledge representation is a cornerstone of artificial intelligence, enabling machines to process, reason, and act upon information in ways that mimic human intelligence. From semantic networks to probabilistic models, the variety of techniques available provides powerful tools for different applications, from natural language processing to ...
Knowledge representation and reasoning (KR&R) is a part of artificial intelligence that is solely dedicated to representing information about the real world in such a form that a computer can understand and take action accordingly. This leads to solving complex problems, such as computation, having a dialog in natural language, diagnosing a ...
Knowledge representation is a fundamental concept in artificial intelligence (AI) that involves creating models and structures to represent information and knowledge in a way that intelligent systems can use. Objects, events, performance, meta-knowledge, facts, and knowledge-base are the different kinds of knowledge.
Knowledge representation is a fundamental process in artificial intelligence (AI) that enables AI systems to comprehend and manipulate information. By structuring and organizing data in a way that mirrors human understanding, knowledge representation facilitates the reasoning and decision-making capabilities of AI systems.
Outline 1 Representation systems Categories and objects Frames Events and scripts Practical examples - Cyc - Semantic web Philipp Koehn Artificial Intelligence: Knowledge Representation 7 March 2024
Abstract. Although knowledge representation is one of the central and, in some ways, most familiar concepts in AI, the most fundamental question about it -- What is it? -- has rarely been answered directly. Numerous papers have lobbied for one or another variety of representation, other papers have argued for various properties a representation ...
Enumerating objects vs painting objects. Extend the existing behavior by adding new beliefs. Assert that canaries are yellow. Debug faulty behavior by locating the erroneous beliefs. By changing the color of sky we change any routine that uses that information. Explain and Justify the behavior of the system.
Knowledge Representation in AI describes the representation of knowledge. Basically, it is a study of how the beliefs, intentions, and judgments of an intelligent agent can be expressed suitably for automated reasoning. One of the primary purposes of Knowledge Representation includes modeling intelligent behavior for an agent.
In artificial intelligence and computational logic, two fundamental types of logic are widely used for knowledge representation: propositional logic and first-order logic. These logical systems provide the foundation for constructing and manipulating knowledge in a formal and precise manner. This article explores the key differences between proposi
In real world, knowledge plays a significant role in exhibiting intelligent behavior by artificial intelligence (AI) agents. In AI, an agent can act accurately if it has past experience related to input or have some knowledge of input. In terms of machines, all the things done by humans are termed as knowledge representation and reasoning (KRR ...
Knowledge representation is an active area of research in artificial intelligence (Brachman and Bector 2004).It often refers to the complex and time-consuming technical process performed by knowledge engineers (Knowledge Engineering) when acquiring domain knowledge for use in knowledge-based systems.The question of how to represent human knowledge is an old problem, and knowledge ...
Knowledge representation and reasoning (KR, KRR) is the part of Artificial intelligence which concerned with AI agents thinking and how thinking contributes to intelligent behavior of agents. It is responsible for representing information about the real world so that a computer can understand and can utilize this knowledge to solve the complex ...
suitable way to represent such knowledge. For this reason. artificial intelligence (AI) researchers are preoccupied with what is known as knowledge representation. According to Brachman and Levesque. (Brachman and Levesque. 1985). "[Knowledge representatlOn]. .. simply has to do with writing down. in some
Knowledge Representation Techniques in Artificial Intelligence: An Overview Koenraad De Smedt 1. Introduction ... Researchers in the field of artificial intelligence (AI) have been investigating how knowledge can be expressed in a computer system. The term which is used nowadays for the development of knowledge-intensive computer systems ...
Knowledge Representation in First-Order Logic. First-order logic (FOL), also known as predicate logic, is a powerful formalism used for knowledge representatio n in artificial intelligence and computer science. It extends propositional logic by allowing the use of quantifiers and predicates, enabling the representation of complex statements about objects and their relationships.
👉Subscribe to our new channel:https://www.youtube.com/@varunainashots 0:00 - Introduction3:58 - Logic4:20 - Rules4:28 - Semantic Net5:49 - Frame6:37 - Scrip...
Knowledge representation is an important factor in making the future AI system much better than it is today. While designing an AI knowledge representation system, there are some knowledge representation problems one has to be aware of. As mentioned before, the key is to impart wisdom to the systems, for which we require a knowledge ...
Abstract. Artificial intelligence (AI)-based solutions, knowledge representation and reasoning, and the Internet of Things applications have transformed how researchers and practitioners view the analytical and computational capabilities. The disruptive evolution of these technologies has encouraged researchers and practitioners to develop ...
Knowledge representation is a field of artificial intelligence that allows AI programs to answer questions intelligently and make deductions about real-world facts. It refers to representing information about the world in a way that a computer system can understand and use it to solve real-life problems or handle real-life tasks.
Knowledge graph (KG) embedding representation provides a foundation for knowledge reasoning and applications by mapping entities and relations into vector space. Federated learning (FL) promotes the development and application of artificial intelligence technologies by enabling model sharing and collaboration while safeguarding data privacy.