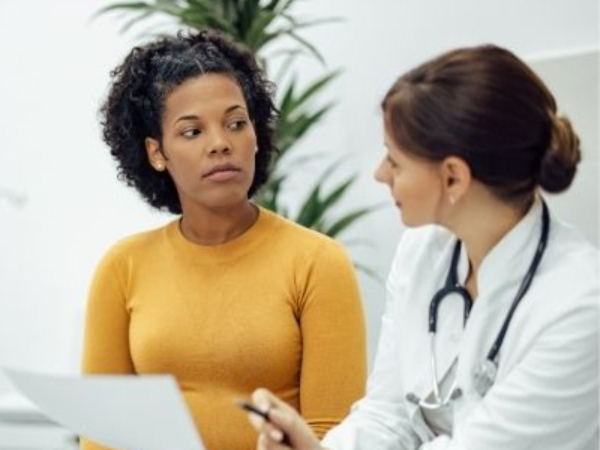
Exploring Mental Health and Diabetes Through Case Studies
These 12 asynchronous case studies include adult, pediatric, and transitioning to adults and focus on how behavioral health professionals can help people from different cultures and backgrounds manage diabetes. This program includes interactive modules with several patient videos and allows learners to practice treating people with diabetes.
LEARNING OBJECTIVES
- Identify mental health factors influencing diabetes care.
- Analyze appropriate personalized treatment plans for people with diabetes.
- Integrate behavioral health best practices into clinical scenarios using collaborative care models, team-based approaches, and effective communication strategies.
Free CE (6 APA, 6 ASWB , 5.5 COP)
EXPIRATION DATE
June 16, 2025
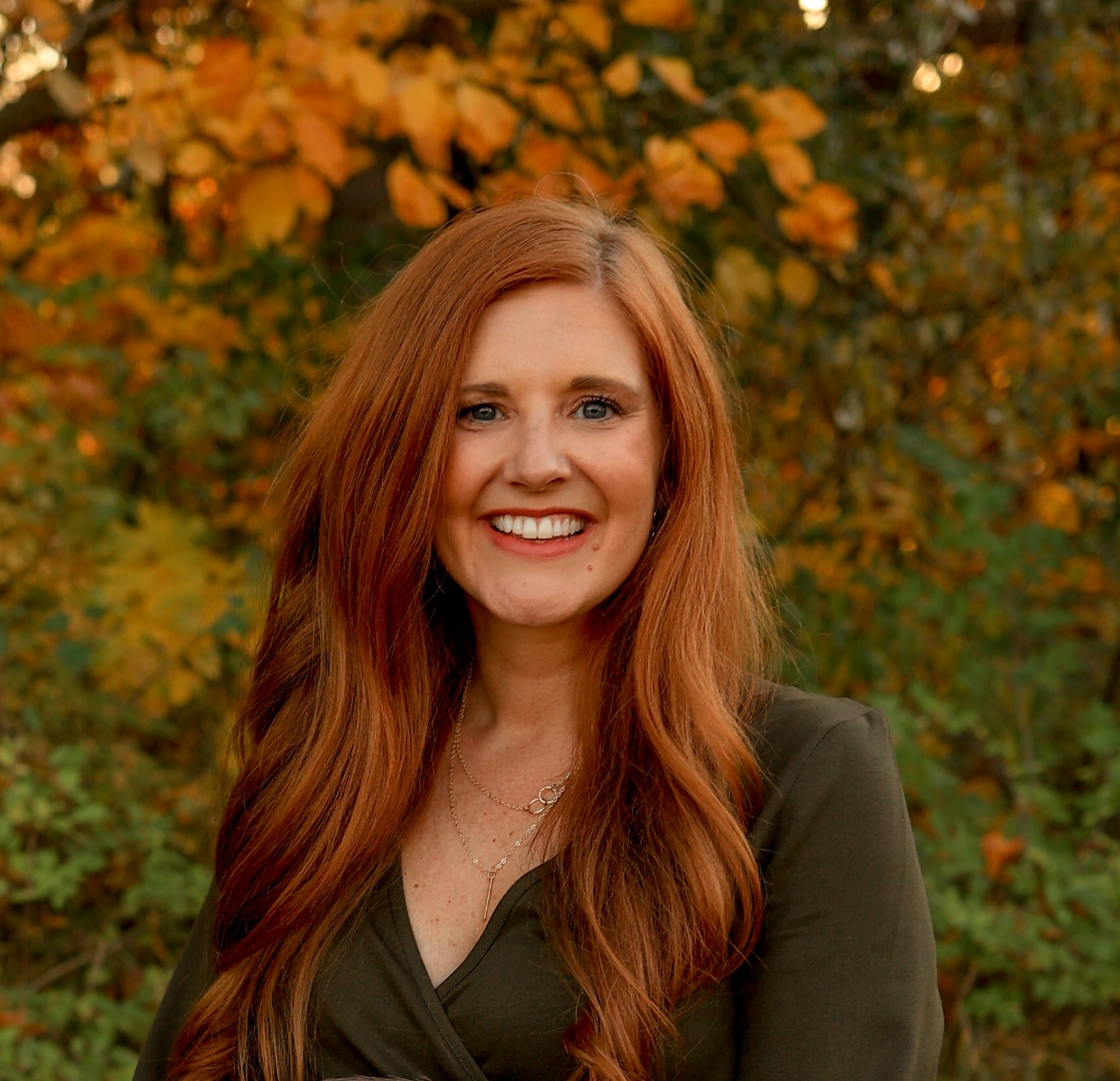
Dana Albright, PhD Clinical Psychologist & Research Scientist Mirro Center for Research and Innovation, Parkview Health
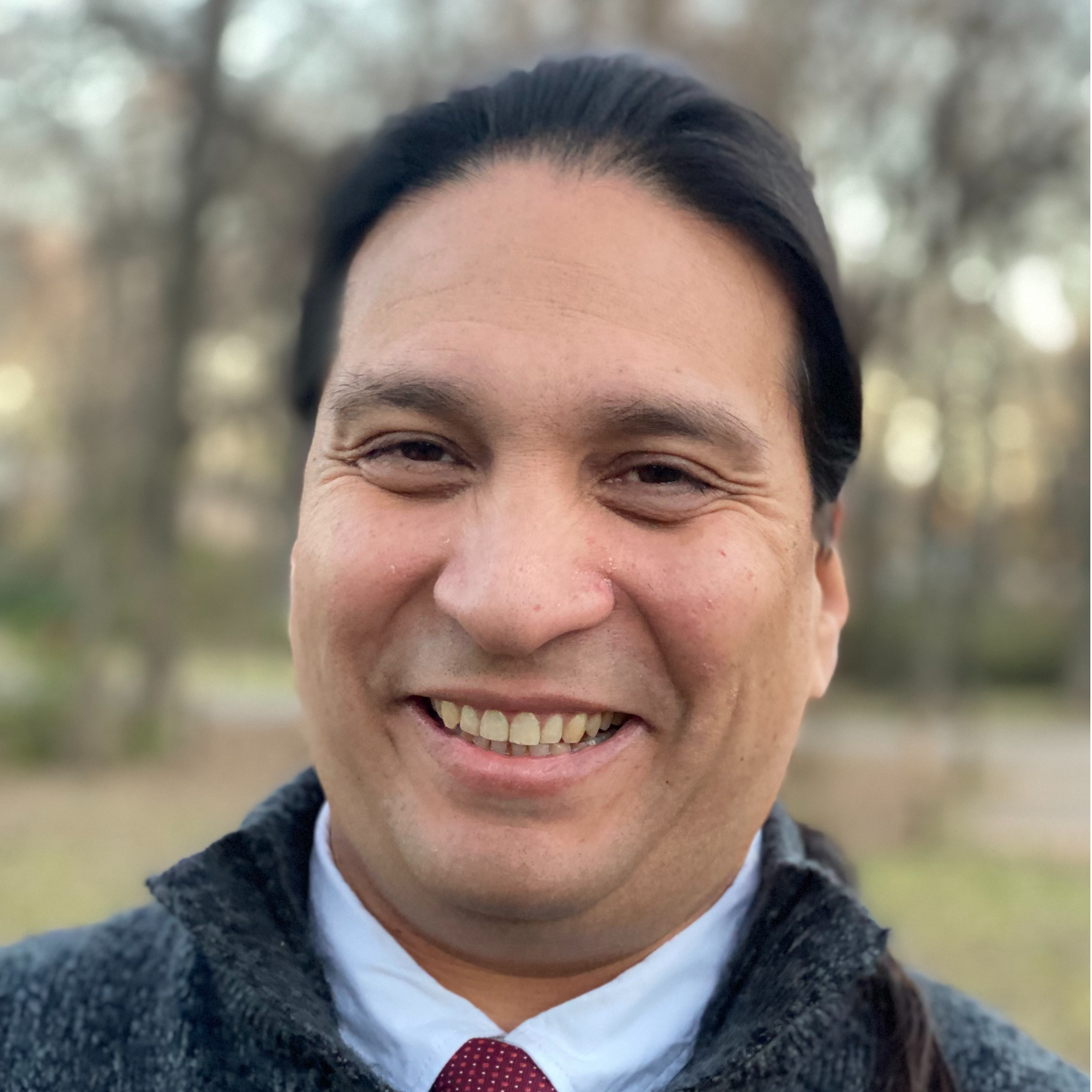
Shannon Beach, PhD Psychologist
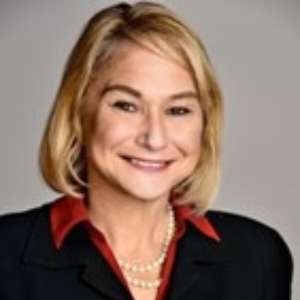
Beth Braun, PhD Health Psyschologist Chicago School of Psychology
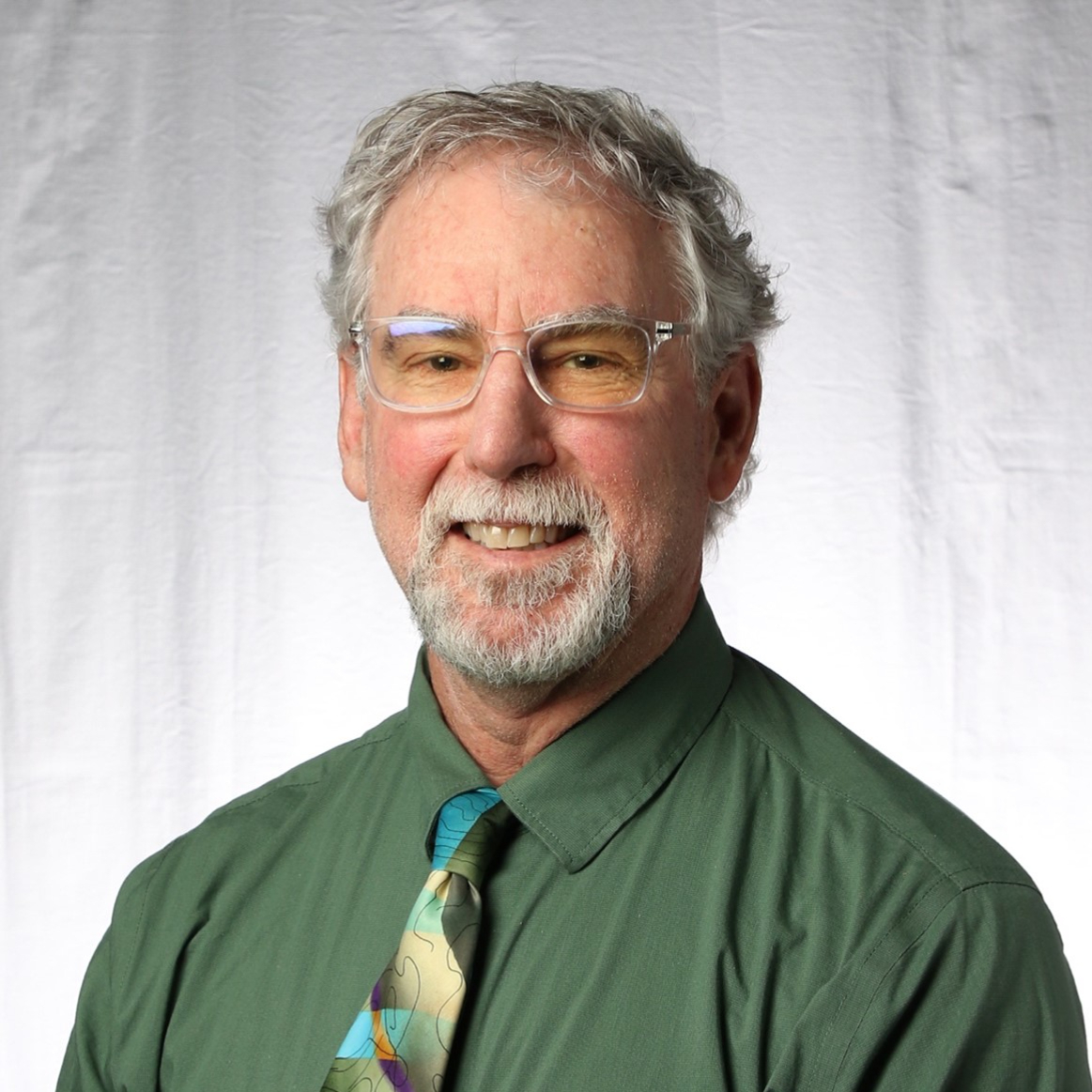
Michael Harris, PhD Professor, Pediatrics Oregon Health & Science University
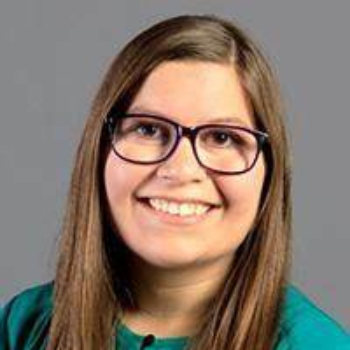
Allyson Hughes, PhD Assistant Professor Ohio University Heritage College of Osteopathic Medicine
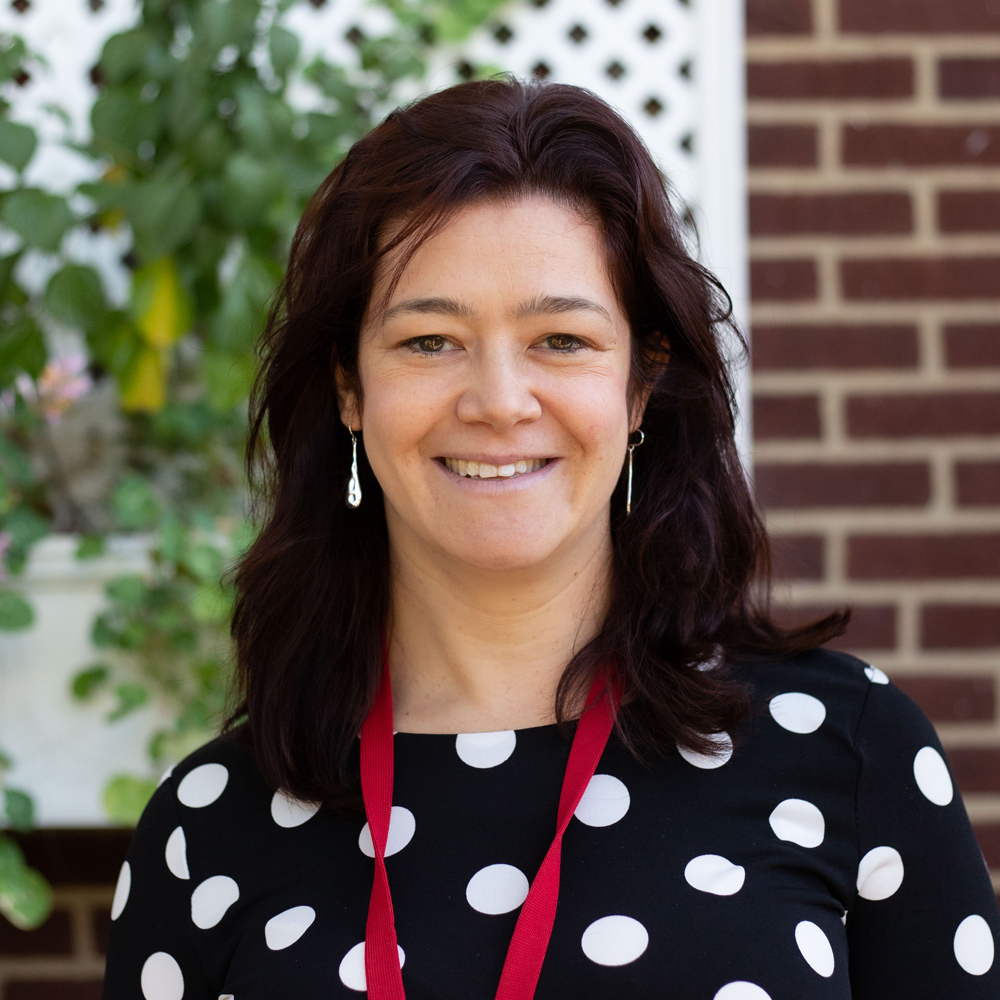
Dana Lehman, PsyD Director of Mental Health Education Programming AIDS Care Group
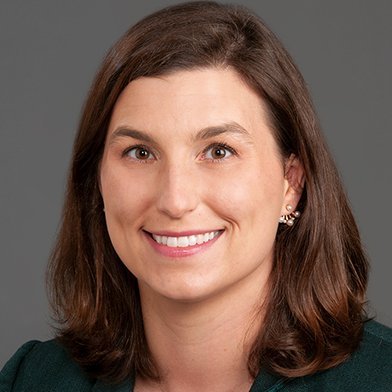
Katharine McLean, PhD, MS Licensed Psychologist/Health Service Provider
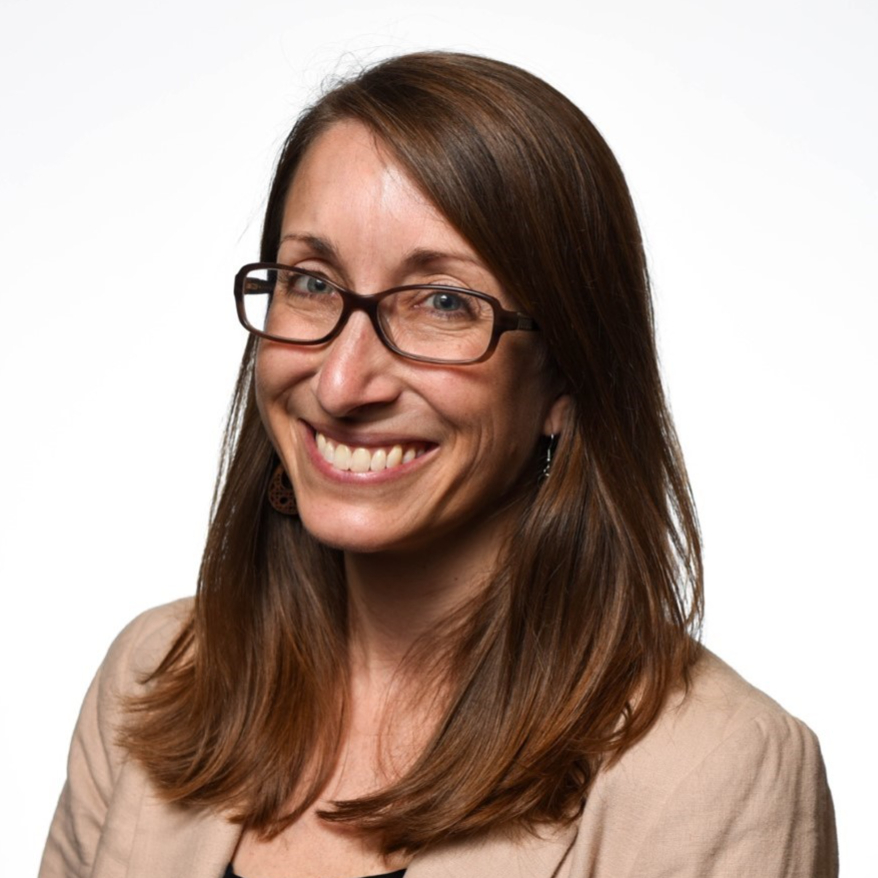
Maureen Monaghan, PhD, CDCES Program Director, Diabetes Behavioral Science Division of Diabetes, Endocrinology, & Metabolic Diseases NIH/NIDDK
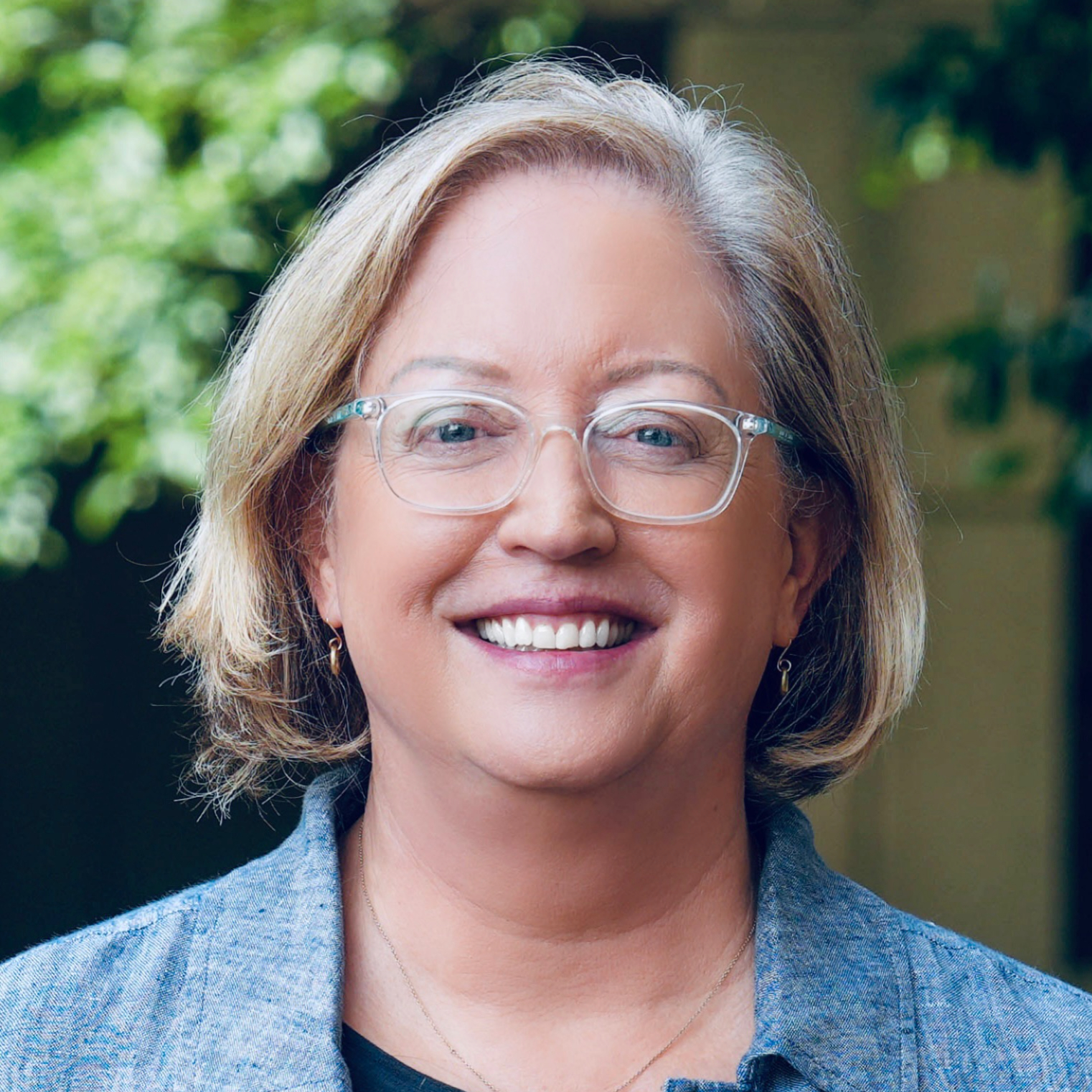
Shelagh Mulvaney, PhD Associate Professor Vanderbuilt University
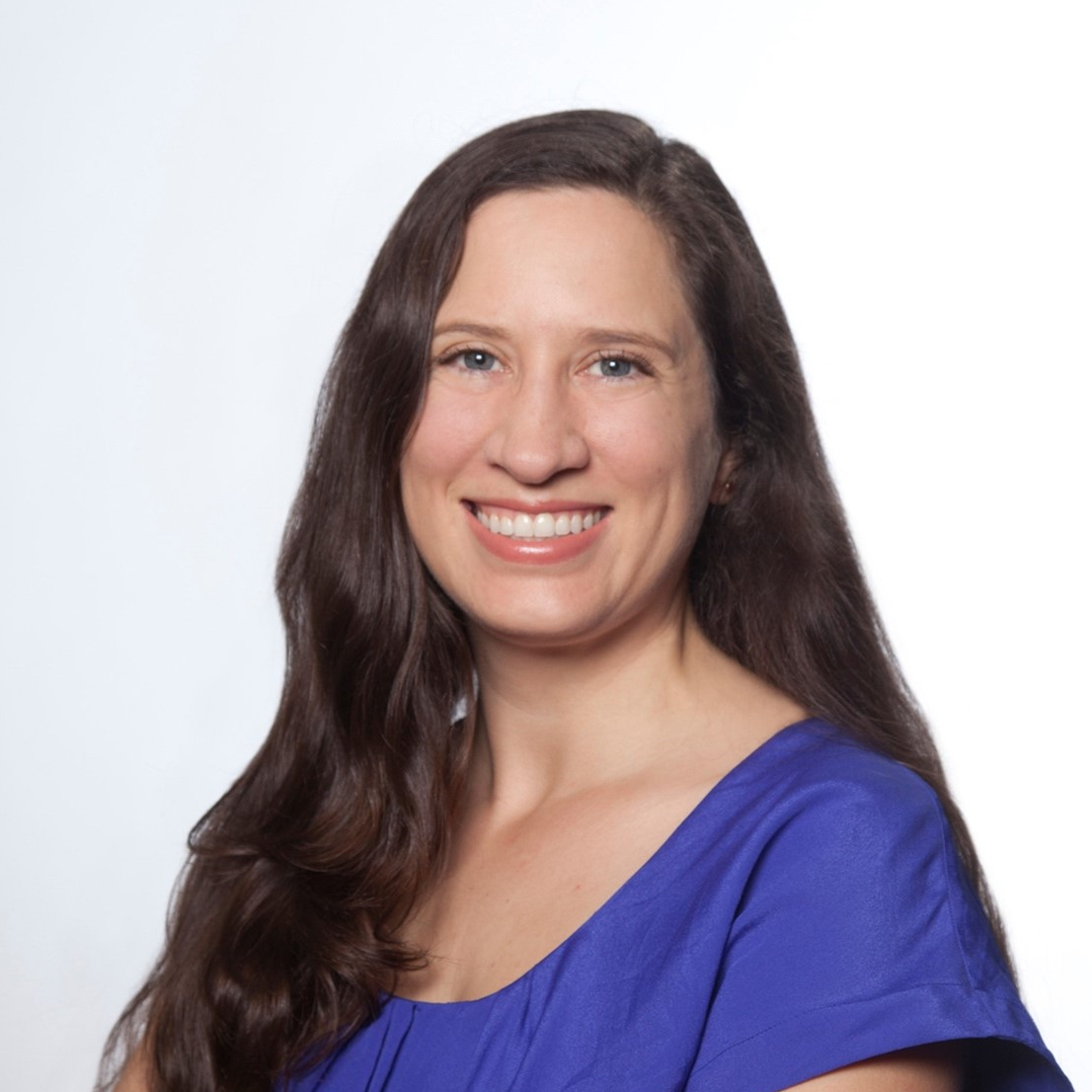
Diana Naranjo, PhD Clinical Professor of Pediatrics Stanford University
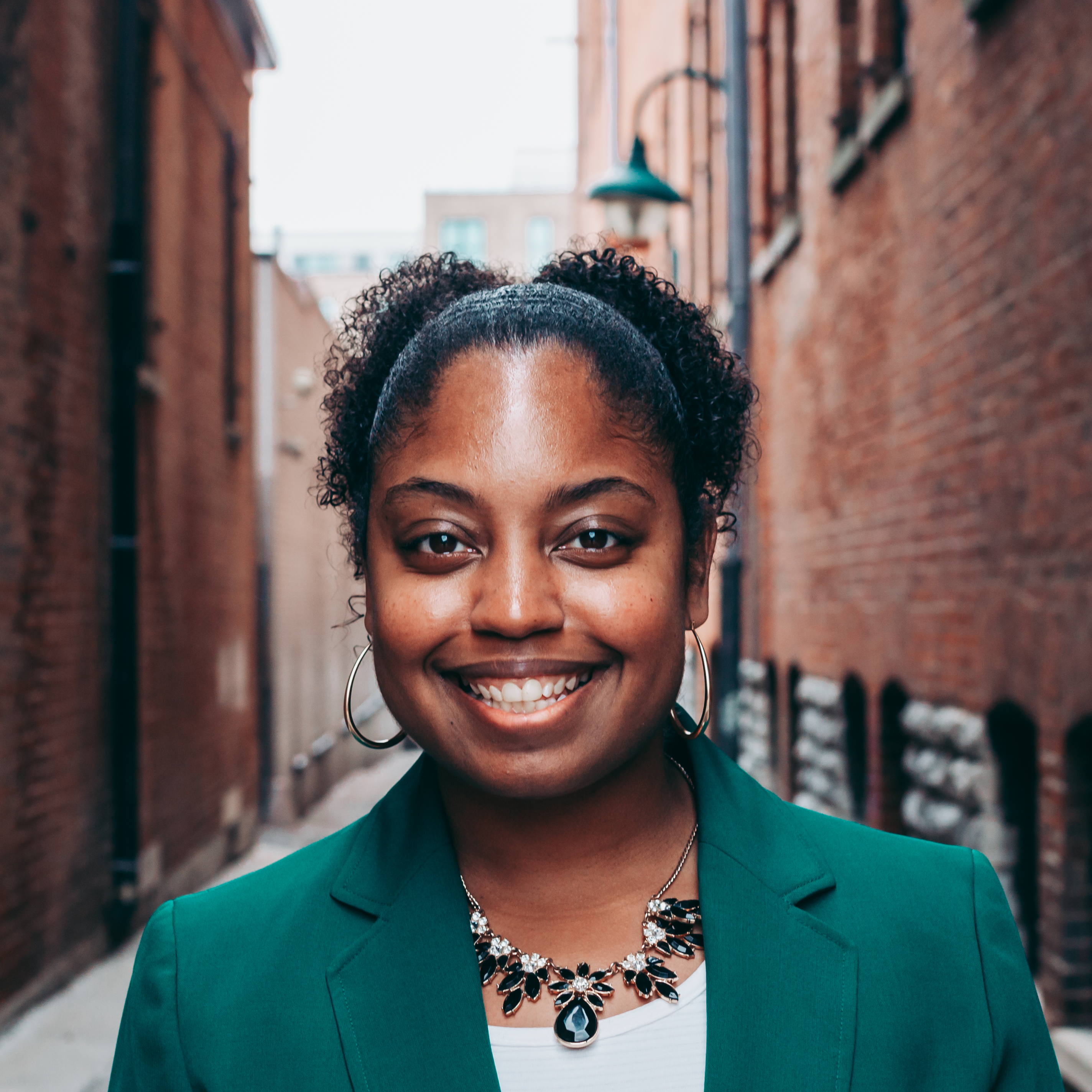
Desiree Williford, PhD, MPH Postdoctoral Research Fellow Cincinnati Children's Hospital Medical Center
This program is supported, in part, by unrestricted educational grants from The Leona M. and Harry B. Helmsley Charitable Trust.
Note: You do not need to be an ADA professional member to access our free continuing education programs. Simply create a free account and start exploring 70+ free CE courses. Login to get started!

Advertisement

Diabetes and conversational agents: the AIDA project case study
- Open access
- Published: 22 September 2021
- Volume 1 , article number 4 , ( 2021 )
Cite this article
You have full access to this open access article
- Francesca Alloatti 1 , 2 ,
- Alessio Bosca 1 ,
- Luigi Di Caro 2 &
- Fabrizio Pieraccini 3
5974 Accesses
4 Citations
5 Altmetric
Explore all metrics
One of the key aspects in the process of caring for people with diabetes is Therapeutic Education (TE). TE is a teaching process for training patients so that they can self-manage their care plan. Alongside traditional methods of providing educational content, there are now alternative forms of delivery thanks to the implementation of advanced Information Technologies systems such as conversational agents (CAs). In this context, we present the AIDA project: an ensemble of two different CAs intended to provide a TE tool for people with diabetes. The Artificial Intelligence Diabetes Assistant (AIDA) consists of a text-based chatbot and a speech-based dialog system. Their content has been created and validated by a scientific board. AIDA Chatbot—the text-based agent—provides a broad spectrum of information about diabetes, while AIDA Cookbot—the voice-based agent—presents recipes compliant with a diabetic patient’s diet. We provide a thorough description of the development process for both agents, the technology employed and their usage by the general public. AIDA Chatbot and AIDA Cookbot are freely available and they represent the first example of conversational agents in Italian to support diabetes patients, clinicians and caregivers.
Similar content being viewed by others
Healthcare Conversational Agents: Chatbot for Improving Patient-Reported Outcomes
Artificial Intelligence for Chatbots in Mental Health: Opportunities and Challenges
Conversational Agents for Mental Health and Wellbeing
Explore related subjects.
- Artificial Intelligence
Avoid common mistakes on your manuscript.
1 Introduction
Natural Language Processing has significantly improved over the past years, thanks to the development of new and powerful Artificial Intelligence algorithms and computational methodologies. Results of this improvement include the growth of conversational technologies in specific fields, such as healthcare [ 1 , 2 ]. However, healthcare is a broad domain and not all conditions or situations are equally represented in the Conversational AI landscape. Diabetes is one of many topics that has been only partially tackled by computer science.
The standard treatment for diabetes poses several goals and challenges [ 3 ]. For instance, the preservation of adequate blood glucose values, expressed by the levels of glycated hemoglobin, has been associated with a lower risk of disease related complications compared to progressively increasing blood glucose levels [ 4 , 5 , 6 ]. One of the goals of treatment is the prevention of complications. However, the employment of numerous—and effective—pharmacological therapeutic strategies cannot ignore the factor of patient satisfaction: their quality of life, both objectively measured and subjectively perceived, is an extremely important component in the process of care.
Unfortunately, despite the availability of innovative drugs and medical devices, a high proportion of people with both type 1 and type 2 diabetes fail to reach the desired therapeutic targets [ 7 ]. This issue questions the standard approach to patient management and it has a significant economic impact on health systems too [ 8 , 9 ]. In this context, one of the key aspects in the process of caring for people with diabetes is Therapeutic Education (TE) [ 10 ]. TE is a teaching process that aims to train patients to self-manage the aspects of their life related to the illness [ 11 ]. Autonomy in care management allows patients to reach a new level of well-being; it is also a more flexible way of approaching the process, as it can be adapted as the disease evolves, or to changes in people’s way of life. Such educational interventions are always aimed at improving patients’ knowledge and practical ability to manage their care plan. The final goal is to modify their behaviors until a balance is obtained between the patients’ needs and the constraints of the disease. The goal of TE is therefore to make patients with diabetes as conscious and autonomous as possible in the management of glycemic measurements, in the choice of the most appropriate therapy (for example the insulin dose) and in the management of any acute complications related to the disease.
Alongside traditional methods of providing educational content, patients and caregivers can now enjoy new alternative forms of information transmission. Nowadays, people with diabetes may use technological support such as websites, apps, or digital assistants. Artificial Intelligence (AI) applications can provide assistance in a personalized way and can amplify the machine’s ability to connect different medical sources, thus providing patients with new tools to obtain and manage information regarding their condition [ 12 , 13 , 14 ]. In the healthcare domain, one of the most promising benefits of AI technologies is their ability to create new forms of dialogue systems. Those systems, also known as conversational agents (CAs), are equipped with the ability to understand natural language and manage complex information exchange [ 15 , 16 , 17 ]. TE can now be delivered through the tool of human-machine dialogue which brings a series of benefits: patients can obtain information autonomously, simply by interacting with a chat interface; CAs are usually available at every hour, while a doctor may not always be available for a rapid consultation; moreover, patients who interact with a machine may be more inclined to ask about sensitive topics that they do not feel comfortable addressing with a person [ 18 , 19 ].
In this article we present the Artificial Intelligence Diabetes Assistant (AIDA) project, which includes two CAs designed and built with the explicit goal of providing patients with an innovative tool to improve their access to therapeutic information. Specifically, our contribution consists it:
Highlighting the design process upon which AIDAs are built. Especially in the healthcare domain, CAs should be built with particular attention towards their potential users. We employed established techniques in the human–computer interaction domain to inform the design;
Outlining the architecture that empowers the system’s reasoning. AIDAs exploit both machine learning capabilities and a rule-based engine. We believe that the balance of these two modules constitutes the best approach for this kind of CAs and their description is a unique insight into industrial technologies for conversational AI;
Providing the system’s usage data. AIDAs were made available to the public during the year 2020. Our first goal was to ascertain the usability of such tools within the diabetic population of Italy and their reaction to CA technology. We present usage data gathered in the wild and provide insights into the behavior of the users when interacting with CAs for TE.
The case study offers a novel perspective at the crossroad of two disciplines: therapeutic education and conversational AI. Our goal is to provide the scientific community with information about industrial CAs, which are often developed solely on private company premises and the features of which are usually not revealed to the public.
Artificial Intelligence Diabetes Assistant (AIDA) is a chatbot delivered via a written channel as well as a voice-based assistant conveyed via an Alexa Skill. Footnote 1 For the text-based CA, we refer to it as AIDA Chatbot; in the second case, as AIDA Cookbot. They are AI-based conversational systems devised by the pharmaceutical company Novo Nordisk and created by computational linguists from the NLP company CELI, with the support of a scientific board. Both AIDAs use the Italian language and are mainly directed to Italian speakers.
The voice-based agent focuses on the diet regime theme and provides recipes for users. It was deemed that a speech system would be more engaging and effective in delivering this kind of content. The text-based agent, on the other hand, has a broader scope: it answers numerous questions about diabetes and offers help for people with type 1 and type 2 diabetes, caregivers and general practitioners. Since its goal is to answer questions, rather than engaging the user, the written channel was deemed to be more appropriate.
The goal for both is to generate awareness about diabetes among the general public and help those who have already been diagnosed to easily obtain information and advice about everyday questions. Especially during the COVID-19 pandemic, the tool would help people obtain secure information in an autonomous way, as well as help clinicians to focus on more pressing issues while delegating simpler educational tasks to a machine [ 20 , 21 ].
The article is structured as follows: in Sect. 2 we provide some background information by analysing related work and the differences between previous studies and the AIDA project. In Sects. 3 and 4 we describe the start of the project: specifically, how the initial knowledge base for both the chatbot and the voice-based assistant was created, its adherence to scientific principles, and the User Experience precepts that guided the whole project. AIDA is built upon proprietary software integrated with external endpoints when appropriate. Its architecture is described in Sect. 5 . In Sect. 6 the authors describe the technology behind the Conversational Agent: the components that allow the reasoning to take place and its relevant infrastructural details. Once the CA was built, it was made available to the public by means of a dedicated landing page, a third-party chat system (Telegram) and through Amazon Alexa. Our goal was to provide patients and caregivers with ready to use tools as soon as possible during 2020. Section 7 describes the release of the system in production and provides data about its usage and performance. The article ends with a Conclusion and prospects for the future path of AIDA and Therapeutic Education Technologies.
2 Related work
Conversational interfaces allow humans to interact with devices using “natural language”, that is, the set of words and structures that humans use daily to express themselves. Instead of communicating with the machine through a formal system that the computer can understand (like a programming language), the user can speak or write freely, delegating the interpretation of the message to mechanical components [ 22 ]. The application of CAs in the healthcare sector is a relatively recent phenomenon, in fact most of the studies have been carried out starting from 2010 [ 23 , 24 ]. Since then, however, there has been a steep increase in research and publications [ 25 , 26 ]: several experiments have introduced CAs that help patients and doctors manage the treatment process more effectively.
With regard to patients, CAs are tools that provide health information and help patients manage their drug therapy or comply with physicians’ instructions [ 27 , 28 ]. These interfaces can also help doctors, for example by conducting medical history interviews, thus relieving the amount of repetitive and impersonal work [ 29 ]. The various conversational systems can be categorised according to certain characteristics [ 23 ]: the type of input they accept (written, spoken, or both); whether the action is agent-driven or user-driven (i.e. whether the system asks questions to guide the user or vice versa); on which platform they are hosted (a dedicated app, a web interface, a bot on a messaging application, etc...); their purpose (chat-oriented or task-oriented). It is useful to note that most of the interfaces in the healthcare field are currently task-oriented agents—that is, they help patients, doctors or caregivers carry out a task—rather than chat-oriented agents whose only goal is to perform casual conversation.
2.1 Conversational agents and diabetes
In the domain of diabetes, some studies on educational tools to facilitate pathology self-management have been carried out [ 30 , 31 , 32 , 33 ]. However, most of them concern either type 1 or type 2 diabetes patients, while our focus is on both: as will be detailed in Sect. 3 , one of the requirements was to design a system that would cover questions from both types of patients. Many studies either concentrate on the information needs of patients [ 34 , 35 ], or the building of an AI-based agent [ 36 , 37 , 38 ], rarely both. Although some studies focus on the theme of recipe recommendation [ 39 , 40 ], the recipes are not specific to people with diabetes or they are not delivered by means of a voice-based agent.
Other studies concern therapeutic education for diabetic patients but are not CAs [ 41 , 42 ], or they are CAs but not specifically aimed at diabetes patients [ 43 ]. The first premise of our work was to provide patients with a conversational tool. One of the closest studies to ours is by Stephens et al. [ 38 ]. They illustrate Tess, a support tool for obese children in a pre-diabetic stage. Tess is also equipped with a pass-to-human feature that appears to be seamless, without the users being aware of the switch between machine and human. AIDA does not involve a pass-to-human intervention at any of its conversation stages; the only human intervention comes into play during the Validation task. This decision has the clear purpose of experimenting with the agent only, measuring its performance only afterwards and not making any human corrections mid-interaction.
Wang and colleagues [ 35 ] describe the process of classifying users’ questions about diabetes. The questions were obtained by crawling 39 Health , a popular health related website in China. The crawling approach differs from that of AIDA, where the KB was manually created by the scientific board, while it is more similar to the work by Crangle et al. [ 44 ]. Moreover, AIDA’s KB is in Italian, differentiating this work from previous ones [ 45 ]. By obtaining questions from a website, Wang and colleagues managed to capture from the very beginning all the different linguistic forms employed by real patients. However, the classification tasks alone would start from spurious data, slowing down the whole development process. Moreover, the linguistic features clinicians apply when talking to patients are specific and they should be applied when chatbots are conversing with patients [ 46 ]. Correct use of medical language has been proven to be essential to the positive outcome of the treatment [ 47 ] and a CA should use the same terminology that is used by clinicians. The DI@L-log agent described by Black et al. [ 8 ] even poses as a nurse. In our case, the scientific board thought that it would be unethical to imply that AIDA had the same capabilities as a human clinician.
2.2 Multimedia systems
Some systems exploit multimedia content along text-based exchanges, such as voice, videos or images [ 37 ]. For instance, Emmi appears to be a ready-to-use web app that provides text and multimedia content [ 36 ]. However, it is not clear whether Emmi is a conversational assistant (since it answers questions via voice), or whether it consists of a Graphical User Interface enriched by audio and videos.
Frøisland and Årsand [ 34 ] propose an application made of two components. The first is a mobile app that allows users to take a picture of their meal; by doing this, patients create a diary of their own eating habits, thus making themselves more aware of their food consumption. The second component is a SMS communication protocol established between patients and their care provider. The process is fully managed by humans though, while in this context a CA could automatize the communication protocol.
Maharjan and colleagues [ 48 ] propose an Alexa-based technology to deliver information about nutritional values specifically for diabetic patients. This work is particularly interesting because it demonstrates the feasibility of such an intervention and therefore the scientific soundness of AIDA Cookbot. However, their Alexa skill is in English and aimed specifically at the Native American population. In our case, we refer to the Italian public, which has different eating habits. Moreover, their system recommends recipes but does not guide users in the actual preparation of the meal.
In general, CAs in the healthcare domain are a constant presence, as are studies aimed at enhancing Therapeutic Education for people with diabetes. The intersection between these two domains, however, has not yielded many results yet.
3 User experience design of the conversational agent
In order to build agents compliant with the final users’ expectations, the design process takes into consideration requirements expressed by the main stakeholders of the project: patients and diabetologists. Patients are framed as the future main users of the systems, while diabetologists are the ones that, thanks to their medical expertise, will provide the KB (Knowledge Base, i.e. the content of the agents). Their needs and suggestions guided both the construction of the KB as well as the type of interaction that the agents would conduct with their users.
3.1 Requirements gathering
The AIDA project began with a series of interviews. This methodology is widely used in the domain of human–computer interaction for CA, where qualitative interviews provide meaningful insights into the expectations and the mental model of future users [ 49 , 50 , 51 ].
The interviews had two purposes: first, to clarify the scenario within which the CA will have to be situated in terms of perception, expectations and desires; second, to find out what themes users would like to discuss with a CA [ 52 ]. The interviews were structured in the form of two interactive workshops. In the first workshop, the participants were eight diabetologists while the second workshop involved eight people with diabetes type 1 or 2.
Each group was balanced according to three criteria:
Gender and age —in the first group there was a slighter predominance of males (M = 5, mean age = 40.4), while between patients, the majority identified as women (F = 6, mean age = 38.3).
Inclination to use digital tools —the majority of clinicians declared they were inclined to rely on digital tools, either because they recognized they could empower patients’ independence (N = 3), or because they considered them to be more practical than paper-based systems (N = 3). The remaining two expressed more skeptical views. Between patients, the inclination correlated heavily with age: patients were comfortable with digital devices in the range 25–40 years (N = 3) and in the range 41–60 years old (N = 2); in the range 61–80 (N = 3) they were less comfortable.
Type of diabetes —this criteria is only applicable to the second group. Older people had type 2 diabetes (N = 5), while younger ones type 1 (N = 3).
Figure 1 summarize the scope and features of the two workshops.
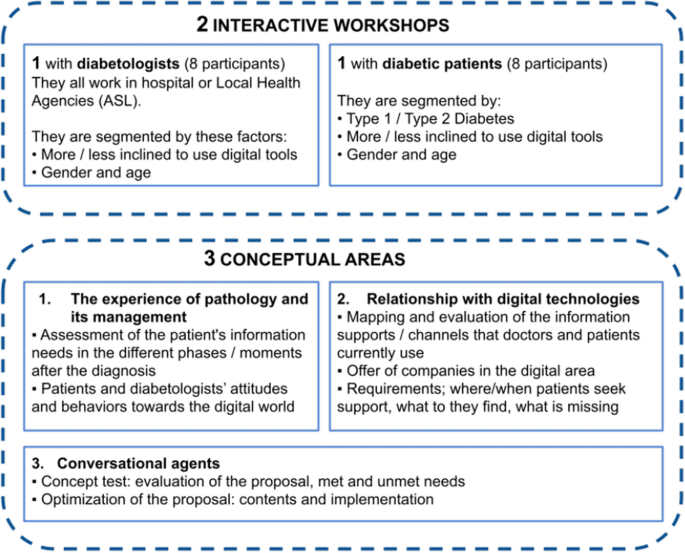
Participants and goals of the two interactive workshops
The workshops tackled three main conceptual areas that are relevant to both parties: (i) the experience of pathology and its management; (ii) doctors and patients’ relationship with digital technologies; (iii) thoughts about the proposal of a CA. Results were extracted by means of qualitative interviews.
In the first area, the two groups agreed on the need for patients to understand the pathology and all its consequences, so that they can absorb the necessary information to manage the pathology and control any possible outcome. During the time that follows the diagnosis, patients need to be reassured about the new challenges and doubts they are now facing. The questions that patients pose to their diabetologists relate to everyday aspects of their life: lifestyle advice, such as what kind of sport is permitted, how often, which food is better to eat and when to schedule one’s own meals. In this phase, patients feel insecure and often seek psychological support to help them accept the pathology and the social adjustments that are necessary. While on one side diabetologists need to inform persons in a reassuring but also firm way, on the other side patients need to resolve all their doubts, even minor ones, in order to accept their new reality.
Diabetologist: the most frequent question I get at the beginning is “Will I ever be cured?” Patient: why did it happen to me? Nobody in my family has diabetes...
In the domain of digital technologies, both doctors and patients’ perceptions change according to their age group. While there is a general familiarity with technological instruments (some of which are an everyday necessity, such as the glucometer), younger generations feel more comfortable with them: they look for apps and IoT devices that can help them monitor their situation and share their data directly with their physician. In general, all groups use internet search to look for answers to their doubts, although younger patients profess to using only specialist sites because they do not trust any information that may come up from a Google search or in a Facebook group. All age divisions agree on the need to receive verified information through trustworthy channels that can be reached from various endpoints, without any discrepancies between a channel and the other.
Diabetologists, on the other hand, feel less comfortable with a plethora of digital devices, as they see potential privacy issues as well as an increased burden on their part to gather and analyse data from different sources. For them, ease of use, security in data management and re-use of existing applications is an essential requirement of a new technology.
Diabetologist: it’s an issue of time and resources, most of the time the devices will not simplify my job, although they do help to monitor the patients Patient: I look up special recipes on YouTube; [Internet] is ok, but you can find all sort of true and fake information there
Finally, doctors and patients expressed their views about a CA that would provide information about diabetes. Both parties declared their need for a generalist tool, a system that would contain all sorts of information about pathology and the answers to all those lifestyle doubts that emerged before. Moreover, they wanted the agent to provide psychological support in the form of positive reinforcement and personalized motivational messages. They all agreed that a certain degree of customization would be preferable, even though that would imply sharing personal information.
Patient: knowing how many carbs a certain food has would be helpful. For instance, I didn’t know that even tomatoes have carbs...
The insights gained via the interview produced a detailed report of the requirements and expectations of both stakeholders.
3.2 Design guidelines
The design guidelines were informed by the report produced after the workshops: the needs and suggestions that emerged during the interviews were used as dos and don'ts to create both CAs. To our knowledge, this is one of few cases where the design guidelines were informed by interviews and at the same time they were empirically applied to the construction of two different CAs [ 49 , 53 , 54 , 55 ].
The first area indicated that the agents should be a reassuring presence, trustworthy and reliable from a medical point of view. Their internal knowledge should reflect that of the diabetologists and their tone of voice should be firm. Since many patients also need psychological support after the diagnosis, the agents should employ empathetic language and encourage them to reach out to family, friends and professional help if they express any discomfort.
The second area expressed various preferences in terms of devices, technologies and privacy. Apart from the soundness of the information that the agents would give, which was always considered as a paramount guideline, the workshops instructed the design of an agent that could be used from any device and that did not collect any personal data about the user. Ease of use and availability was one of the requirements suggested by the clinicians; by deploying the agents on a website, a free and popular messaging app and one of the most known voice-based platforms in Italy, we met those needs. The opportunity of both a text-based and a voice-based interaction also met the demands of the different age groups, that may find one agent or the other easier to use according to their familiarity with new technologies. Even though patients desired personalized interactions with the agent by sharing some of their data, it was deemed best not to collect any personal information about the users in order to comply with pharmaceutical regulations. Therefore both CA are agnostic to users and the interactions do not take into account any external information.
Ease of use piloted AIDA Chatbot towards user-driven interaction, where the user would be free to ask even impromptu questions without the need to engage in a complex and guided dialogue session. AIDA Cookbot, on the other hand, was created as agent-driven interaction because of the different communication channel it would exploit: via voice, it is important that the agent instruct users on what to say next in order to benefit from the CA’s content.
4 Construction of the knowledge base
The results of the interview stated the principles that were later followed in the design of the CA’s KB. The KB was built during a co-design workshop, where a scientific board drafted the content guided by three facilitators and two computational linguists. Unlike previous work [ 33 , 35 ], AIDAs’ KB was built manually, in order to assure that both questions and answers were scientifically sound as well as linguistically correct.
The scientific board was composed of four diabetologists, four specialists from the pharmaceutical company, and a psychologist. The presence of these different figures assured medical accuracy and adherence to pharmaceutical regulations. The psychologist in the group advised the board on the themes that were important to address according to the interviews as well as the tone that was appropriate to use while responding to certain doubts, some more delicate than others [ 56 ].
The scientific board agreed that the most important issue was the personal safety of the user: no patient or caregiver should ever find herself in a dangerous situation because of the information provided by the agents [ 57 ]. Moreover, no data exchange with other systems or request for personal data from the patient should be allowed, in order to meet the diabetologists’ concerns for privacy. AIDA would not be a predictive tool, therefore it could not be a medical device. As such, it could never provide treatment instructions or information about medication. It should be clearly stated that the agents are not a substitute for medical advice.
While the text-based AIDA would answer questions related to diabetes from a broad set of themes, AIDA Cookbot focusses on the food theme by supplying recipes that are compliant with a diabetic regime. Therefore, the board was tasked with the creation of the Q&A corpus, as well as with the recipe corpus.
4.1 AIDA Chatbot’s KB
During the workshop, the multidisciplinary team selected four main themes that were deemed particularly important:
Diagnosis—all the information about symptoms, cures and statistics on diabetes;
Lifestyle—this section encompasses subjects such as sport, habits and all the services that are available for people with diabetes: fiscal exemption, measures for children in school, etc.
Diet—one of the major issues for patients is the kind and quantity of food they are allowed to eat. This section aims to debunk false preconceptions about the strict connection between diabetes and diet habits, and supply sound information about correct food consumption.
Prevention and complications—information about the prevention of diabetes as well as the possible consequences of a disease that, if not properly managed, can lead to severe consequences.
For each of these themes, the scientific board drafted several questions that the user may ask and wrote the answers. The psychologist revised those answers in order to make them empathetic, respectful of the emotions of the patients. Table 1 presents all the main themes with their sub-themes, the number of questions each one contains and a couple of examples for each category. Figure 2 presents some example dialogues.
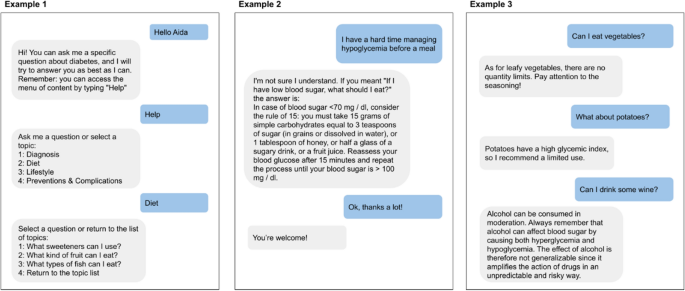
Some example dialogues. In Example 1, the user decides to consult AIDA by interacting with the content menu. In Example 2, the user asks a very specific question and AIDA tries to match it to the closest one in its KB. In Example 3, the user asks a series of questions related to the same topic. AIDA keeps track of the current topic in order to answer appropriately. The dialogues, originally in Italian, have been translated into English for the purpose of this article
The first KB thus created contained roughly 120 questions, each with its own answer. The final KB shown here was expanded by including suggestions and comments from other doctors that tested AIDA while still in development, resulting in a total of 170 questions. The agent available on the web page and on Telegram both refer to this KB, while the voice application, available on the Amazon Alexa Skill platform, has its own content.
4.2 AIDA Cookbot’s KB
One of the main subjects of interest that emerged from the interviews and the workshops is the diet theme. Food consumption is one of the major preoccupations for people with diabetes of both type 1 and type 2 [ 58 ]. For this reason, the scientific board decided to base the voice agent around food education by creating a system that provides recipes with a low glycemic index. The recipes were created by the medical personnel, thus ensuring the content’s reliability [ 14 ]. AIDA Cookbot’s KB consists of 61 recipes distributed in four categories: starters, first courses (pasta, rice), second courses (meat, fish) and desserts. While the board provided the recipes, the linguists on the team designed the interaction following the principles of voice-based human-machine interaction. The interaction is agent-driven and follows a series of steps:
Step 1—The agent asks users if they have any allergies. This information is stored and used to exclude recipes that contain those allergens.
Step 2—The agent asks what users would like to cook. No restrictions are imposed on the input: people might say an ingredient (e.g. I would like to cook some rice ), a type of course ( Can you recommend me a first course? ), or a characteristic they would like ( I need a vegetarian recipe , What about something easy to cook? ).
Step 3—The agent proposes a recipe based on users’ preferences. Users can accept it or ask for a different recipe. Once users find the recipe they want, the agent will proceed to list the ingredients. Diabetic patients can then proceed to the various steps of the recipe procedure, or hear the nutritional values. This latter information was deemed particularly important, given the dietary constraints imposed by the pathology. As the recipe is followed, users may ask to jump to the next step, to repeat the previous step or start again from the beginning.
Unrestrained step—At any time, users may interrupt the flow by asking a general question about the Diet theme (e.g. Am I allowed to eat candy? . The diet theme questions present in AIDA Chatbot were indeed included in AIDA Cookbot in order to obtain a certain homogeneity between the two agents. Once the agent has answered the questions, it will prompt the user to come back to the main dialogue flow.
Figure 3 shows some example dialogue with AIDA Cookbot. AIDA Cookbot is delivered as an Alexa Skill integrated with proprietary software for the interaction model component. More detail about the Skill’s architecture can be found in Sect. 5 . The agents are made available for free on a website, Footnote 2 on Telegram Footnote 3 and on the Amazon Alexa Skill store. Footnote 4
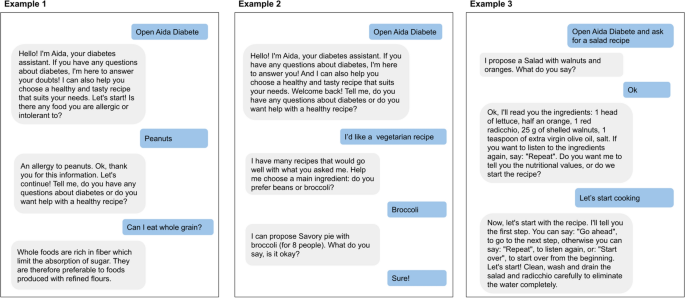
In Example 1, the user interacts with AIDA Cookbot for the first time and the agent asks for any allergy, in order to memorize it. Users can ask a Diet-related question or for a recipe. In Example 2, the user returns for a second interaction and expresses a preference for a recipe. AIDA guides the user towards something more specific. In Example 3, the user asks directly for a recipe and AIDA immediately proposes something related to the request
5 Conversational agents’ architecture
Both AIDA Chatbot and AIDA Cookbot were created by the same team from CELI company exploiting internal resources and proprietary software called Sophia Chatbot described in [ 59 , 60 , 61 ]. However, in order to create a voice-based experience, it was necessary to integrate the original software with one of the available platforms for building voice applications, Amazon Alexa. Therefore the two projects share some components but their architecture differs, according to their functioning and their endpoint. Specifically, AIDA Cookbot includes a connection with the Lex platform as well as the integration of a proprietary search engine.
5.1 AIDA Chatbot’s architecture
The architecture of the chatbot is composed of various modules that are integrated in a complete service. The backend includes the functionalities of Natural Language Understanding, Natural Language Generation and Dialogue Manager. The NLU module aims to understand the user input. The understanding operation can be defined as a classification task; details about the NLU module can be found in the next section. For architectural description purposes, it is sufficient to say that the NLU module classifies the user’s input according to its internal KB (described in the previous section). The NLG module is in charge of producing an answer to the question asked by the user. It either retrieves the appropriate response from a predefined set, or it can generate it dynamically from a template (e.g. in the “welcome” message, a variable is filled according to the time of day with “Good morning”, “Good afternoon” or “Good evening”). Figure 4 illustrates the whole architecture.
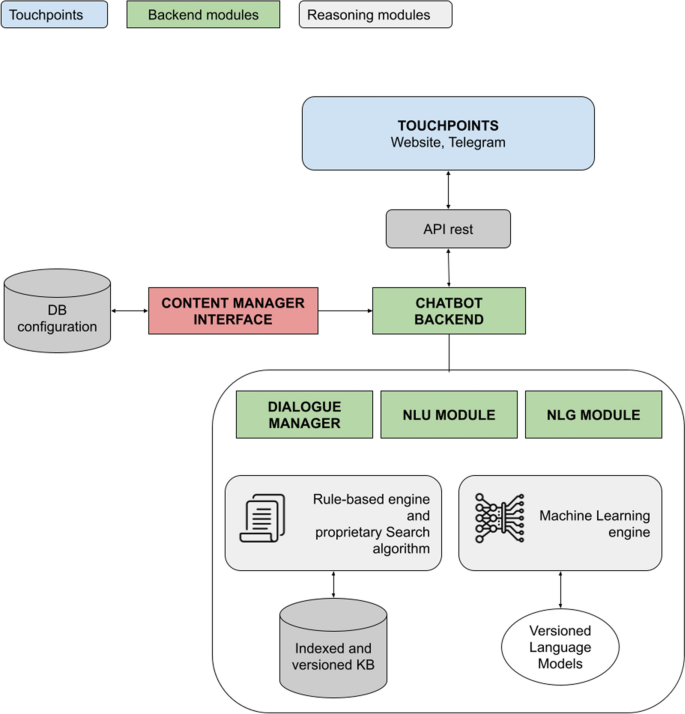
AIDA Chatbot architecture. The figure shows the interlacing between the touch points and the backend modules: the dialogue manager, the NLU and NLG modules, the reasoning ones (AIDA relies on a machine learning system as well as a rule-based engine). The communication with the touch points is established via API rest, while the contents of the agents themselves are managed in a separate database, through a specific content manager graphical interface
The Dialogue Manager is in charge of managing the conversation, making sure that the response is appropriate in the specific context and that the overall exchange is consistent. These three modules constitute the backbone of the chatbot back-end. They exploit both machine learning resources and rule-based approaches in order to build a reasoning system that is robust, but also refinable and explainable [ 62 ]. The following section will also illustrate the interplay between the rule-based engine and the machine learning engine. While the back end constitutes the logic of the application, the content is managed through the Content Manager Interface (CMI). In the CMI, linguists and product owners can insert new questions, modify the chatbot’s answers, enhance its small-talk capabilities, and many other operations to change the system’s content and improve its performance.
The separation of the content from the logic itself allows for flexible management of the experience. Any change in the KB, in the linguistic components, the re-indexing of the content or new language model training can be carried out autonomously, without interacting directly with the codebase.
5.2 AIDA Cookbot’s architecture
The architecture of the voice-based agent integrates different modules, as illustrated in Fig. 5 . First of all, the Amazon Alexa intent recognizer engine matches the user input to one of the intents in the system. The intent recognizer is one of the native functions of Alexa that has to be employed, since it is the module in charge of listening and transcribing the words of the users. Once the sentences have been transcribed, they are matched to one of the intents configured in the Alexa console. AIDA is equipped with two kinds of intents: those that are part of the Recipe interaction model recognize a declaration of allergy or a request for a recipe; those that are part of the FAQ interaction model match user requests to one of the diet theme questions inserted in the agent. Various intents gather the necessary information through the Interaction model: allergens, main ingredient, course, etc. The matched intents and the slots are passed over to the proprietary NLP engine by means of the Lambda function. Footnote 5
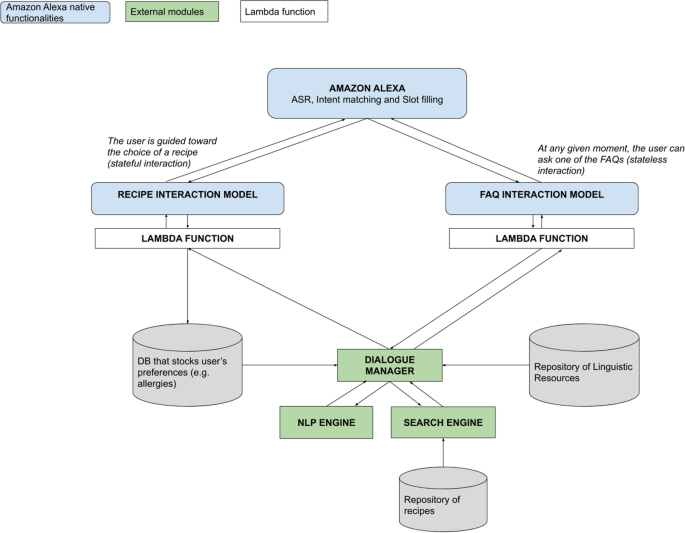
AIDA Cookbot’s architecture. The direction of the arrows symbolizes the flow of the information: from the Alexa endpoint towards one of the two models, down to the understanding engines. Once the proper answer has been crafted, it is passed up again through the up-pointing arrows
The native Lambda function sends the information about the matched intent, as well as eventual slots, to the correct part of the back end. The proprietary back end, composed of the Dialogue Manager and its sub-components, is in charge of directing the whole conversation and retrieving the appropriate content from the repositories. The Dialogue Manager, coupled with the NLP engine, orchestrates the conversation and decides what action is to be taken next in order to keep the dialogue consistent. The NLP engine analyzes the linguistic input it has available (the matched intent and its eventual slots) and extracts meaningful information through its own Named Entity Recognizer module. If the only input is the intent (with or without filled slots), the engine retrieves the answer from the repository of resources and passes it to the previous module. Otherwise, it will pass the information to the Search Engine, which will look for an appropriate recipe in its database, in accordance with the preferences expressed by the user. Once the engine retrieves the correct response, it passes it back to Alexa which will deliver it through its text-to-speech native module.
Similarly to the AIDA Chatbot architecture, all the repositories of linguistic resources or content (list of possible allergens and ingredients, database of recipes) are separated from the codebase. Its logic is agnostic to the resources it exploits, making it easier to update them at any given time.
6 Natural language understanding and dialogue manager modules
This section shows the core algorithms that allow the chatbot to understand patients’ requests, provide the correct answer and manage the dialogue. While for AIDA Chatbot the understanding module is handled autonomously by the authors’ own software, for AIDA Cookbot the intent recognition is regulated solely by Amazon Alexa.
6.1 AIDA Chatbot’s core system
Once the Knowledge Base has been drafted by the scientific board, all the contents are transferred into the CMI in order to be processed. Each question constitutes one of the classes (or label ) that the NLU module will have to predict in order to give the correct answer.
6.1.1 Classification task with BERT
For the machine learning component (ML), each label must be enriched with variations of that question in order to provide the neural model with data. Those variations, also named expansions, are created manually by expert linguists. For a question like Is it okay to eat fried food? , the variations could be I like fried food, can I eat it everyday? or Is it true that fried food is bad for people with diabetes? . The goal is to extend the language model with different examples of how that question could be expressed.
The various examples of a label will be split between train and test. For each question 15 different expansions were generated. This quantity was determined empirically: we observed that for the current amount of questions in the KB (170 units), 15 expansions already accounted for high performances on the test set (0.8 F1 score or higher). This is due to the fact that each example is handcrafted by domain experts. The apparent scarcity of data is therefore compensated by its quality.
6.1.1.1 Model training
We use a bi-directional transformer [ 63 ] that has been pre-trained with the Italian language, known as BERT language model [ 64 ]. BERT produces several models through the various training cycles. If the selected model performs with an accuracy above a threshold of 0.8 in F1 score, it is implemented in the chatbot. Otherwise, it is discarded and the previous version is reinstated. This automated check enables a high quality baseline for the chatbot performances.
The model is trained for 5 epochs with a batch size of 16. These values are kept unmodified during the various training attempts in order to maintain consistency. The results are measured in terms of accuracy. The Appendix document details single labels performances for the model that was released into production.
6.1.1.2 Input processing
Once users write their questions, the sentences are processed through the proprietary NLP pipeline which includes normalization, tokenization, lemmatization, POS tagging, disambiguation and dependency parsing [ 61 ]. This pre-processed data is passed to the language model and it allows for better performance compared to using only the ML component [ 65 ]. BERT has yielded good results for classification tasks in different contexts [ 66 , 67 , 68 ], although those performances are usually obtained by pretraining the model [ 60 , 69 ]. In this context, no specific pretraining was carried out, since the raw performance was already up to 0.8 in terms of accuracy. Such good results are due mainly to the good quality of entry data that was manually created and configured by expert linguists. Our methodology confirms the importance of data quality over quantity for ML applications [ 70 ].
If BERT fails to match the user input to one of the classes with sufficient confidence, a fallback mechanism makes another attempt at understanding the question. This fallback system is based on a proprietary rule-based engine.
6.1.2 Fallback classification with the rule-based engine
The rule-based engine serves multiple purposes: first, it enriches the input with additional information (e.g. specific synonyms of a term) before passing it to the language model. It also checks the user input against a series of patterns, in order to provide a quicker and simpler answer without consulting the neural part of the application. For instance, sentences such as “hello”, “goodbye”, “thank you” do not need to be classified, because they are quite unambiguous and there’s no need to employ a complex system to answer “see you soon”, “you’re welcome”. Moreover, this engine takes care of requests in the form of commands, such as “help” or “menu”. These inquiries directly trigger the Menu of contents, i.e. the list of the chatbot’s themes and sub-themes, without further linguistic analysis.
Secondly, the rule-based engine works as a fallback option in case the language model were to fail. The machine learning module enables a strong and automatized understanding of natural language; nonetheless, neural approaches have their limitations, and lack of explainability is one of them. Even though some work has been done in this direction [ 71 , 72 ], in the setting of an industrial live chatbot the system still needs an explainable backup plan. If BERT is unable to classify the input with sufficient confidence, the chatbot relies on a proprietary algorithm based on Apache Lucene to look for meaningful keywords that were previously configured through the CMI. Footnote 6 The threshold is set at accuracy = 0.4; below that value, the fallback system is activated.
The proprietary algorithm also handles the internal cohesion of the conversation. The dialogue with the chatbot is voluntarily stateless: in a user-driven interaction, the person may ask a different question or change the subject at any time. On the other hand, people naturally employ conversational strategies such as anaphoric references and the machine should be able to interpret even elliptic sentences [ 73 , 74 ]. For this reason, the algorithm also works as a light form of Dialogue Manager, keeping track of the topic that is under discussion. Its topic memorization ability empowers the resolution of these kinds of dialogues:
USER: I was just diagnosed. Does the therapy prescribe mandatory insulin injections? CHATBOT: Injection therapies can be a cause of concern, but it depends on your diagnosis. In type 1 diabetes, therapy is necessarily injective, while in type 2 diabetes there are both injection and oral therapies. USER: And do they hurt? CHATBOT: I understand your concern about the pain of insulin injections - I can reassure you that they are not particularly painful, as small needles (5-6 mm) are used. [...]
In this example, a user employs the anaphoric pronoun they . The chatbot is able to resolve the missing information by remembering the topic from the previous question, i.e. “insulin injections”. Details about the algorithm in charge of the anaphora resolution are described in the work by Bianchini et al. [ 59 ].
6.2 AIDA Cookbot’s interaction model
As has been stated before, the NLU module for the voice-based agent is managed directly by Amazon technology and therefore little work can be done on that by a third party [ 75 ]. On the other hand, the interaction model—i.e. the Skill’s dialogue manager module—has been implemented in-house, in order to have complete control over the conversational path.
AIDA Cookbot’s interaction model relies on a finite state automaton (FSA) structure. Although FSAs have their limitations [ 76 ], it was deemed to be the best strategy in this context where developers have limited control over the NLU part. Given that the input information is already pre-processed by Alexa and therefore somehow implicit, an FSA enables control of the actions of the dialogue system by implementing a stateful interaction. Figure 6 shows the first part of the automaton. When a user enters the Skill, the system checks against its database whether the presence or absence of allergies has already been stored. If the user’s preferences are known, AIDA produces a welcome back message and proceeds to the rest of the dialogue. Otherwise, the Skill asks if the person has any allergies. The user can answer or ask one of the questions related to the diet theme (or produce an out-of-context request; however, this possibility is always true). In any case the system will retrieve the appropriate answer and then go back to the user allergy unknown state.
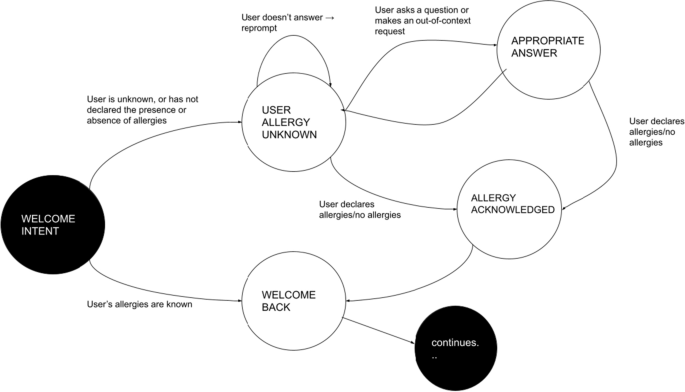
The initial states of AIDA Cookbot’s interaction model
7 Release and usage data
The medical board, the facilitators and the computational linguists began the design process of both AIDAs in February 2020. Shortly after Italy was placed in lockdown due to the COVID-19 situation, the project was strengthened by the increasing needs of patients who could no longer confer with their doctors in person. Both AIDA Chatbot and AIDA Cookbot were made available to the public from July 2020 and officially launched with the communication campaign on October 12, 2020. Between July and October, the systems were refined by incorporating the interaction with medical personnel invited to test the agents. Afterwards, in order to promote the existence of AIDAs, a digital advertising strategy was put in place.
The main objective of this action was to expand the visibility of the Chatbot and the Cookbot to the general public, generate interest and boost traffic to the dedicated landing page. Given the high technological component of the project, we were unsure that users would agree to engage with this tool.
The controlled launch and promotion of the agents also helped to set expectations around the systems [ 77 ]. All editorials stressed the recent nature of the agents, the fact that they were machines (and therefore should not be expected to behave as their doctors) and outlined the contents the CAs were able to deliver.
7.1 AIDA Chatbot usage data and performance
Table 2 presents usage data from the months of November and December 2020. Since some users may interact with the agent during multiple sessions, the number of unique users shall always be lower than the number of sessions. The number of requests coincides with the number of questions posed by the users; however, some of them may be commands (e.g. “menu”) and not natural language sentences. The number of questions posed in natural language is marked in the Assessable requests line. The mean duration of a session is 70 s: even though it is possible to argue that the goal of a chatbot is to engage in dialogue with a person for the longest time possible, AIDA is a task-oriented agent. Users should be able to get the information they are seeking in the shortest possible time; therefore we argue that the data fits the scenario of a user who is looking for specific information and gets it with virtually no latency. The majority of the users come from the website-based AIDA, while a minority access the agent through Telegram. This behavior can be explained with the fact that it is easier or preferable to reach a web page via a link or a plain URL rather than download a specific app.
The most asked subject is Diet , the Diagnosis → General information theme is in second place, while Diagnosis → Symptoms of diabetes is placed third. This user behavior is consistent with the design premises of AIDAs that anticipated the need for two different systems: one written agent with different information spanning the whole spectrum of diabetes, and a voice-based agent focused on the diet theme that provides recipes that comply with diabetes requirements.
7.1.1 Validation
Even though the system is equipped with a fallback system, the rule-based algorithm only activated on 32% of the total number of requests. This means that for most of the questions posed by the users, the BERT-based engine was confident enough to provide the answer. Unfortunately it is not easy to compare our model’s performance with standard baselines [ 78 , 79 ], because the text upon which BERT performs its prediction has already been pre-processed by a specific pipeline that modifies the original input [ 61 ]. In order to shed some light into the actual performances of AIDA Chatbot with real users, we conducted a manual validation task. This task aims to evaluate the performance of the text-based agent through a human-in-the-loop approach [ 80 ].
It is carried out by an annotator with a formal training in linguistics. This person also took part in the co-design phase of the project, so that he was already aware of the content of the system and he was also privy to the internal functioning of the agent. His knowledge was necessary to interpret the quality of the questions and the correctness of the given answer. The annotator can mark any answer given by the chatbot as correct, error, out of context, not applicable or unknown theme. The out of context tag is used when a user makes a request that is beyond the agent’s scope; non applicable is used for commands or other input that is not useful to evaluate; unknown theme marks a question that is within the theme of diabetes, but it is not currently included in the agent’s KB. Unknown theme questions can then be analysed by the scientific board and, if they are deemed appropriate, can be included in the KB along with their answer. When an answer is marked as an error, the annotator can select the correct answer that should have been provided for that request. The system will then integrate the human feedback into its dialogue policy and learn the correct behavior for that occasion. The next time a user asks a similar question, the agent will know the right answer, thanks to the instruction given during the validation session.
The Validation task was performed on a portion of the data for November. Table 3 shows the number of questions that were evaluated together with the evaluation results. The number of correct answers is the highest (42% of the total assessed requests), while the errors amount to 31%. This is due to the fact that while asking the questions, real users may employ linguistic structures that are rather different to the ones drafted by the scientific board. For instance, many users often employed the term “blood glucose” as a synonym of diabetes, even though it is not strictly correct. Other users misspelled certain technical terms such as “glycated hemoglobin”. The confusion and the incorrect writing of medical terms resulted in the match of wrong answers by the system. It is also interesting to note the quantity of unknown theme questions (13%): naturally, users will ask about subjects that were not included in the KB initially. A lot of questions were concerned with the possibility to eat specific food such as pizza, risotto, or polenta (typical Italian cornmeal) that were not taken into account in the construction of the KB. Out of context questions (14%) cover requests that were not pertinent with the scope of the agent: trolling, insults and such.
This analysis serves two purposes: on the one hand, it improves the accuracy of the agents through the human-in the-loop approach. On the other hand, it discovers untouched themes that users find interesting and that can later be incorporated into the system’s knowledge.
7.2 AIDA Cookbot usage data and performances
Table 4 presents usage data from the months of November and December 2020. The lower number of users for the cookbot compared to the chatbot can be explained by the fact that in order to access AIDA Cookbot, people should possess an Amazon Alexa enabled device. Commercial research conducted in 2019 demonstrated that the prevalence of Alexa devices in Italy is still quite low compared to other countries. Footnote 7 It is also true that it is possible to use Alexa’s features from a mobile app, but it is not a widespread phenomenon. The mean duration of the session is higher, as is to be expected from voice-based interaction.
Unfortunately, it is not possible to provide information about users’ requests with the same granularity as AIDA Chatbot. The Alexa Skill console does provide aggregated analytics about the skill’s usage: for instance, intent recognition confidence is estimated at 80% and endpoint latency at less than 292.8 ms. However, without knowing the exact sentences pronounced by the user, it is hard to interpret whether the amount of “NoIntent” matches corresponds to actual “no” requests. Moreover, AIDA Cookbot is mainly structured as a stateful interaction, since its goal is to provide guided recipes based on a series of preferences; nonetheless, users may ask one of the diet related questions at any time, going out of the interaction path momentarily, only to return to it after a while. Analytics may then show a deviation from the mainstream path, but without knowing what was the question asked by the user, it is difficult to tell if it was an out-of-context request, an unknown topic, or simply a question related to the recipe.
8 Discussion
The goal of our work was threefold: to apply typical HCI techniques to a collaborative design of two different CAs, one text-based and one voice-based; to demonstrate the effectiveness of a complex architecture that relies on both machine learning and rule-based engines; and finally, to test the agents in the wild . Specifically, we aimed to investigate whether Italian users would accept such a technology and how would they use it.
With regard to the first objective, our design approach allowed for a quick development of the agents: the workshops gathered all the necessary requirements of the stakeholders and served as constant guidelines during the whole process. By exploiting this methodology, we were constantly checking the progress against the initial specifications, thus ensuring a final product compatible with the needs of patients and clinicians.
The results shown in the previous section demonstrated the success of the second goal. The classification system composed by BERT language model together with a proprietary algorithm was able to classify 91% of the requests with sufficient confidence. We carried out a validation task to gain more insights into the system’s performance: by means of a manual analysis we observed that in the majority of occurrences the agent correctly interpreted users’ requests. In the cases where the CA did not output the right answer, the annotator was able to provide feedback to the agent by indicating the correct label. This human-in-the-loop approach aims to improve the agent’s performance by embedding the annotator’s feedback into the classification task. In the future, we expect to see a reduction in the number of error occurrences thanks to a constant monitoring of the interactions.
Finally, we tackled the third objective by releasing both AIDAs to the general public on widely accessible platforms. Together with the digital advertising campaign, these actions aimed to attract as many users as possible. We believe that the usage data demonstrates the success of these activities: considering the fact that both AIDAs talk in Italian (therefore they have a limited public) and they are dedicated to a subset of the general population (those affected with diabetes), we reached almost 4000 unique users in the span of 2 months. Unfortunately, this kind of data about industrial-based systems in production is usually not available and it is therefore hard to make a comparison with other CAs. Through the analysis of the dialogues we confirmed some expected behavior (e.g. the importance of diet related questions), while others were unforeseen, such as the limited interest in the sport theme. We were also able to gather new questions that are not currently supported but are indeed relevant to the agent’s scope. The 133 occurrences marked as unknown theme constitute excellent suggestions for future expansion of AIDAs’ KB.
9 Conclusion
In this work we presented AIDA, the Artificial Intelligence Diabetes Assistant. AIDA bases its scientific premises on Therapeutic Education, a teaching system that proposes to train patients in order to increase their autonomy and enable them to reach a new level of well-being. The final objective of the project is to offer tools that can guide patient behavior until a balance is obtained between their needs and the constraints imposed by the pathology. In fact, Therapeutic Education is intended to make people with diabetes as conscious and autonomous as possible in the management of their condition [ 10 ]. In order to do so, AIDA was devised as two autonomous but related CAs: AIDA Chatbot and AIDA Cookbot.
AIDA Chatbot is based on written communication and is deployed through a website and via the chat application Telegram. It provides answers to a vast number of questions concerning various aspects of diabetes. AIDA Cookbot, on the other hand, focuses on a specific subject: diet-related questions and advice for people with diabetes. AIDA Cookbot is delivered as an Alexa Skill and therefore expresses itself as voice. It recommends healthy and savory recipes that have been approved by the scientific board. The article reports usage data from the month of November and December 2020. Since the sponsoring campaign started in October, those 2 months represent the most recent and most significant usage data for both agents. AIDAs are, to the best of our knowledge, the sole examples of CAs for diabetes patients that employ the Italian language.
While they were meant primarily for patients, they can be used by caregivers as well. Moreover, physicians may find them useful to help them educate newly diagnosed patients and the general public. For this reason both agents are made publicly available for free, with no restrictions and no technical requirements other than a device with an Internet connection. Different requirements by all the stakeholders were taken into account during the design phase: the subjects of interest for the patients, their preoccupations, and the diabetologists’ point of view.
The aim of this work was to demonstrate the feasibility of two different CAs for TE in the context of diabetes. While the data suggests that the public is willing to interact with those CAs, it would be useful to capture the opinions of users about them. In the future, we plan to conduct a precise evaluation of the systems with a controlled group of patients. It is our intention to investigate what benefits users will experience once they discover and use AIDAs, and whether the dialogues with CAs will have an impact on their clinical progression or psychological well-being.
Data availability
Both systems described in the project are publicly available. The dataset generated and analysed during the current study is not publicly available due to the fact that users may disclose personal information (even though such information is never elicited) to the agents that cannot be divulged. However, once data is accurately anonymized, it could be made available from the corresponding author on reasonable request.
Code availability
The systems described in the work are built upon proprietary software. In some cases, the software relies on publicly available resources (e.g. language models) or it is connected to other endpoints (e.g. Amazon Alexa). These instances are clearly specified in the text. Footnotes or bibliographical references allow readers to further explore the resources.
Alexa Skills are the voice app that can be experienced via Alexa-enabled devices and mobile interface provided by Amazon. For more information: https://developer.amazon.com/en-US/alexa/alexa-skills-kit .
https://www.aidachatbot.it/ .
Handle: @aida_chatbot.
Provided that the specific Alexa device can access Italian content, it is sufficient to say Alexa, apri Aida Diabete in order to access the skill.
https://docs.aws.amazon.com/lambda/latest/dg/welcome.html .
https://lucene.apache.org/ .
https://www.celi.it/blog/2019/08/assistenti-vocali-la-ricerca-di-celi-rivela-le-abitudini-degli-italiani/ .
Pereira J, Diaz O. Using health Chatbots for behavior change: a mapping study. J Med Syst. 2019;43:135. https://doi.org/10.1007/s10916-019-1237-1 .
Article Google Scholar
Vaidyam AN, Wisniewski H, Halamka JD, Kashavan MS, Torous JB. Chatbots and conversational agents in mental health: a review of the psychiatric landscape. Can J Psychiatry. 2019;64(7):456–64. https://doi.org/10.1177/0706743719828977 .
Introduction: standards of medical care in diabetes—2020. Diabetes Care. 2020;43(Supplement 1):S1–2.
Intensive diabetes treatment and cardiovascular disease in patients with type 1 diabetes. N Engl J Med. 2005;353(25):2643– 2653. https://doi.org/10.1056/NEJMoa052187 .
Holman RR, Paul SK, Bethel MA, Matthews DR, Neil HAW. 10-year follow-up of intensive glucose control in type 2 diabetes. N Engl J Med. 2008;359(15):1577–89. http://content.nejm.org/cgi/content/full/359/15/1577 .
Shichiri M, Kishikawa H, Ohkubo Y, Wake N. Long-term results of the Kumamoto Study on optimal diabetes control in type 2 diabetic patients. Diabetes Care. 2000;23(Suppl 2):B21–9.
Google Scholar
Juarez DT, Ma C, Kumasaka A, Shimada R, Davis J. Failure to reach target glycated a1c levels among patients with diabetes who are adherent to their antidiabetic medication. Popul Health Manag. 2014;17(4):218–23.
Black LA, McTear M, Black N, Harper R, Lemon M. Appraisal of a conversational artefact and its utility in remote patient monitoring. In: 18th IEEE symposium on computer-based medical systems (CBMS’05); 2005. p. 506–8.
Klonoff DC, Schwartz DM. An economic analysis of interventions for diabetes. Diabetes Care. 2000;23(3):390–404. https://doi.org/10.2337/diacare.23.3.390 .
Powers MA, Bardsley JK, Cypress M, Funnell MM, Harms D, Hess-Fischl A, et al. Diabetes self-management education and support in adults with type 2 diabetes: a consensus report of the American Diabetes Association, the Association of Diabetes Care & Education Specialists, the Academy of Nutrition and Dietetics, the American Academy. Diabetes Care. 2020;43(7):1636–49. https://care.diabetesjournals.org/content/43/7/1636 .
Vargas-Schaffer G, Cogan J. Patient therapeutic education. Can Fam Physician. 2014;60(3):235–41. https://www.cfp.ca/content/60/3/235 .
Albano MG, Crozet C, d’Ivernois J. Analysis of the 2004–2007 literature on therapeutic patient education in diabetes: results and trends. Acta Diabetol. 2008;45(4):211–9.
Contreras I, Vehi J. Artificial intelligence for diabetes management and decision support: literature review. J Med Internet Res. 2018;20(5):e10775.
Lin J, Li X, Jiang S, Ma X, Yang Y, Zhou Z. Utilizing technology-enabled intervention to improve blood glucose self-management outcome in type 2 diabetic patients initiated on insulin therapy: a retrospective real world study. Int J Endocrinol. 2020. https://doi.org/10.1155/2020/7249782 .
Lester J, Branting K, Mott B. Conversational agents. The practical handbook of internet computing. 2004:220–240.
Moore RJ, Arar R. Conversational UX design: a practitioner’s guide to the natural conversation framework. New York: ACM; 2019.
Book Google Scholar
Riccardi G. Towards healthcare personal agents. In: Proceedings of the 2014 workshop on roadmapping the future of multimodal interaction research including business opportunities and challenges; 2014. p. 53–6.
Maynard D, Hudak P. Small talk, high stakes: interactional disattentiveness in the context of prosocial doctor-patient interaction. Lang Soc. 2008;37:661–8.
Staples S. In: Identifying linguistic features of medical interactions: a register analysis. London: Palgrave Macmillan; 2016.
Miner AS, Laranjo L, Kocaballi AB. Chatbots in the fight against the COVID-19 pandemic. npj Digit Med. 2020;3:65. https://doi.org/10.1038/s41746-020-0280-0 .
Sezgin E, Huang Y, Ramtekkar U, Lin S. Readiness for voice assistants to support healthcare delivery during a health crisis and pandemic. npj Digit Med. 2020;3:122. https://doi.org/10.1038/s41746-020-00332-0 .
McTear M, Callejas Z, Griol D. The conversational interface—talking to smart devices. Berlin: Springer International Publishing; 2016.
Laranjo da Silva L, Dunn AG, Tong HL, Kocaballi AB, Chen J. Conversational agents in healthcare: a systematic review. J Am Med Inform Assoc JAMIA. 2018;25:1248–58.
Shah R, Chircu A. IOT and AI in healthcare: a systematic literature review. Issues Inf Syst. 2018;19(3):33–41.
Liao QV, Wang YC, Bickmore T, Fung P, Grudin J, Yu Z, et al. Human agent communication: connecting research and development in HCI and AI. In: Conference companion publication of the 2019 on computer supported cooperative work and social computing. New York: Association for Computing Machinery; 2019. p. 122–6. https://doi.org/10.1145/3311957.3358607 .
Montenegro JLZ, da Costa CA, da Rosa RR. Survey of conversational agents in health. Expert Syst Appl. 2019;129:56–67.
Schachner T, Keller R, van Wangenheim F. Artificial intelligence-based conversational agents for chronic conditions: systematic literature review. J Med Internet Res. 2020;22(9):e20701. https://doi.org/10.2196/20701 .
Yin K, Laranjo L, Tong HL, Lau AY, Kocaballi AB, Martin P, et al. Context aware systems for chronic disease patients: scoping review. J Med Internet Res. 2019;21(6):e10896. https://doi.org/10.2196/10896 .
Rehman UU, Chang DJ, Jung Y, Akhtar U, Razzaq MA, Lee S. Medical instructed real-time assistant for patient with glaucoma and diabetic conditions. Appl Sci. 2020;10:2216.
Bukhsh A, Goh BH, Zimbudzi E, Lo C, Zoungas S, Chan KG, et al. Type 2 diabetes patients’ perspectives, experiences, and barriers toward diabetes-related self-care: a qualitative study from Pakistan. Front Endocrinol. 2020;11:711. https://doi.org/10.3389/fendo.2020.534873 .
Harvey J, Dopson S, McManus RJ, Powell J. Factors influencing the adoption of self-management solutions: an interpretive synthesis of the literature on stakeholder experiences. Implement Sci. 2015;10(159):103–10. https://doi.org/10.1186/s13012-015-0350-x .
Laranjo L, Neves AL, Costa A, Ribeiro RT, Couto L, Sà AB. Facilitators, barriers and expectations in the self-management of type 2 diabetes—a qualitative study from Portugal. Eur J Gen Pract. 2015;21(2):103–10. https://doi.org/10.3109/13814788.2014.1000855 .
Xie H, Xie W, Ding R, Yan J, Qu Y. A mobile-based question-answering and early warning system for assisting diabetes management. Wirel Commun Mob Comput. 2018. https://doi.org/10.1155/2018/9163160 .
Frøisland DH, Årsan E. Integrating visual dietary documentation in mobile-phone-based self-management application for adolescents with type 1 diabetes. J Diabetes Sci Technol. 2015;9(3):541–8. https://doi.org/10.1177/1932296815576956 .
Wang TH, Zhou XF, Ni Y, Pan ZG. Health information needs regarding diabetes mellitus in China: an internet-based analysis. BMC Public Health. 2020;20:990. https://doi.org/10.1186/s12889-020-09132-3 .
Elsabrout K. Increasing diabetic patient engagement and self-reported medication adherence using a web-based multimedia program. J Am Assoc Nurse Pract. 2018;30(5):293–8. https://doi.org/10.1097/JXX.0000000000000045 .
Sanchez Bocanegra CL, Sevillano Ramos JL, Rizo C, Civit A, Fernandez Luque L. HealthRecSys: a semantic content-based recommender system to complement health videos. BMC Med Inform Decis Mak. 2017;17(63):1–10. https://doi.org/10.1186/s12911-017-0431-7 .
Stephens TN, Joerin A, Rauws M, Werk LN. Feasibility of pediatric obesity and prediabetes treatment support through Tess, the AI behavioral coaching chatbot. Transl Behav Med. 2019;9(3):440–7. https://doi.org/10.1093/tbm/ibz043 .
Freyne J, Berkovsky S. Intelligent food planning: personalized recipe recommendation. In: Proceedings of the 15th international conference on Intelligent user interfaces; 2010. p. 321–4.
Pecune F, Callebert L, Marsella S. A Socially-aware conversational recommender system for personalized recipe recommendations. In: Proceedings of the 8th international conference on human-agent interaction. New York: Association for Computing Machinery; 2020. p. 78–86. https://doi.org/10.1145/3406499.3415079 .
Calle Bustos AM, Juan MC, Garcia Garcia I, Abad F. An augmented reality game to support therapeutic education for children with diabetes. PLoS ONE. 2017;12(9):1–23. https://doi.org/10.1371/journal.pone.0184645 .
Trouilloud D, Regnier J. Therapeutic education among adults with type 2 diabetes: effects of a three-day intervention on perceived competence, self management behaviours and glycaemic control. Glob Health Promot. 2013;20(2 suppl):94–8. https://doi.org/10.1177/1757975913483331 .
Angara P, Jiménez M, Agarwal K, Jain H, Jain R, Stege U, et al. Foodie fooderson: a conversational agent for the smart kitchen. In: Proceedings of the 27th annual international conference on computer science and software engineering. CASCON ’17. USA: IBM Corp.; 2017. p. 247–53.
Crangle CE, Bradley C, Carlin PF, Esterhay RJ, Harper R, Kearney PM, et al. Exploring patient information needs in type 2 diabetes: a cross sectional study of questions. PLoS ONE. 2018;13(11):1–19. https://doi.org/10.1371/journal.pone.0203429 .
Guo X, Liang L, Liu Y, Weng H, Hao T. The construction of a diabetes oriented frequently asked question corpus for automated question answering services. In: Proceedings of the 2020 conference on artificial intelligence and healthcare. CAIH2020. New York: Association for Computing Machinery; 2020. p. 60–6. https://doi.org/10.1145/3433996.3434008 .
Ferguson G. English for medical purposes. In: Paltridge B, Starfield S, editors. The handbook of english for specific purposes. Chichester: Wiley; 2013. p. 243–61.
Hull M. Medical language proficiency: a discussion of interprofessional language competencies and potential for patient risk. Int J Nurs Stud. 2016;54:158–72.
Maharjan B, Li J, Kong J, Tao C. Alexa, What Should I Eat?: a personalized virtual nutrition coach for native American diabetes patients using Amazon’s Smart Speaker Technology. In: 2019 IEEE international conference on E-health networking, application services (HealthCom); 2019. p. 1–6.
Chen ML, Wang HC. How personal experience and technical knowledge affect using conversational agents. In: Proceedings of the 23rd international conference on intelligent user interfaces companion. IUI ’18 Companion. New York: Association for Computing Machinery; 2018. https://doi.org/10.1145/3180308.3180362 .
Grimes GM, Schuetzler RM, Giboney JS. Mental models and expectation violations in conversational AI interactions. Decis Support Syst. 2021;144:113515.
Luger E, Sellen A. “Like Having a Really Bad PA”: the gulf between user expectation and experience of conversational agents. In: Proceedings of the 2016 CHI conference on human factors in computing systems. CHI ’16. New York: Association for Computing Machinery; 2016. p. 5286–97. https://doi.org/10.1145/2858036.2858288 .
O’Leary TK, Stowell E, Kimani E, Parmar D, Olafsson S, Hoffman J, et al. Community-based cultural tailoring of virtual agents. In: Proceedings of the 20th ACM international conference on intelligent virtual agents. New York: Association for Computing Machinery; 2020. https://doi.org/10.1145/3383652.3423875 .
Benítez-Guijarro A, Bond R, Booth F, Callejas Z, Ennis E, Esposito A, et al. In: D'Haro LF, Callejas Z, Nakamura S, editors. Co-creating requirements and assessing end-user acceptability of a voice-based chatbot to support mental health: a thematic analysis of a living lab workshop. Singapore: Springer; 2021. p. 201–12. https://doi.org/10.1007/978-981-15-8395-715 .
Radziwill NM, Benton MC. Evaluating quality of Chatbots and intelligent conversational agents; 2017. arXiv:1704.04579 [cs.CY].
Sweeney C, Potts C, Ennis E, Bond R, Mulvenna MD, O’Neill S, et al. Can Chatbots help support a person’s mental health? Perceptions and views from mental healthcare professionals and experts. ACM Trans Comput Healthc. 2021;2(3):1–15. https://doi.org/10.1145/3453175 .
Bickmore TW, Pfeifer LM, Jack BW. Taking the time to care: empowering low health literacy hospital patients with virtual nurse agents. New York: Association for Computing Machinery; 2009. p. 1265–74. https://doi.org/10.1145/1518701.1518891 .
Bickmore TW, Trinh H, Olafsson S, Oeary TK, Asadi R, Rickles NM, et al. Patient and consumer safety risks when using conversational assistants for medical information: an observational study of Siri, Alexa, and Google Assistant. J Med Internet Res. 2018;20(9):e11510. https://doi.org/10.2196/11510 .
Fukuoka Y, Lindgren TG, Bonnet K, Kamitani E. Perception and sense of control over eating behaviors among a diverse sample of adults at risk for type 2 diabetes. Diabetes Educ. 2014;40(3):308–18. https://doi.org/10.1177/0145721714522717 .
Gimme the usual—how handling of pragmatics improves Chatbots. In: Proceedings of the fourth Italian conference on computational linguistics (CLiC-it 2017). Rome: CEUR-WS; 2017. http://ceur-ws.org/Vol-2006/paper035.pdf .
Alloatti F, Di Caro L, Sportelli G. Real life application of a question answering system using BERT language model. In: Proceedings of the 20th annual SIGdial meeting on discourse and dialogue. Stockholm: Association for Computational Linguistics; 2019. p. 250–3. https://www.aclweb.org/anthology/W19-5930 .
Tarasconi F, Di Tomaso V. Geometric and statistical analysis of emotions and topics in corpora. IJCol. 2015;1(1). http://journals.openedition.org/ijcol/3232 .
Liao QV, Singh M, Zhang Y, Bellamy RKE. Introduction to explainable AI. New York: Association for Computing Machinery; 2020. p. 1–4. https://doi.org/10.1145/3334480.3375044 .
Vaswani A, Shazeer N, Parmar N, Uszkoreit J, Jones L, Gomez AN, et al. Attention is all you need; 2017. arXiv:1706.03762 [cs.CL].
Devlin J, Chang MW, Lee K, Toutanova K. BERT: pre-training of deep bidirectional transformers for language understanding. arXiv preprint. 2018; arXiv:1810.04805v1 .
Suta P, Mongkolnam P, Chan J, Lan X, Wu B. An overview of machine learning in Chatbots. Int J Mech Eng Robot Res. 2020;9:502–10. https://doi.org/10.18178/ijmerr.9.4.502-510 .
Adhikari A, Ram A, Tang R, Lin J. DocBERT: BERT for document classification; 2019. arXiv:1904.08398 [cs.CL].
Lee JS, Hsiang J. PatentBERT: patent classification with fine-tuning a pre-trained BERT Model; 2019. arXiv:1906.02124 [cs.CL].
Nikolov A, Radivchev V. Nikolov-Radivchev at SemEval-2019 Task 6: offensive tweet classification with BERT and ensembles. In: Proceedings of the 13th international workshop on semantic evaluation; 2019. p. 691–5.
Sun C, Qiu X, Xu Y, Huang X. How to fine-tune bert for text classification? In: China national conference on Chinese computational linguistics. Springer; 2019. p. 194–206.
Jain A, Patel H, Nagalapatti L, Gupta N, Mehta S, Guttula S, et al. Overview and importance of data quality for machine learning tasks. In: Proceedings of the 26th ACM SIGKDD international conference on knowledge discovery and data mining. KDD ’20. New York: Association for Computing Machinery; 2020. p. 3561–2. https://doi.org/10.1145/3394486.3406477 .
Aken BV, Winter B, Loser A, Gers FA. VisBERT: hidden-state visualizations for transformers. In: Companion proceedings of the web conference 2020. WWW ’20. New York: Association for Computing Machinery; 2020. p. 207–11. https://doi.org/10.1145/3366424.3383542 .
van Aken B, Winter B, Loser A, Gers FA. How does BERT answer questions? A layer-wise analysis of transformer representations. In: Proceedings of the 28th ACM international conference on information and knowledge management. CIKM ’19. New York: Association for Computing Machinery; 2019. p. 1823–32. https://doi.org/10.1145/3357384.3358028 .
Gandrabur S, Buist E, Curin J, Kleindienst J, Havelka J. Anaphora resolution using linguistic cues, dialogue context, and general knowledge. Google Patents; 2015. US Patent 9,171,542.
Skantze G. Error handling in spoken dialogue systems-managing uncertainty, grounding and miscommunication. Stockholm: Gabriel Skantze; 2007.
Ashktorab Z, Jain M, Liao QV, Weisz JD. Resilient Chatbots: repair strategy preferences for conversational breakdowns. New York: Association for Computing Machinery; 2019. p. 1–12. https://doi.org/10.1145/3290605.3300484 .
Larsson S, Traum DR. Information state and dialogue management in the TRINDI dialogue move engine toolkit. Nat Lang Eng. 2000;6(3–4):323–40. https://doi.org/10.1017/S1351324900002539 .
Kocielnik R, Amershi S, Bennett PN. Will you accept an imperfect AI? Exploring designs for adjusting end-user expectations of AI systems. In: Proceedings of the 2019 CHI conference on human factors in computing systems. New York: Association for Computing Machinery; 2019. p. 1–14. https://doi.org/10.1145/3290605.3300641 .
Alberti C, Lee K, Collins M. A BERT baseline for the natural questions. CoRR. 2019. arXiv:1901.08634 .
Wang S, Manning CD. Baselines and bigrams: simple, good sentiment and topic classification. In: Proceedings of the 50th annual meeting of the association for computational linguistics: short papers—volume 2. ACL ’12. USA: Association for Computational Linguistics; 2012. p. 90–4.
Li J, Miller AH, Chopra S, Ranzato M, Weston J. Dialogue learning with human-in-the-loop; 2017. arXiv:1611.09823 [cs.AI].
Download references
The AIDA project was funded by Novo Nordisk Spa in its entirety (design workshops, development and promotion).
Author information
Authors and affiliations.
H-FARM Innovation, Turin, Italy
Francesca Alloatti & Alessio Bosca
Department of Computer Science, University of Turin, Turin, Italy
Francesca Alloatti & Luigi Di Caro
Novo Nordisk Spa, Rome, Italy
Fabrizio Pieraccini
You can also search for this author in PubMed Google Scholar
Contributions
All authors contributed to the work. Specifically, FP made substantial contributions to the conception or design of the work. FA and AB worked on the acquisition, analysis, and interpretation of data as well as the creation of the software described in the article. FA also revised the manuscript, integrating the comments by the reviewers. LDC revised the manuscript to assure scientific accuracy and quality. All authors read and approved the final manuscript.
Corresponding author
Correspondence to Francesca Alloatti .
Ethics declarations
Consent to participate.
Informed consent was obtained from the people that took part in the design workshops (Sect. 3 ). With regard to the usage data, since the study is observational in nature, the University of Turin has confirmed that no ethical approval is required.
Competing interests
One of the authors, Fabrizio Pieraccini, is employed in Novo Nordisk Spa and had a direct role in the creation and promotion of the AIDA project. Francesca Alloatti and Alessio Bosca are part of H-FARM Innovation, the company that developed the agents. Francesca Alloatti is also affiliated to the University of Turin, as is Luigi Di Caro. The University has no interest to declare.
Additional information
Publisher's note.
Springer Nature remains neutral with regard to jurisdictional claims in published maps and institutional affiliations.
Supplementary Information
Below is the link to the electronic supplementary material.
Supplementary file1 (DOCX 15 KB)
Rights and permissions.
Open Access This article is licensed under a Creative Commons Attribution 4.0 International License, which permits use, sharing, adaptation, distribution and reproduction in any medium or format, as long as you give appropriate credit to the original author(s) and the source, provide a link to the Creative Commons licence, and indicate if changes were made. The images or other third party material in this article are included in the article's Creative Commons licence, unless indicated otherwise in a credit line to the material. If material is not included in the article's Creative Commons licence and your intended use is not permitted by statutory regulation or exceeds the permitted use, you will need to obtain permission directly from the copyright holder. To view a copy of this licence, visit http://creativecommons.org/licenses/by/4.0/ .
Reprints and permissions
About this article
Alloatti, F., Bosca, A., Di Caro, L. et al. Diabetes and conversational agents: the AIDA project case study. Discov Artif Intell 1 , 4 (2021). https://doi.org/10.1007/s44163-021-00005-1
Download citation
Received : 04 May 2021
Accepted : 02 August 2021
Published : 22 September 2021
DOI : https://doi.org/10.1007/s44163-021-00005-1
Share this article
Anyone you share the following link with will be able to read this content:
Sorry, a shareable link is not currently available for this article.
Provided by the Springer Nature SharedIt content-sharing initiative
- Find a journal
- Publish with us
- Track your research

An official website of the United States government
The .gov means it’s official. Federal government websites often end in .gov or .mil. Before sharing sensitive information, make sure you’re on a federal government site.
The site is secure. The https:// ensures that you are connecting to the official website and that any information you provide is encrypted and transmitted securely.
- Publications
- Account settings
The PMC website is updating on October 15, 2024. Learn More or Try it out now .
- Advanced Search
- Journal List
- J Family Med Prim Care
- v.8(1); 2019 Jan

Impact of health education on knowledge, attitude, practices and glycemic control in type 2 diabetes mellitus
Sumit pal singh chawla.
1 Department of Medicine, Guru Gobind Singh Medical College and Hospital, Faridkot, Punjab, India
Sarabjot Kaur
Aman bharti, ravinder garg, manjeet kaur, amrita ghosh.
2 Department of Biochemistry, Calcutta Medical College, Kolkata, West Bengal, India
Ranabir Pal
3 Department of Community Medicine, MGM Medical College and LSK Hospital, Kishanganj, Bihar, India
Background:
Effective health education consequently improves knowledge, attitude, and practices (KAP) leading to better glycemic control and is widely accepted as an integral part of comprehensive diabetes care for affected individuals and their families in primary care settings.
To assess the impact of health education on knowledge, attitude, practices, and glycemic control in type 2 diabetes mellitus patients.
Settings and Design:
This case control study was conducted in the department of Medicine of a tertiary care teaching hospital.
The study was conducted on 100 diabetic subjects aged more than 40 years comprising of 50 cases and 50 controls. Cases were given education on their disease, drugs, dietary, and lifestyle modifications along with patient education leaflet at baseline and at first follow-up, while controls received neither of these. Subjects were assessed for KAP by administering KAP questionnaire and for glycemic control by measuring glycated hemoglobin (HbA1C) at baseline and at the end of the study.
In this study, end mean knowledge, attitude, practice, and KAP SUM scores of cases (10.28 ± 1.78, 3.46 ± 0.93, 3.14 ± 0.86, and 16.82 ± 3.40, respectively) showed significant increase from the baseline (3.86 ± 0.93, 1.00 ± 0.83, 0.40 ± 0.64, and 5.26 ± 2.10, respectively) compared to controls, accompanied by significant reduction in HbA1C of cases at the end of the study compared to the controls.
Conclusions:
Effective health education improves knowledge, attitude, and practices, particularly with regard to lifestyle modifications and dietary management, culminating into better glycemic control that can slow down the progression of diabetes and prevent downstream complications.
Introduction
Diabetes mellitus (DM) leads to alarming clinical, social, financial, and public health issues with devastating long-term effects on the well-being, affecting quality of life including neuropathy, retinopathy, nephropathy, dementia, and cognitive problems.[ 1 , 2 , 3 ] Self-monitoring of blood sugar and apt self-care with effective metabolic regulation affect hypoglycemia, ketoacidosis, or microvascular and macrovascular complications.[ 3 ] DM prevalence has risen dramatically over the past 2 decades, with India being the major contributor. Global burden in 2011 was 8.3% (366.2 millions) compared to 4% in 1995 and is projected to rise to 551.9 million by 2030.[ 4 , 5 ] However, our National Health Policy 2017 envisaged reducing premature mortality from diabetes and other non-communicable diseases to 25% by 2025.[ 6 ] There is a need to focus on preventive efforts with research on optimum choice of user-friendly test/s in the first contact care. Although glycated hemoglobin (HbA1C) does not require fasting and may be the best compromise, diagnosis and prognosis cannot be solely relied on HbA1C values that are affected in presence of different physiological and co-morbid conditions.[ 7 , 8 , 9 , 10 ] Diabetes education is considered an essential tool as its management largely depends on knowledge, motivation, and ability to pursue self-care in activities of daily living. Therefore, counseling and health education should be given paramount importance by the physicians even in their busy schedule including elaborate advice on lifestyle modifications and diet.[ 10 , 11 , 12 , 13 ] Although, evidences suggest that diabetics with more knowledge and motivated self-care help to achieve better glycemic control, there is difference of opinion on effectiveness of methods of health education and educational efforts to improve interventions are key components of effective treatment plan for DM.[ 14 , 15 , 16 , 17 ]
Health education is essential in resource poor settings such as ours, wherein DM pose great financial burden and calls for urgent participation of clinicians at all levels especially primary care physicians as they are usually the first care givers and come across known as well as recently detected diabetics. These patients can be imparted health education by primary care physicians to adopt healthy lifestyle practices, remain motivated for regular testing of glycemic status, and be aware of diabetic complications. The consequent changes in knowledge, attitude, and practices of diabetic patients are vital for achieving glycemic control and for preventing the development of complication of diabetes.
This study was undertaken to assess the positive impact of health education on knowledge attitude, and practices with effectively improved patient glycemic control in type 2 DM.
This case-control study, conducted at the department of Medicine of a tertiary care teaching hospital in north-west India, enrolled 100 diabetics randomized into 50 cases and 50 controls.
Inclusion criteria: Both males and females aged more than 40 years having type 2 DM as per the American Diabetes Association ADA criteria and normal cognition were included in this study.[ 18 ]
Exclusion criteria: Patients aged less than 40 years, pregnant women, those having type 1 DM, mental retardation, psychotic disorders, or cognitive decline due to any cause and non-consenters were excluded.
Data collection procedure: The study conformed to the Helsinki Declaration. Approval was sought from the institutional ethics committee. All the participants were explained about the purpose of the study and were ensured strict confidentiality, and that it would be used only for academic purpose. Then, informed consent was taken from each of them before the total procedure. The participants were given the options not to participate in the study if they wanted. All the participants underwent detailed history, clinical examination, and necessary laboratory investigations; baseline levels of knowledge, attitude, and practices regarding DM were assessed. The case group participants received health education on their disease, drugs, dietary, and lifestyle modifications along with the “patient education leaflet” to compliment the verbal counseling at baseline; same was not provided to the control group. After baseline, two follow-ups were made at an interval of 2 months each. At baseline and at each follow-up visit, random plasma glucose (RPG) was measured in all the subjects (cases and controls). HbA1C was measured at baseline and final follow-up. A pre-designed and validated KAP questionnaire [ Table 1 ] was administered at baseline and at final follow-up to assess awareness regarding DM and its management. The questionnaire, in English or regional language was filled in a face-to-face interview, contained questions relating to the socio-demographic information of the participants. The pilot study was carried out among comparable population, following which some of the questions from the interview schedule were modified. Patients taken for the study were treated as per standard treatment guidelines. Patients requiring major treatment modifications during follow-up period were dropped out. Random venous blood samples were taken and were examined in the biochemistry laboratory of the institute.
Knowledge, attitude, and practices questionnaire
Yes/no | Score | |
---|---|---|
Knowledge questions | ||
Diabetes is a condition in which the body contains higher level of blood sugar than normal | ||
The major causes of diabetes are hereditary, obesity, and unhealthy eating habits | ||
The symptoms of diabetes are frequent urination, increased thirst, and hunger | ||
Diabetes, if not treated, will cause damage to other organs such as heart, eyes, kidneys, and foot ulcers | ||
The most accurate method of monitoring diabetes is checking blood glucose levels | ||
In a diabetic patient, high blood pressure can worsen the risk of heart attack, stroke, kidney, and eye problems | ||
A diabetic patient should measure his or her blood pressure regularly | ||
The lifestyle modification required for diabetic patients is weight reduction, stopping alcohol consumption, and smoking | ||
A diabetic patient should have his or her eyes checked every year | ||
The important factors that help in controlling blood sugar are controlled and planned diet, and medication | ||
Regular urine tests will help in knowing the amount of proteins in your urine and functional status of kidneys | ||
A regular exercise regimen will help in blood glucose control | ||
A well-balanced diet include carbohydrates, proteins, fruits, and fibres | ||
Diabetes is a curable/non-curable disease | ||
Attitude questions | ||
Do you exercise regularly? | ||
Are you following a controlled and planned diet? | ||
Do you think missing doses of your diabetic medication will have a negative effect on your disease control? | ||
Are you aware of your blood sugar levels fall below normal when you are taking drugs? | ||
Do you think you should keep in touch with your physician? | ||
Practice questions | ||
When your blood pressure was last checked? | ||
When was your last eye examination? | ||
When was your last urine examination? | ||
When was your last visit to your physician? | ||
When was your blood sugars last checked? | ||
When was your lipids last checked? | ||
Total |
Data analysis: The results were analyzed statistically to assess the impact of health education on knowledge, attitude, practices and glycemic control in diabetic patients. The data collected were entered into Excel spread sheets, and analysis was carried out using IBM SPSS Statistics for Windows, Version 11.0. Armonk, NY SPSS software. The pre-tested closed ended questionnaire was used to assess the impact of health education intervention, where a score of each answer was coded as Yes = 1, No = 0. Mean were used to derive information on knowledge, attitude, and practice aspect of diabetic patients. Paired t test was applied to the pre-test and post-test results of knowledge, attitude, and practice on diabetes to assess the impact of health education intervention, P value <0.05 was considered statistically significant.
Mean age of the cases was 55.50 ± 9.37 years and of controls was 52.02 ± 7.83 years; 22 (44%) males were among cases and 25 (50%) among controls. Among cases, 26 (52%) were literate; in the control group, 30 (60%) were literate. Among cases, 16 (32%) were recently detected to have diabetes, while 34 (68%) were having diabetes for more than 6 months. In the control group, 20 (40%) were recently detected to have diabetes, while 30 (60%) were having diabetes for more than 6 months. In the case group, 27 (54%) were overweight and 23 (46%) were obese; in the control group 32 (64%) were overweight and 18 (36%) were obese. RPG was checked in all the participants at baseline and at each follow-up, showing significant declining trend of RPG in the case group from baseline to first follow-up and subsequently to final follow-up. Similar trend was not observed in the control group [ Table 2 ].
Random plasma glucose-cases and controls
RPG | Baseline | First follow-up | Final follow-up | |||
---|---|---|---|---|---|---|
Case group | Control group | Case group | Control group | Case group | Control group | |
≤200 | 0 | 0 | 0 | 0 | 0 | 0 |
201-210 | 0 | 0 | 0 | 0 | 0 | 0 |
211-220 | 0 | 0 | 0 | 0 | 7 | 0 |
221-230 | 0 | 0 | 0 | 0 | 20 | 0 |
231-240 | 0 | 0 | 4 | 0 | 13 | 0 |
241-250 | 0 | 0 | 1 | 0 | 0 | 0 |
251-260 | 0 | 0 | 11 | 0 | 0 | 0 |
261-270 | 0 | 0 | 15 | 0 | 0 | 0 |
271-280 | 0 | 0 | 8 | 0 | 6 | 0 |
281-290 | 0 | 0 | 1 | 0 | 4 | 0 |
291-300 | 0 | 0 | 5 | 0 | 0 | 1 |
301-310 | 4 | 7 | 3 | 8 | 0 | 6 |
311-320 | 0 | 6 | 2 | 5 | 0 | 10 |
321-330 | 0 | 2 | 0 | 7 | 0 | 7 |
331-340 | 9 | 9 | 0 | 8 | 0 | 6 |
341-350 | 7 | 10 | 0 | 6 | 0 | 5 |
351-360 | 9 | 0 | 0 | 1 | 0 | 2 |
361-370 | 3 | 5 | 0 | 4 | 0 | 6 |
371-380 | 3 | 4 | 0 | 4 | 0 | 0 |
381-390 | 4 | 0 | 0 | 0 | 0 | 1 |
391-400 | 5 | 2 | 0 | 3 | 0 | 3 |
401-410 | 4 | 4 | 0 | 3 | 0 | 3 |
411-420 | 2 | 1 | 0 | 1 | 0 | 0 |
421-430 | 0 | 0 | 0 | 0 | 0 | 0 |
431-440 | 0 | 0 | 0 | 0 | 0 | 0 |
441-450 | 0 | 0 | 0 | 0 | 0 | 0 |
≥451 | 0 | 0 | 0 | 0 | 0 | 0 |
RPG: Random plasma glucose
At baseline, the case group patients had mean knowledge score of 3.86 ± 0.93, and at final follow-up, this score was 10.28 ± 1.78. In the control group, the mean knowledge score was 5.66 ± 1.61 at baseline, and at final follow-up, it was 5.76 ± 1.72. There was a significant increase in mean knowledge score of cases compared to controls at the final follow-up ( P = 0.004) [ Figure 1 ].

Mean knowledge scores of cases and controls at baseline and at final follow-up
Mean attitude score of the cases at baseline was 1.00 ± 0.83, and at final follow-up, the score rose to 3.46 ± 0.93. The control group had mean attitude scores of 1.78 ± 0.97 at baseline and 1.79 ± 0.48 at final follow-up. The increase in the mean attitude score of cases was statistically significant as compared to the controls ( P = 0.003) [ Figure 2 ].

Mean attitude scores of cases and controls at baseline and at final follow-up
The cases had mean practice score of 0.40 ± 0.64 at baseline, and at final follow-up, this score was 3.14 ± 0.86. In the control group, at baseline, the mean practice score was 1.54 ± 0.73, and at final follow-up, it was 1.58 ± 0.86. There was significant increase in the mean practice score of cases as compared to controls at the final follow-up ( P = 0.001) [ Figure 3 ].

Mean practice scores of cases and controls at baseline and at final follow-up
At baseline, cases had mean KAP SUM score 5.26 ± 2.10, and at final follow-up, this score increased to 16.82 ± 3.40. In the control group, the mean KAP SUM score was 8.98 ± 3.24 at baseline and 9.08 ± 3.36 at final follow-up. Increase in the mean KAP SUM score of cases was statistically significant compared to controls at the final follow-up ( P = 0.003) [ Figure 4 ].

Mean knowledge, attitude, and practice sum scores of cases and controls at baseline and at final follow-up
HbA1c was measured in all the participants at baseline and at final follow-up. At baseline, 22 (44%) cases had HbA1c in the range of 7.1–8%, 17 (34%) had HbA1c between 8.1–9%, and remaining 11 (22%) had HbA1 c between 9.1–10%. At final follow-up, 30 (60%) had HbA1c 7%, 11 (22%) had HbA1c in the range of 7.1–8%, and remaining 9 (18%) had HbA1C between 8.1–9%. The reduction in HbA1C of case group patients from baseline to final follow-up was statistically significant ( P = 0.01). Among controls, at baseline, 13 (26%) had HbA1C in the range of 7.1–8%, 30 (60%) had HbA1C between 8.1–9%, and remaining 7 (14%) had HbA1C between 9.1–10%. At the final follow-up, 13 (26%) were having HbA1C in the range of 7.1–8%, 30 (60%) had HbA1C ranging between 8.1–9%, and remaining 7 (14%) had HbA1C between 9.1–10%. Thus, there was no reduction in HbA1C of controls at the final follow-up ( P = 0.159) [ Figure 5 ].

HbA1C of cases at baseline and at final follow-up
Health education imparts knowledge on environmental, physical, social, emotional, intellectual, and spiritual health. Sincere health education is able to hone knowledge, attitude, and practices of individuals and can lead to better control of diabetes. It is widely accepted as an integral part of comprehensive diabetes care especially in resource poor settings wherein diabetes poses a great financial burden. The complications of DM have an adverse impact on the quality of life of patients and their families, with a devastating long-term effect on their financial and social well-being. Various studies performed so far suggest positive outcome of health education in terms of slowing the progression of disease and preventing complications.
In this study, a total of 100 diabetics were allocated randomly into 2 groups, 50 cases, and 50 controls (age and sex-matched); 54% were overweight and 46% obese among cases, and in the control group, 64% were overweight and 36% were obese. In a similar study conducted by Fatema et al . in Bangladesh, the mean BMI of subjects was 24.4 ± 4.1 kg/m 2 .[ 19 ]
RPG was checked in all participants at baseline and at each follow-up which showed a declining trend in the case group from baseline to first follow-up and subsequently to final follow-up, which was not observed in the control group. This highlighted the relevance of health education in bringing glycemic control in diabetic patients. Similar results were seen in the study by Shareef et al . who noted a significant decrease in the fasting and post prandial blood glucose levels from baseline on the final follow-up in the intervention group.[ 20 ]
In this study, mean knowledge, attitude, practice, and KAP SUM scores of cases measured at the end of the study showed significant increase from the baseline compared to the controls. Thus, imparting health education led to significant improvement in all 3 spheres of diabetes management i.e., knowledge, attitude, and practice. The study conducted by Shareef et al . also found significant increase in overall diabetes knowledge from 18.05% to 25.43% in the intervention group compared to controls. The mean scores of patient's knowledge about diabetes, self-care practices, and knowledge regarding complications of diabetes showed significant improvement in the intervention group patients after they were imparted health education. A significant decrease in the fasting and post prandial blood glucose levels was also observed at final follow-up from the baseline period in the intervention group.[ 21 ] Ghazanfari Z. et al . also observed a significant improvement in the knowledge ( P < 0.001), attitudes, and practices of the intervention group toward healthy behaviors regarding nutrition, physical activity, and self-care.[ 22 ]
In another study similar to ours, the mean knowledge, attitude, and practice scores at baseline were 6.56 ± 4.06, 2.24 ± 1.65, and 1.74 ± 1.26 in the control group, respectively and 6.48 ± 4.49, 2.18 ± 1.36, and 3.1 ± 1.5 in the test group, respectively. Mean KAP scores were 10.46 ± 6.19 and 11.96 ± 6.84 in the control and test groups, respectively at baseline. At final follow-up, the mean knowledge scores were 7.12 ± 3.98 and 12.12 ± 2.12, mean attitude scores were 2.24 ± 1.33 and 4.12 ± 0.87, mean practice scores were 1.92 ± 1.34 and 4.46 ± 1.01, and the mean KAP scores were 11.36 ± 6.12 and 20.78 ± 3.13 in the control and test groups, respectively. Statistically, there was significant difference in knowledge, attitude, practice, and total KAP scores between the test and the control groups at final follow-up.[ 23 ]
HbA1C was measured at baseline and at final follow-up; statistically significant reduction in HbA1C was in the cases at final follow-up as compared to baseline HbA1C values. Similar reduction in HbA1C was not observed in the control group. This emphasized the importance of health education in attaining long-term glycemic control in diabetes and preventing its complications. Similar findings were reported in the study by Ahmed et al . wherein the mean levels of FBS and HbA1C had significantly decreased at the second visit compared to the first visit (180.33 ± 34.81; 8.69 ± 0.72 versus 168.04 ± 28.56, 7.50 ± 0.97, respectively). In addition, there was a strong negative correlation between the knowledge attitude (KA) score and HbA1C and between the KA score and fasting blood glucose.[ 24 ] However, Islam SMS et al . noted a weak negative association between diabetes knowledge score and HbA1C.[ 25 ]
Study by Palaian S et al ., resolved with contrasting results. Although knowledge scores in the test group of patients improved with health education compared with those of the control group, the investigators did not observe significant improvement in attitude or practice scores. They concluded that patient counseling improved knowledge scores, but this improved knowledge did not lead to appropriate attitudes or practices.[ 26 ]
A study in the north-eastern India pursued to determine the existing knowledge, attitude, practices, and impact of a structured health education intervention regarding diabetes in adults. There was significant improvement in knowledge after intervention regarding (a) risk factors, (b) early symptoms, (c) organs affected, (d) warning signs of hypoglycemia, and (e) personal precautions. In addition, significant improvement of positive attitude was noted (a) to motivate blood sugar testing yearly in family members after 40 years of age, (b) to undergo regular check-up, continue medication, and motivate family members. This study emphasized that there is a dire need of better health information through large scale awareness programs to change the attitude and practices of public regarding diabetes.[ 14 ]
Research in Navi Mumbai, Maharashtra, India in their study to assess knowledge, attitude, and self-care activities among type-2 diabetic patients noted that diabetics who were regularly involved in self-care practices (particularly dietary management) attained better glycemic control; there was a significant difference between knowledge toward self-care activities between the glycemic controlled versus uncontrolled cases. In self-care practices, strict diet, and glucose management significantly achieved glycemic control.[ 23 ]
Ahmed MM et al . from Department of Family Medicine, Faculty of Medicine, Cairo University, Egypt concluded from their study that educational intervention was a powerful tool to motivate patients’ knowledge and attitude and effectively improved patient glycemic control.[ 27 ] Imparting health education through SMS was a feasible and acceptable method for improving glycemic control and self-management behaviors in another Egyptian study.[ 24 ] In the study at Xiamen University, Xiamen, Fujian Province, China, the researchers observed that extension of diabetes health education toward self-management support effectively improve the self-care skills of diabetics, reduction of medical costs, and quality of life.[ 28 ]
In a U.K study, the British Pakistani women were assessed before and 6 months after intervention by questionnaire and HbA1C blood test for glycemic control. Nearly everyone improved their knowledge scores in the intervention group; illiterate women did not do as well, continuing to score less on knowledge parameters and did not show an improvement in glycemic control.[ 29 ] In the 2015 updated position statement of the ADA and the European association for the study of diabetes, it was strongly encouraged that diabetics should adhere to the newer paradigm of the management protocol of hyperglycemia in type 2 diabetes with a patient-centered approach for the best possible outcomes.[ 13 ]
Diabetes Canada Clinical Practice Guidelines Expert Committee recommended a variety of education and support programs including group classes and individual counseling sessions, with technology-based strategies (e.g., Internet-based computer programs and mobile phone apps). Diabetics need self-management education and support when first diagnosed, as well as during times when there are changes in treatment, general health, or life circumstances. Diabetics were suggested to adhere to diabetes team with a trusting and collaborative relationship, set goals for caring for disease and health, and identify tailor-made strategies.[ 16 ]
Brazilian study stressed on the importance of education and health communication guided dialogical relations and appreciation of popular knowledge, by reorienting the educational practices for self-care, to establish strategies for prevention in their study on knowledge, attitudes, and practices of self-care in individuals with DM.[ 30 ] Bangladesh researchers feel that diabetes education improves knowledge and attitudes for self-empowerment regardless of the education program tools (workshop or training package), to promote self-care skills improving the quality of life.[ 31 , 32 ] Australian research group on diabetes recommended education united with behavioral or psychological interventions as the snowball effect on knowledge and physiologic control.[ 33 ] Diabetic Association of India conducts Diabetes Education Program every month for all individuals in the field of diabetes whether patients or caregivers.[ 34 ]
Above observation and previous literature thus highlighted the positive impact of health education on knowledge, attitude, and practices of diabetic patients which can contribute in achieving desired glycemic control in type 2 DM. This study emphazises the effective role health education can play in reducing severity of disease activity in type 2 DM with regard to glycemic control and progression to complications.
In the era of practicing evidence-based health care, we have forgotten an important truth about putting people's health at the hand of people and how to care for it with a mindset to generate data of our own. The diabetics have to be willing to remove age old ideas from their mindset which is probably the first step to diabetes education. Further, health care providers at all levels have to internalize that with a paradigm shift, we have to consider not only the help of scientific feedback from the teachers and students but also from all the stakeholders of health care delivery system so that patient education should be given due importance for the success of any intervention regarding diagnosis and prognosis. In that direction, we need to overhaul our medical curriculum so that future physicians get trained to effectively communicate with the patients to impart optimum health education.[ 35 ]
Strength of the study
Few studies from India focused on impact of health education on diabetes control. This study highlighted the impact of health education on knowledge, attitude, and practices pertaining to diabetics that can be practiced in the primary care settings even in rural areas.
Limitations of the study
This was a single-center study with a small sample size.
Conclusions
In our study, effective health education resulted in improved knowledge, better attitude, and adoption of favorable practices which ultimately lead to better glycemic control in patients with type 2 DM, thus help to slow progression and prevent complications. Non-pharmacological interventions form a cornerstone of diabetes management and require favorable patient knowledge, attitude, and practices. Health education, therefore, is an essential tool in the management of diabetes. Effective patients’ education results in improved knowledge, better attitude, and adoption of right practices which transform into better glycemic control in patients with type 2 diabetes, thus slowing the progression of disease and preventing its complications. Health education is essential in resource poor primary care settings wherein DM pose great financial burden and calls for urgent participation of clinicians and health care workers to inform and motivate diabetic patients and to propagate healthy lifestyle practices vital for achieving glycemic control.
Financial support and sponsorship
Conflicts of interest.
There are no conflicts of interest.
Ohio State nav bar
The Ohio State University
- BuckeyeLink
- Find People
- Search Ohio State
Patient Education
Patient education (video script).

Welcome to this brief educational video about Type 2 Diabetes, a condition in which blood sugar levels run consistently higher than normal. In order to understand what is not working in Type 2 Diabetes, it is important for us to understand what “normal” is.

When we eat or drink, our body takes that food and drink and breaks it down into nutrients that are absorbed into our bloodstream. This includes sugar, and that is how we end up with elevated blood sugar levels. This is normal right after we eat or drink.

Our body then produces something called insulin. Insulin’s job is take that sugar out of the bloodstream and help cells use it for energy. As insulin does its job and moves the sugar from the bloodstream into the cell, blood sugar levels naturally go down. If our body needs more sugar, during times of intense exercise or when we haven’t eaten for a long time, the liver has stored sugars that it is able to release into the bloodstream. These factors together all help keep our blood sugar level basically even over time.

In Type 2 Diabetes, blood sugars can run higher than normal pretty consistently, and for a number of reasons. One is that cells can start having trouble using the insulin that is there and available. This is called insulin resistance. A second reason is because the body becomes unable to keep with the demand for insulin. It cannot produce enough, and it becomes fatigued in the effort – producing less and less insulin. And the third reason is that the liver can release extra sugar into our system that we don’t even really need.

So why does this matter? Well, the kidneys work extra hard trying to help clear the extra sugar out of our bloodstream, by releasing it into our urine. This can lead to people peeing more frequently, and because they are peeing more frequently, they’ll feel more thirsty in order to prevent dehydration. The kidneys work overtime, and as they work overtime over the period of years, it can lead to eventual kidney disease or even kidney failure.

It is possible to have Type 2 Diabetes without these symptoms, however. Other symptoms include fatigue, vision changes, frequent infections, itching, numbness or tingling, weakness, and wounds that do not heal. It is important to share with your care provider any symptoms you are experiencing or any changes to your symptoms, so they can help you create the best plan possible for your treatment. Thank you!

An official website of the United States government
The .gov means it’s official. Federal government websites often end in .gov or .mil. Before sharing sensitive information, make sure you’re on a federal government site.
The site is secure. The https:// ensures that you are connecting to the official website and that any information you provide is encrypted and transmitted securely.
- Publications
- Account settings
- My Bibliography
- Collections
- Citation manager
Save citation to file
Email citation, add to collections.
- Create a new collection
- Add to an existing collection
Add to My Bibliography
Your saved search, create a file for external citation management software, your rss feed.
- Search in PubMed
- Search in NLM Catalog
- Add to Search
Evaluating patient education: a case study of a diabetes program
- PMID: 7275640
- DOI: 10.1177/109019818000700204
This paper presents an evaluation of a diabetic education program for patients at Stephens County Hospital in Toccoa, Georgia. An analysis of covariance is employed along with multiple classification analysis to determine the effect of the program in reducing hospital readmissions. The major finding is that the teaching program is an important variable in the reduction of readmissions. A second outcome is that the occurrence of diabetic ketoacidosis (DKA) on initial admission is a second factor in determining readmissions. Patients having the benefit of instruction are less likely to experience readmission than those not being educated; those having DKA are more likely to be readmitted within six months from release than those not having this degree of loss of control. Implications of this study suggest that in designing a diabetic education program, greater attention must be focused upon the needs of the ketosis-prone patients.
PubMed Disclaimer
Similar articles
- Dissonance in nurse and patient evaluations of the effectiveness of a patient-teaching program. Adom D, Wright AS. Adom D, et al. Nurs Outlook. 1982 Feb;30(2):132-6. Nurs Outlook. 1982. PMID: 6916204 No abstract available.
- Incidence and long-term outcomes of critically ill adult patients with moderate-to-severe diabetic ketoacidosis: retrospective matched cohort study. Azevedo LC, Choi H, Simmonds K, Davidow J, Bagshaw SM. Azevedo LC, et al. J Crit Care. 2014 Dec;29(6):971-7. doi: 10.1016/j.jcrc.2014.07.034. Epub 2014 Aug 16. J Crit Care. 2014. PMID: 25220529
- Effect of post-hospital discharge telephonic intervention on hospital readmissions in a privately insured population in Australia. Hamar GB, Coberley C, Pope JE, Cottrill A, Verrall S, Larkin S, Rula EY. Hamar GB, et al. Aust Health Rev. 2018 Jun;42(3):241-247. doi: 10.1071/AH16059. Aust Health Rev. 2018. PMID: 28390471
- Effectiveness of discharge interventions from hospital to home on hospital readmissions: a systematic review. Braet A, Weltens C, Sermeus W. Braet A, et al. JBI Database System Rev Implement Rep. 2016 Feb;14(2):106-73. doi: 10.11124/jbisrir-2016-2381. JBI Database System Rev Implement Rep. 2016. PMID: 27536797 Review.
- Hospital readmission of patients with diabetes. Rubin DJ. Rubin DJ. Curr Diab Rep. 2015 Apr;15(4):17. doi: 10.1007/s11892-015-0584-7. Curr Diab Rep. 2015. Corrected and republished in: Curr Diab Rep. 2018 Mar 13;18(4):21. doi: 10.1007/s11892-018-0989-1. PMID: 25712258 Corrected and republished. Review.
- Resolution 8224: parental notification of prescription contraceptives for teenagers. American Public Health Association. American Public Health Association. Am J Public Health. 1983 Mar;73(3):346. Am J Public Health. 1983. PMID: 11643957 Free PMC article.
Publication types
- Search in MeSH
LinkOut - more resources
- MedlinePlus Health Information
- Citation Manager
NCBI Literature Resources
MeSH PMC Bookshelf Disclaimer
The PubMed wordmark and PubMed logo are registered trademarks of the U.S. Department of Health and Human Services (HHS). Unauthorized use of these marks is strictly prohibited.
- Open access
- Published: 27 September 2024
The effect of diabetes in the multifaceted relationship between education and cognitive function
- Constantin Reinke 1
BMC Public Health volume 24 , Article number: 2584 ( 2024 ) Cite this article
Metrics details
Education has been shown to be positively associated with cognitive performance. However, the pathways via lifestyle-related disease through which education is related to cognitive performance have not been sufficiently explored. Diabetes is an important lifestyle-related disease with increasing prevalence worldwide. Low education is associated with an increased risk of developing diabetes, while diabetes may also lead to a deterioration in cognitive performance. This study aims to explore if the associations between education and cognitive function is mediated by the diabetes status among older adults.
The data utilized in this study were derived from the first two waves of the Dutch Lifelines Cohort Study (2006–2015). The analyzed sample included 26,131 individuals aged 50 years or above at baseline. The baseline assessment included measurements of educational attainment (exposure) and the potential mediator diabetes. The outcome of cognitive function was assessed using age-standardized reaction times from the psychomotor function and attention tasks, as measured by the Cogstate Brief Battery. The Cogstate Brief Battery was only conducted at the follow-up assessment, not at the baseline assessment. Faster reaction times correspond to higher cognitive performance. The study employed linear and logistic regression models, in addition to a causal mediation approach which estimated the average causal mediation effect (ACME).
Higher education was associated with a lower risk of diabetes (b= -0.1976, 95%CI= -0.3354; -0.0597) compared to low or middle education as well as with faster reaction times (b= -0.2023, 95%CI= -0.2246; -0.1798), implying better cognitive function. Diabetes was associated with slower reaction times (b = 0.0617, 95%CI = 0.0162; 0.1072). Most importantly, the mediation approach identified a significant indirect effect of education on cognitive function via the diabetes status (ACME= -0.00061, 95%CI= -0.00142; -0.00011).
The findings emphasize the potentially importance of diabetes in explaining the role of education in promoting healthy cognitive function and mitigating the risk of cognitive decline. Early detection and treatment of diabetes may be particularly beneficial for individuals with low or middle levels of education in order to maintain good levels of cognitive function.
Peer Review reports
Introduction
The doubling of the population aged 60 years and older by 2050 [ 1 ], in conjunction with the continued aging of the global population, will result in a significant increase in the global challenge of cognitive decline. The decline in cognitive function affects the individual’s daily activities, resulting in a diminished quality of life and loss of independence with a high burden on caregivers and health care systems. While brain changes associated with cognitive decline are part of normal brain aging [ 2 ], certain diseases such as diabetes can accelerate neurodegeneration and also be a driver of cognitive decline [ 3 ]. In 2021, it is estimated that there are 537 million people with diabetes, with a predicted increase to 783 million by 2045 [ 4 ]. Conversely, factors such as education can compensate for or delay cognitive decline [ 5 , 6 ]. In many parts of the world, an impressive expansion of education has taken place during the last decades [ 7 ], which, however, leaves those with less education at a particular risk of disease and poor cognition [ 6 , 8 ].
As cognitive aging and brain-altering processes are irreversible, the best strategy for reducing the risk of cognitive decline or delaying the onset or progression to clinical manifestations such as dementia is to identify and address those risk factors that are amenable to modification. The Lancet Commission identified less education as well as diabetes as important modifiable risk factors for dementia [ 9 ]. Thus, a better understanding of the pathways and possible links of these two factors with cognitive function may contribute to potential strategies for preventing or rather delay cognitive decline and dementia.
Diabetes is associated with deficits in cognitive function [ 10 , 11 ] and a higher risk of cognitive impairment [ 12 ] as well as dementia [ 13 ]. Furthermore, there is evidence for a link between diabetes and brain atrophy which leads to deficits in cognitive function [ 14 ]. High blood glucose levels and hyperglycemic events affect the brain by cerebral microvascular dysfunctions [ 15 ] and can lead to brain atrophy [ 16 ]. On the other hand, diabetes is associated with higher risks of a series of cardiovascular diseases [ 17 ], which are known to be the main drivers of cognitive impairment and vascular dementia [ 18 ]. However, cognitive function can also be affected by high blood glucose levels and hyperglycemic events due to more complex pathways including oxidative stress and neuroinflammation [ 19 ]. Prior meta-analyses have indicated that diabetes exerts disparate effects on various domains of cognitive function, notably affecting psychomotoric function and attention [ 10 , 11 ].
The association between education and cognitive function is well researched [ 20 ] and established by the concept of Cognitive Reserve [ 21 ]. The accumulation of education and experience of occupational complexity over a lifetime strengthens resilience to the pathology of cognitive decline due to age-related brain changes and delays the symptoms of cognitive decline or the clinical manifestation of dementia. In addition, there is evidence that education may also interact with the level of tau protein accumulation in the brain and its role in cognitive function [ 22 ]. Education has been identified as the most important proxy measure for cognitive reserve [ 23 ] and this is true for both individuals with and without diabetes as shown by the similar relationship between cognitive reserve level and a variety of executive cognitive function scores independent from the diabetes status [ 23 ].
The risk of diabetes is lower in individuals with higher levels of education [ 24 , 25 ]. Although other determinants of socioeconomic status have been studied and associated with the diabetes risk, education has been the most frequently and consistently associated indicator [ 26 ]. The underlying mechanism for the relationship between education and diabetes is not fully understood, but factors related to lifestyle and healthy behaviors appear to play a crucial role. In particular, BMI has been identified as an important factor [ 27 , 28 ].
It is reasonable to conclude that the most influential factor in the association of education and cognition is the direct link, as evidenced by the aforementioned connections of intellectual stimulation, such as the theory of cognitive reserve [ 20 , 21 ]. Nevertheless, the pathways connecting education and cognitive functions are multifaceted and remain incompletely understood. Lower education is linked to a higher risk of lifestyle-related diseases [ 29 ], which are also associated with cognitive decline. This suggests that individuals with lower education levels may be more vulnerable to cognitive decline due to these conditions. Beside diabetes, also other life-style related diseases like cardiovascular diseases [ 30 ], vascular diseases [ 31 ], the number of chronic diseases [ 32 ] or obesity [ 33 ] are associated with worse cognitive function and represent possible determinates to play a role in the link between education and cognitive function. Among these diseases, diabetes is of particular interest because it is a modifiable risk factor for cognition in multiple ways. Prevention of diabetes and good glucose management in people with diabetes are both important to reduce the risk of cognitive decline [ 34 , 35 ].
However, the interplay of education and diabetes and the consequence for cognitive function is still less researched and remain largely unknown.
Kowall & Rathmann examined the combined effects of education and diabetes on cognitive performance using longitudinal data from more than 27 countries from the SHARE project [ 36 ]. The authors found that people with diabetes had worse cognitive performance than people without diabetes, and that people with diabetes had even worse cognitive performance if they had lower levels of education. However, because the authors found no interaction effect between education and diabetes, they concluded that the effects were additive. A small retrospective case-control study including 1537 individuals from Japan examined the pathway between socioeconomic status and dementia by evaluating lifestyle-related disease as potential mediators [ 37 ]. However, the authors did not find a significant association between educational attainment and the risk of diabetes in their data, so a conclusion about the role of diabetes as a potential mediator is limited.
Because the pathways through which education is related to cognitive performance have not been well studied, this study addressed the question of whether some of the association between education and cognitive function may operate through the diabetes status. The hypothesis is that the diabetes status partly mediates the association between education and cognitive function.
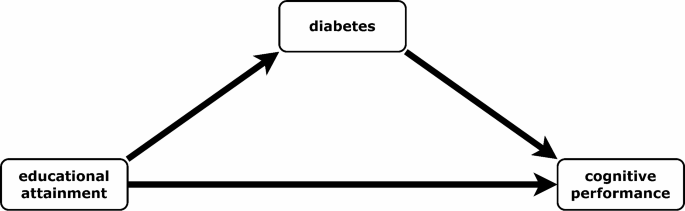
Hypothesized relationship between outcome (cognitive performance), mediator (diabetes) and exposure (educational attainment), source: Own illustration
Materials and methods
Analyses were conducted using data from the Dutch Lifelines Cohort Study. Lifelines is a multi-disciplinary prospective population-based cohort study examining in a unique three-generation design the health and health-related behaviours of 167,729 persons living in the North of the Netherlands. It employs a broad range of investigative procedures in assessing the biomedical , socio-demographic , behavioural , physical and psychological factors which contribute to the health and disease of the general population , with a special focus on multi-morbidity and complex genetics [ 38 ]. The large dataset includes information on physical examinations, biological samples, cognitive tests and a comprehensive questionnaire. Data collection was conducted between 2006 and 2013 for the baseline assessments and between 2014 and 2015 for the second assessment. Lifelines was conducted in accordance with the guidelines of the Declaration of Helsinki and has been approved by Medical ethical committee of the University Medical Center Groningen (The Netherlands) under number 2007/152. All participants signed an informed consent form.
Study design & sample
The Lifelines cohort includes 152,860 individuals aged 18 years or older at baseline. Of these, 111,959 participated in the second assessment. All individuals younger than 50 years at baseline were excluded, as were individuals with missing data on outcome (no Cogstate examination), exposure (education), mediator (diabetes), or confounders. See Fig. 2 . The final sample consisted of 26,131 individuals. To set up a study design with a causal time order, the variables for the exposure, the mediator and confounders were built by information from the baseline assessment. As the analysis only included individuals aged 50 or older at baseline, it can be assumed that their highest educational attainment was achieved well before the mediators and confounders were measured. Since the Cogstate examination was not conducted at baseline, the outcome measure was taken from the second assessment only. As a result, it was not possible to investigate the change in cognitive function over time or to adjust for baseline cognitive function.
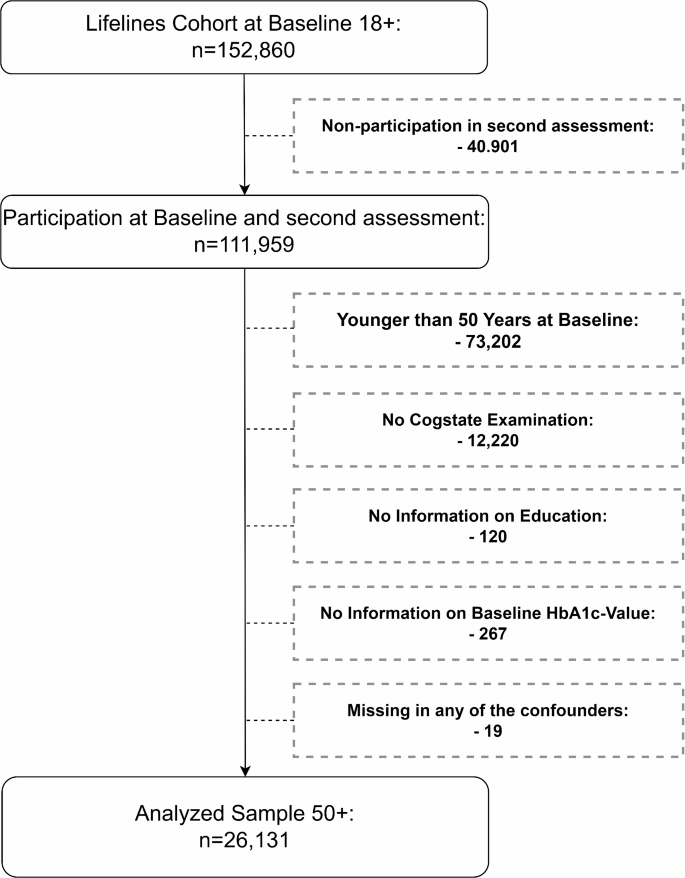
Selection of the study cohort, source: lifelines data 2006–2015, own calculation
Data availability
Data may be obtained from a third party and is not publicly available. Researchers may apply to use the Lifelines data used in this study. For information on how to request Lifelines data and terms of use are available on their website at ( https://www.lifelines.nl/researcher/how-to-apply ).
Cognitive function measure (outcome)
Individuals cognitive function was measured by tasks from the Cogstate Brief Battery at the second assessment only. The Cogstate Brief Battery is a validated computer based cognitive assessment [ 39 ]. It has been used in previous studies to detect mild cognitive impairment and cognitive impairment in Alzheimer’s disease [ 40 ] and also in the context of the Lifelines cohort [ 41 ]. The battery includes four different tasks to measure the cognitive domains of psychomotoric function (detection test), attention (identification test), visual learning (one card learning test), and working memory (one back test). Outcomes of each task were reaction time and accuracy.
Previous studies point out, that the associations of diabetes and cognitive function varied between the domains of cognitive function [ 10 , 11 ]. There is evidence that primarily the domains of psychomotoric function and attention were affected by diabetes. Therefore, a composite score from these two domains was built by the detection and identification tasks of the Cogstate Brief Battery, similar to Maruff and colleagues [ 40 ]. In order to achieve this, the log-10-transformed (closer to a normal distribution) reaction time (milliseconds) of both tests were z-standardized in 5-year age groups (from age group 50 upwards) and then summed up (Distribution: Supplementary Figure S1 ). Accordingly, a positive value indicates a higher (slower) reaction time compared to the respective age group, representing poorer cognitive function. Conversely, a negative value displays a lower (faster) reaction time, implying better cognitive function.
Educational attainment (exposure)
Individuals educational attainment was defined by the highest obtained degree at baseline. The information was self-reported through a questionnaire with the following possible responses: no education (1); primary education (2); lower or preparatory secondary vocational education (3); junior general secondary education (4); secondary vocational education or work-based learning pathway (5); senior general secondary education, pre-university secondary education (6); higher vocational education (7); university education (8); other (9). We categorized the education into two categories low-middle (1–6) and high education (7–8). Individuals who reported “other” were assigned to one of the two categories in a further step (see: https://wiki.lifelines.nl/doku.php?id=educational_attainment ).
Diabetes status (mediator)
Diabetes was defined as the presence of at least one of the following conditions at baseline: Self-reported diagnosis of diabetes, baseline HbA1c ≥ 6.5%, fasting plasma glucose ≥ 7 mmol/L, random plasma glucose ≥ 11.1 mmol/L or use of any medical diabetes treatment.
Control variables
The statistical models were adjusted for age, sex, physical activity, obesity, smoking history, income and a set of comorbidities: depression, hypertension, stroke, heart failure and high cholesterol. The models on cognitive function were also controlled for the accuracy (number correct responses/number total response) of the tasks from the Cogstate Brief Battery. All confounders were measured at the baseline assessment.
Physical activity was defined as any amount of vigorous physical activity per week. So, the variable is classified into physical active vs. not physical active persons.
Obesity was defined by a body mass index of 30 or higher.
The smoking history was classified by using the cumulative risk measure of packyears, where one pack year implies smoking 20 cigarettes (or an equivalent number of other smoking derivatives) per day for one year. The variable was categorized into: Never smokers, persons with equal or less pack years than the median of the ever smokers, persons with more pack years than the median of the ever smokers, no answer/pack years not calculable.
Income was measured by the following question: “what is your net income per month? (if you share a household, include the net income of your partner(s))”. The variable is classified into the following categories: Lower than 1500€, 1500€ − 2500€, Over 2500€, Don’t know/no answer.
All comorbidities were measured by self-report (had the person ever had the condition).
Statistical analysis
To explore the associations between education, diabetes status and cognitive function linear and logistic regression models were used. A causal mediation approach [ 42 ] was used to test whether there was an indirect effect of education ( E xposure) on cognitive function ( O utcome) through diabetes status ( M ediator) controlled for the c onfounders. This approach which based on the idea or structural equation modeling estimates the average causal mediation effect (ACME) or indirect effect. The approach included several steps: First, two statistical models were fitted separately, one to model the mediator (1) and one to model the outcome (2). In a second step, model parameters for outcome and mediator were simulated from their sampling distributions. Third, potential values for the mediator were simulated prior to the potential outcomes, given these simulated mediator values, in order to then calculate the causal mediation effects from the simulated values. Finally, the point estimation for the ACME (and direct effect) as well as the confidence interval was calculated from the simulated distribution. The approach is described in detail by Imai et al. [ 42 ]
In contrast to the classical framework of structural equation modeling, here the two models do not have to be linear regression models. Since the mediator (diabetes status) is a binary variable, it was estimated using logistic regression. While the outcome is a continuous variable, it was estimated by linear regression. Both models were estimated with robust standard errors. The causal mediation approach was performed using the mediation package in R [ 43 ].
To check the robustness of the results, a number of sensitivity analyses were applied. First, to test the sequential ignorability assumption that there are no unmeasured confounders of the mediator-outcome relation [ 44 ], the “medsens” function from the mediation package in R [ 43 ] was used. The function estimates a parameter which indicates the correlation of \(\:{\varepsilon _M}\) and \(\:{\varepsilon _o}\) at which the ACME would be zero. The function required a probit regression model instead of a logistic one.
As a further sensitivity analysis, a classical structural equation modeling approach with robust standard errors was used. However, in this classical framework both models have to be linear regression models, the mediator was the (continuous) HbA1c-level instead of the diabetes status otherwise the equations were identical to (1) and (2). After the simultaneously estimation of the outcome and the mediator model, the effect of education was decomposed in a direct and indirect effect and the Sobel test was used to check significance [ 45 ].
To test the robustness of the study design, the mediation analysis described above was also tested using indicators other than diabetes as potential mediators. In the same way as for diabetes, the indirect effect of education was tested via high blood pressure, high cholesterol and obesity.
In a further sensitivity analysis, the diabetes status was defined as the presence of at least one of the conditions mentioned in Sect. 2.5 at baseline or follow-up.
All analyses were performed using R 4.2.2 and Stata 17.
Study cohort
Our analyzed sample included 26,131 individuals of which 18,486 had a low or middle educational attainment and 7645 had a high educational attainment, and there were 1449 diabetes cases. The mean age-standardized reaction time of the outcome measure was − 0.0003 (SD = 0.8796). The mean age at baseline was 58.1 (SD = 6.6) years, with a range of 50 to 88 years. The majority of participants were between 50 and 60 years of age. The mean age was 57.9 (SD = 6.5) without and 61.1 (SD = 6.9) for persons with diabetes. The individuals are divided into 14,503 women and 11,628 men. Table 1 shows characteristics of the study cohort. When looking at the outcome, it can be noticed that the mean age-standardized reaction time for high educated people (-0.2175) is clearly lower (faster/better) than for people with low or middle education (0.0895). So, in the cognition tests individuals with high education performed faster/better compared to their age-group while low-middle educated individuals performed slower/worse. A reverse pattern was observed in diabetes. Non-diabetic individuals demonstrated faster/better cognitive performance relative to their age group than diabetic individuals.
Mediation analysis
Table 2 shows the estimated regression coefficients for the mediator model 1 (logistic) and the outcome model 2 (Ordinary Least Squares) with the corresponding confidence intervals (CI) as well as the direct and the indirect effect (ACME) of education on cognitive function. From model 1 it can be derived that higher education is associated with a lower risk of diabetes compared to low-middle education, indicated by the negative regression coefficient (-0.1976, p = 0.005). The regression coefficient (-0.2023, p < 0.001) in model 2 shows a significant association between education and cognitive function, implying that those with higher education have a lower reaction time to the outcome measure, and therefore better cognitive function than those with low or middle education. The regression coefficient for diabetes in model 2 is 0.0617 ( p = 0.008). This demonstrates that individuals with diabetes have a significantly higher reaction time to the outcome measure and therefore worse cognitive function than individuals without diabetes. The average mediation effect of education trough diabetes was − 0.00061 and the direct effect of education was − 0.20247. This results in a total effect of -0.20307. In particular, the coefficient indicates that individuals with higher levels of education, in comparison to those with low-to-middle levels of education, completed the examined tasks, on average, 0.20307 standard deviation faster (better cognitive function) than the average in their respective age group. All these effects were significantly different from zero. However, the ratio of the indirect effect to the total effect implies that the indirect effect contributes less than 1% to the total effect. The average mediation effect (-0.00061) represents the difference in the effect of education on cognitive function through the mediator. In other words, it is the total effect minus the direct effect.
The first sensitivity analysis tested the sequential ignorability assumption of the causal mediation approach. The estimated parameter ρ that would lead to an ACME of zero was 0.036 (Supplementary Figure S2 ). So even a weak pre-treatment confounder could render the effect insignificant.
In a second sensitivity analysis, a classical structural equation modelling approach was applied with HbA1c level instead of diabetes status as a potential mediator. The results of the Sobel test showed that HbA1c was also a significant mediator for education (Supplementary Table S1 ). The proportion of the indirect effect from the total effect was also less than 1%.
A further sensitivity analysis was conducted to test the robustness of the study design by using other lifestyle-related diseases as potential mediators. The results showed that there were no indirect effects (ACME not significantly different from zero) of education on cognitive function via hypertension, high cholesterol, or obesity (Supplementary Table S2 – Table S4 ).
The sensitivity analysis, which defined diabetes status based on baseline and follow-up information, resulted in a larger number of cases of diabetes (2013 vs. 1449). However, the results of the mediation analysis did not differ significantly from those presented in Table 2 (see Supplementary Table S5 ).
The question of this study was whether diabetes partly mediates the link between educational attainment and cognitive function in individuals aged 50 years and older, using a large data set from the Netherlands. The results revealed significant positive effects of higher education on cognitive function as well as a lower risk of diabetes for higher educated individuals. The most noteworthy finding was the identification of a significant indirect effect of education on cognitive function via diabetes, although this effect was relatively small.
Although the mediating effect of diabetes on the relationship between education and cognition has not been considerably studied, an earlier study did examine the interaction effect between education and diabetes [ 36 ]. They did not find one and therefore concluded that the effects of the two risk factors were purely additive. This conclusion does not rule out that the connection may be partly a mediated association. In addition to the increased risk of developing diabetes among individuals with lower levels of education, potential reasons for the association to cognition may lie in the disparities in health literacy and adherence to diabetes therapy between the educational groups. Supporting Kowall and Rathmann, worse glycemic control which is more prevalent in lower educated people with diabetes [ 46 , 47 ] and the association of worse glycemic control with cognitive dysfunction [ 48 ] may be a mechanism here. Treatment recommendations for glycemic control are challenging and include diabetes self-management by monitoring of blood glucose, use of medication as well as physical activity and nutrition/diet [ 49 ]. This health-related behaviors are linked to education [ 46 , 50 , 51 ]. Furthermore, the compliance of diabetes self-management decreases over time [ 52 ] and there is evidence that individuals with lower levels of education are at an increased risk of developing diabetes complications [ 53 ] which point out the possible link to cognitive function. This may give a higher potential for reducing the burden of cognitive decline in lower educated people by avoiding diabetes as well as diabetes complications through improving diabetes self-management and adherence.
Nakahori and colleagues [ 37 ] concluded that diabetes plays a minimal role in the link between educational attainment and dementia. This was not surprising as their study showed no significant association between education and diabetes, or between education and other dementia risk factors such as smoking. However, the authors emphasized that their findings are limited to Japan. In contrast to the findings of Nakahori et al., this study confirmed the existing evidence for the connection of education and diabetes [ 24 , 25 , 31 ] and argued that diabetes plays a significant role in the link between education and cognitive function. It should be noted that Nakahori et al. employed dementia as outcome, rather than cognitive function. Nevertheless, both are closely related and share a significant number of risk factors. A further reason for the disparate findings may be attributed to the differing definitions of diabetes applied. While Nakahori et al. only include diagnosed cases of diabetes, this study also incorporates individual’s laboratory results, which cover undiagnosed cases of diabetes. The link between undiagnosed diabetes and cognitive function appears to be particularly strong [ 54 ].
One of the main strengths of our study is its large sample size. The lifelines cohort covers about 10% of the population of the northern Netherlands. In this study more than 26,000 people were included and analyzed using information from questionnaires, measurements, and blood sample data. Central to the validity of the study is the utilization of a validated and well-established measure for assessing cognitive function. A composite score of cognitive function was constructed using tests from the Cogstate Brief Battery. This score was derived from two domains: psychomotor function and attention. There is a body of evidence indicating that these domains are associated with diabetes [ 10 , 11 ]. An additional strength of our study is the comprehensive definition of diabetes, which includes both diagnosed and undiagnosed cases by incorporating the HbA1c-level from the blood sample. Furthermore, the statistical models were adjusted for a set of life style-related confounders. The sensitivity analyses concerning a further statistical approach, as well as the robustness of the study design using other possible mediators or extended definition of diabetes represent further strengths of this study.
Despite the strengths of this study, it is important to acknowledge its limitations. The Cogstate Brief Battery was not assessed at baseline; thus, it was not possible to examine changes in cognitive function in the context of the analyses. Moreover, the cognitive function at follow-up is supposed to be affected by the cognitive function at baseline; however, it was not possible to adjust the analysis for this information. In light of the findings of this study, it can be concluded that the outcome measure of cognitive function encompasses both the baseline information and the change from baseline to follow-up. Consequently, it is not possible to interpret these elements separately. It is relevant to consider this when interpreting the results of the study. It is necessary for future studies to take this issue into account in order to strengthen the validity of these findings. Considering the sensitivity analysis and the low robustness to the assumption of pre-treatment confounding, it is necessary to reflect on the results with this in mind. In particular, genetic factors [ 55 , 56 , 57 ] may be important, but further unobserved factors may also be pre-treatment factors that are connected to education, diabetes, and cognitive function such as e.g. the socioeconomic background in childhood [ 58 , 59 ].
The multifaceted relationship between education and cognition has already been pointed out, and several lifestyle factors that are related to education, diabetes and cognition have been controlled in the model. Nevertheless, the complex relationship with nutrition could not be modelled, even if obesity was included in the statistical model. However, we did not find a significant pathway via hypertension, high cholesterol, or obesity in our statistical model which strengthens the importance of diabetes as one of the multifaceted pathways between education and cognition.
Further issues concern the analyzed sample. Population-based health surveys are typically affected by selection or response bias, which leads to a healthier study sample than in the underlying population. This is also suspected here, with a diabetes prevalence of 5.7% for the study sample (age 50+) towards 7.5% in the Netherland population aged 20–79 [ 60 ]. It is reasonable to assume that this also applies to individuals with impaired cognitive function. This may result in an underestimation of the effects in the statistical models and with it the size/proportion of the indirect effect of education on cognitive function. Moreover, the follow-up period between the baseline and second assessment was relatively short given the slow progression of diabetes and cognitive decline.
The relationship between education and cognitive abilities is well established and again evidenced by the findings of this study. Education, particularly in older age, is not a modifiable risk factor, whereas diabetes is. Thus, in the multifaceted relationship between education and cognition, diabetes represents one promising approach to modifying or preventing the risk of cognitive decline thereby counteracting the disadvantages of less education. This holds true, even if the association between education and cognitive function may be mediated by a series of factors, among them most prominently cognitive reserve.
Further research is needed to reveal how cognitive performance changes over time in the different educational groups. Additionally, the interplay between genetic predisposition, education and cognitive function should be explored.
This study found that people with lower levels of education were more likely to have diabetes and that diabetes was associated with poor cognitive function. Most importantly, this study is the first to demonstrate that a part of the effect of education on cognitive function runs through diabetes. While the relationship between education and cognition is multifaceted, these findings emphasize the potentially importance of diabetes in explaining the role of education in promoting healthy cognitive function and mitigating the risk of cognitive decline. Lower and middle educated people are double disadvantaged with respect to cognitive function through a higher risk for diabetes as well as a lower cognitive reserve resulting from lower education. Early detection and treatment of diabetes may be particularly beneficial for these individuals to maintain good levels of cognitive function.
Data may be obtained from a third party and is not publicly available. Researchers may apply to use the Lifelines data used in this study. For information on how to request Lifelines data and terms of use are available on their website at (https://www.lifelines.nl/researcher/how-to-apply).
Abbreviations
Average causal mediation effect
Confidence interval
Glycated hemoglobin (A1c)
Millimole per liter
Standard deviation
University Medical Center Groningen
World Health Organization. Ageing and health: Key facts. 2022. https://www.who.int/news-room/fact-sheets/detail/ageing-and-health . Accessed 14 Jun 2024.
Ferreira LK, Busatto GF. Resting-state functional connectivity in normal brain aging. Neurosci Biobehav Rev. 2013;37:384–400. https://doi.org/10.1016/j.neubiorev.2013.01.017 .
Article PubMed Google Scholar
Verdile G, Fuller SJ, Martins RN. The role of type 2 diabetes in neurodegeneration. Neurobiol Dis. 2015;84:22–38. https://doi.org/10.1016/j.nbd.2015.04.008 .
Article CAS PubMed Google Scholar
Sun H, Saeedi P, Karuranga S, Pinkepank M, Ogurtsova K, Duncan BB, et al. IDF Diabetes Atlas: Global, regional and country-level diabetes prevalence estimates for 2021 and projections for 2045. Diabetes Res Clin Pract. 2022;183:109119. https://doi.org/10.1016/j.diabres.2021.109119 .
Lenehan ME, Summers MJ, Saunders NL, Summers JJ, Vickers JC. Relationship between education and age-related cognitive decline: a review of recent research. Psychogeriatrics. 2015;15:154–62. https://doi.org/10.1111/psyg.12083 .
Zahodne LB, Stern Y, Manly JJ. Differing effects of education on cognitive decline in diverse elders with low versus high educational attainment. Neuropsychology. 2015;29:649–57. https://doi.org/10.1037/neu0000141 .
Lutz KCS. The human core of the shared socioeconomic pathways: Population scenarios by age, sex and level of education for all countries to 2100. Glob Environ Change. 2017;42:181–92. https://doi.org/10.1016/j.gloenvcha.2014.06.004 .
Article PubMed PubMed Central Google Scholar
Beydoun MA, Beydoun HA, Gamaldo AA, Teel A, Zonderman AB, Wang Y. Epidemiologic studies of modifiable factors associated with cognition and dementia: systematic review and meta-analysis. BMC Public Health. 2014;14:643. https://doi.org/10.1186/1471-2458-14-643 .
Livingston G, Huntley J, Sommerlad A, Ames D, Ballard C, Banerjee S, et al. Dementia prevention, intervention, and care: 2020 report of the Lancet Commission. Lancet. 2020;396:413–46. https://doi.org/10.1016/S0140-6736(20)30367-6 .
Monette MCE, Baird A, Jackson DL. A meta-analysis of cognitive functioning in nondemented adults with type 2 diabetes mellitus. Can J Diabetes. 2014;38:401–8. https://doi.org/10.1016/j.jcjd.2014.01.014 .
Palta P, Schneider ALC, Biessels GJ, Touradji P, Hill-Briggs F. Magnitude of cognitive dysfunction in adults with type 2 diabetes: a meta-analysis of six cognitive domains and the most frequently reported neuropsychological tests within domains. J Int Neuropsychol Soc. 2014;20:278–91. https://doi.org/10.1017/S1355617713001483 .
Dove A, Shang Y, Xu W, Grande G, Laukka EJ, Fratiglioni L, Marseglia A. The impact of diabetes on cognitive impairment and its progression to dementia. Alzheimers Dement. 2021;17:1769–78. https://doi.org/10.1002/alz.12482 .
Xue M, Xu W, Ou Y-N, Cao X-P, Tan M-S, Tan L, Yu J-T. Diabetes mellitus and risks of cognitive impairment and dementia: a systematic review and meta-analysis of 144 prospective studies. Ageing Res Rev. 2019;55:100944. https://doi.org/10.1016/j.arr.2019.100944 .
Zhang T, Shaw M, Cherbuin N. Association between type 2 diabetes Mellitus and Brain Atrophy: a Meta-analysis. Diabetes Metab J. 2022;46:781–802. https://doi.org/10.4093/dmj.2021.0189 .
Article CAS PubMed PubMed Central Google Scholar
van Sloten TT, Sedaghat S, Carnethon MR, Launer LJ, Stehouwer CDA. Cerebral microvascular complications of type 2 diabetes: stroke, cognitive dysfunction, and depression. Lancet Diabetes Endocrinol. 2020;8:325–36. https://doi.org/10.1016/S2213-8587(19)30405-X .
Moran C, Phan TG, Chen J, Blizzard L, Beare R, Venn A, et al. Brain atrophy in type 2 diabetes: regional distribution and influence on cognition. Diabetes Care. 2013;36:4036–42. https://doi.org/10.2337/dc13-0143 .
Viigimaa M, Sachinidis A, Toumpourleka M, Koutsampasopoulos K, Alliksoo S, Titma T. Macrovascular complications of type 2 diabetes Mellitus. Curr Vasc Pharmacol. 2020;18:110–6. https://doi.org/10.2174/1570161117666190405165151 .
Johansen MC, Langton-Frost N, Gottesman RF. The role of Cardiovascular Disease in Cognitive Impairment. Curr Geri Rep. 2020;9:1–9. https://doi.org/10.1007/s13670-020-00309-7 .
Article Google Scholar
Gupta M, Pandey S, Rumman M, Singh B, Mahdi AA. Molecular mechanisms underlying hyperglycemia associated cognitive decline. IBRO Neurosci Rep. 2023;14:57–63. https://doi.org/10.1016/j.ibneur.2022.12.006 .
Panico F, Sagliano L, Magliacano A, Santangelo G, Trojano L. The relationship between cognitive reserve and cognition in healthy adults: a systematic review. Curr Psychol. 2022. https://doi.org/10.1007/s12144-022-03523-y .
Stern Y. Cognitive reserve in ageing and Alzheimer’s disease. Lancet Neurol. 2012;11:1006–12. https://doi.org/10.1016/S1474-4422(12)70191-6 .
Yasuno F, Minami H, Hattori H. Interaction effect of Alzheimer’s disease pathology and education, occupation, and socioeconomic status as a proxy for cognitive reserve on cognitive performance: in vivo positron emission tomography study. Psychogeriatrics. 2020;20:585–93. https://doi.org/10.1111/psyg.12552 .
Peña-González P, Mondragón-Maya A, Silva-Pereyra J, Roa-Rojas P. Cognitive Reserve and executive functions in adults with type 2 diabetes. J Diabetes Res. 2020;2020:7941543. https://doi.org/10.1155/2020/7941543 .
Mathisen J, Jensen AKG, Andersen I, Andersen GS, Hvidtfeldt UA, Rod NH. Education and incident type 2 diabetes: quantifying the impact of differential exposure and susceptibility to being overweight or obese. Diabetologia. 2020;63:1764–74. https://doi.org/10.1007/s00125-020-05150-3 .
Whitaker SM, Bowie JV, McCleary R, Gaskin DJ, LaVeist TA, Thorpe RJ. The Association between Educational Attainment and Diabetes among men in the United States. Am J Mens Health. 2014;8:349–56. https://doi.org/10.1177/1557988313520034 .
Agardh E, Allebeck P, Hallqvist J, Moradi T, Sidorchuk A. Type 2 diabetes incidence and socio-economic position: a systematic review and meta-analysis. Int J Epidemiol. 2011;40:804–18. https://doi.org/10.1093/ije/dyr029 .
Steele CJ, Schöttker B, Marshall AH, Kouvonen A, O’Doherty MG, Mons U, et al. Education achievement and type 2 diabetes-what mediates the relationship in older adults? Data from the ESTHER study: a population-based cohort study. BMJ Open. 2017;7:e013569. https://doi.org/10.1136/bmjopen-2016-013569 .
Zhang J, Chen Z, Pärna K, van Zon SKR, Snieder H, Thio CHL. Mediators of the association between educational attainment and type 2 diabetes mellitus: a two-step multivariable mendelian randomisation study. Diabetologia. 2022. https://doi.org/10.1007/s00125-022-05705-6 .
Sommer I, Griebler U, Mahlknecht P, Thaler K, Bouskill K, Gartlehner G, Mendis S. Socioeconomic inequalities in non-communicable diseases and their risk factors: an overview of systematic reviews. BMC Public Health. 2015;15:914. https://doi.org/10.1186/s12889-015-2227-y .
Letellier N, Ilango SD, Mortamais M, Tzourio C, Gabelle A, Empana J-P, et al. Socioeconomic inequalities in dementia risk among a French population-based cohort: quantifying the role of cardiovascular health and vascular events. Eur J Epidemiol. 2021;36:1015–23. https://doi.org/10.1007/s10654-021-00788-8 .
Liu C, Ma Y, Hofman A, Waziry R, Koton S, Pike JR, et al. Educational Attainment and Dementia: mediation by Mid-life Vascular Risk factors. Ann Neurol. 2023;94:13–26. https://doi.org/10.1002/ana.26647 .
Ihle A, Oris M, Fagot D, van der Chicherio C, Sauter J, Kliegel M. Associations of educational attainment and cognitive level of job with old age verbal ability and processing speed: the mediating role of chronic diseases. Appl Neuropsychol Adult. 2018;25:356–62. https://doi.org/10.1080/23279095.2017.1306525 .
Ihle A, Mons U, Perna L, Oris M, Fagot D, Gabriel R, Kliegel M. The relation of obesity to performance in verbal abilities, Processing Speed, and cognitive flexibility in Old Age: the role of Cognitive Reserve. Dement Geriatr Cogn Disord. 2016;42:117–26. https://doi.org/10.1159/000448916 .
Yaffe K, Falvey C, Hamilton N, Schwartz AV, Simonsick EM, Satterfield S, et al. Diabetes, glucose control, and 9-year cognitive decline among older adults without dementia. Arch Neurol. 2012;69:1170–5. https://doi.org/10.1001/archneurol.2012.1117 .
Zheng B, Su B, Price G, Tzoulaki I, Ahmadi-Abhari S, Middleton L, Glycemic, Control. Diabetic complications, and risk of dementia in patients with diabetes: results from a large U.K. Cohort Study. Diabetes Care. 2021;44:1556–63. https://doi.org/10.2337/dc20-2850 .
Kowall B, Rathmann W. Combined effects of Diabetes and Education on decline of cognitive performance in the older Population: the Survey of Health, Ageing, and Retirement in Europe. Gerontology. 2023;69:172–80. https://doi.org/10.1159/000524571 .
Nakahori N, Sekine M, Yamada M, Tatsuse T, Kido H, Suzuki M. A pathway from low socioeconomic status to dementia in Japan: results from the Toyama dementia survey. BMC Geriatr. 2018. https://doi.org/10.1186/s12877-018-0791-6 .
Scholtens S, Smidt N, Swertz MA, Bakker SJL, Dotinga A, Vonk JM, et al. Cohort Profile: LifeLines, a three-generation cohort study and biobank. Int J Epidemiol. 2015;44:1172–80. https://doi.org/10.1093/ije/dyu229 .
Lim YY, Jaeger J, Harrington K, Ashwood T, Ellis KA, Stöffler A, et al. Three-month stability of the CogState brief battery in healthy older adults, mild cognitive impairment, and Alzheimer’s disease: results from the Australian imaging, biomarkers, and lifestyle-rate of change substudy (AIBL-ROCS). Arch Clin Neuropsychol. 2013;28:320–30. https://doi.org/10.1093/arclin/act021 .
Maruff P, Lim YY, Darby D, Ellis KA, Pietrzak RH, Snyder PJ, et al. Clinical utility of the cogstate brief battery in identifying cognitive impairment in mild cognitive impairment and Alzheimer’s disease. BMC Psychol. 2013;1:30. https://doi.org/10.1186/2050-7283-1-30 .
Aretz B, Janssen F, Vonk JM, Heneka MT, Boezen HM, Doblhammer G. Long-term exposure to fine particulate matter, lung function and cognitive performance: a prospective Dutch cohort study on the underlying routes. Environ Res. 2021;201:111533. https://doi.org/10.1016/j.envres.2021.111533 .
Imai K, Keele L, Tingley D. A general approach to causal mediation analysis. Psychol Methods. 2010;15:309–34. https://doi.org/10.1037/a0020761 .
Tingley D, Yamamoto T, Hirose K, Keele L, Imai K. Mediation : R Package for Causal Mediation Analysis. J Stat Soft. 2014. https://doi.org/10.18637/jss.v059.i05 .
Imai K, Keele L, Yamamoto T, Identification. Inference and sensitivity analysis for Causal Mediation effects. Statist Sci. 2010. https://doi.org/10.1214/10-STS321 .
Sobel. Direct and Indirect effects in Linear Structural equation models. Sociol Methods Res. 1987;16:155–76. https://doi.org/10.1177/0049124187016001006 .
Houle J, Lauzier-Jobin F, Beaulieu M-D, Meunier S, Coulombe S, Côté J, et al. Socioeconomic status and glycemic control in adult patients with type 2 diabetes: a mediation analysis. BMJ Open Diabetes Res Care. 2016;4:e000184. https://doi.org/10.1136/bmjdrc-2015-000184 .
Zeng Y, Wu J, Han Y, Chen F, Chen L, Yang S, Fang Y. Educational disparities in the associations between self-monitoring of blood glucose and glycemic control in type 2 diabetes patients in Xiamen, China. J Diabetes. 2018;10:715–23. https://doi.org/10.1111/1753-0407.12651 .
Mansur RB, Lee Y, Zhou AJ, Carmona NE, Cha DS, Rosenblat JD, et al. Determinants of cognitive function in individuals with type 2 diabetes mellitus: a meta-analysis. Ann Clin Psychiatry. 2018;30:38–50.
PubMed Google Scholar
Garber AJ, Abrahamson MJ, Barzilay JI, Blonde L, Bloomgarden ZT, Bush MA, CONSENSUS STATEMENT BY THE AMERICAN ASSOCIATION OF CLINICAL ENDOCRINOLOGISTS AND AMERICAN COLLEGE OF ENDOCRINOLOGY ON THE COMPREHENSIVE TYPE 2 DIABETES MANAGEMENT ALGORITHM–2016 EXECUTIVE SUMMARY, et al. Endocr Pract. 2016;22:84–113. https://doi.org/10.4158/EP151126.CS .
Karter AJ, Stevens MR, Brown AF, Duru OK, Gregg EW, Gary TL, et al. Educational disparities in health behaviors among patients with diabetes: the translating Research Into Action for Diabetes (TRIAD) Study. BMC Public Health. 2007;7:308. https://doi.org/10.1186/1471-2458-7-308 .
Chen P, Callisaya M, Wills K, Greenaway T, Winzenberg T. Cognition, educational attainment and diabetes distress predict poor health literacy in diabetes: a cross-sectional analysis of the SHELLED study. PLoS ONE. 2022;17:e0267265. https://doi.org/10.1371/journal.pone.0267265 .
Shrivastava SR, Shrivastava PS, Ramasamy J. Role of self-care in management of diabetes mellitus. J Diabetes Metab Disord. 2013;12:14. https://doi.org/10.1186/2251-6581-12-14 .
Liao Y-S, Tsai W-C, Chiu L-T, Kung P-T. Educational attainment affects the diagnostic time in type 2 diabetes mellitus and the mortality risk of those enrolled in the diabetes pay-for-performance program. Health Policy. 2023;138:104917. https://doi.org/10.1016/j.healthpol.2023.104917 .
Yang J, Xu H, Li J, Zhao Y, Guan S, Fu Y, et al. The association between undiagnosed diabetes and cognitive function: findings from the China health and retirement longitudinal study. BMC Endocr Disord. 2022;22:151. https://doi.org/10.1186/s12902-022-01055-x .
Guerrero-Berroa E, Ravona-Springer R, Heymann A, Schmeidler J, Levy A, Leroith D, Beeri MS. Haptoglobin genotype modulates the relationships of glycaemic control with cognitive function in elderly individuals with type 2 diabetes. Diabetologia. 2015;58:736–44. https://doi.org/10.1007/s00125-014-3487-2 .
Baumgaertel J, Haussmann R, Gruschwitz A, Werner A, Osterrath A, Lange J, et al. Education and genetic risk modulate hippocampal structure in Alzheimer’s Disease. Aging Dis. 2016;7:553–60. https://doi.org/10.14336/AD.2016.0305 .
Lee M, Hughes TM, George KM, Griswold ME, Sedaghat S, Simino J, Lutsey PL. Education and Cardiovascular Health as Effect modifiers of APOE ε4 on dementia: the atherosclerosis risk in communities Study. J Gerontol Biol Sci Med Sci. 2022;77:1199–207. https://doi.org/10.1093/gerona/glab299 .
Article CAS Google Scholar
Kaplan GA, Turrell G, Lynch JW, Everson SA, Helkala EL, Salonen JT. Childhood socioeconomic position and cognitive function in adulthood. Int J Epidemiol. 2001;30:256–63. https://doi.org/10.1093/ije/30.2.256 .
Bertola L, Benseñor IM, Barreto SM, Giatti L, Moreno AB, Viana MC, et al. Early life socioeconomic status predicts cognition regardless of education level. Eur J Neurol. 2021;28:3972–8. https://doi.org/10.1111/ene.15042 .
International Diabetes Federation. IDF Diabetes Atlas. 2013.
Download references
Acknowledgements
The services of the Lifelines Cohort Study, the contributing research centers delivering data to Lifelines, and all the study participants are gratefully acknowledged. The Lifelines initiative has been made possible by subsidy from the Dutch Ministry of Health, Welfare and Sport, the Dutch Ministry of Economic Affairs, the University Medical Center Groningen (UMCG), Groningen University and the Provinces in the North of the Netherlands (Drenthe, Friesland, Groningen).
This research did not receive any specific grant from funding agencies in the public, commercial, or not-for-profit sectors.
Open Access funding enabled and organized by Projekt DEAL.
Author information
Authors and affiliations.
Institute for Sociology and Demography, University of Rostock, Ulmenstr. 69, 18057, Rostock, Germany
Constantin Reinke
You can also search for this author in PubMed Google Scholar
Contributions
This paper was written by a single author. The author was responsible for all aspects of the research process, including the study design and conception, the analysis and interpretation of data, and preparation of the manuscript.
Corresponding author
Correspondence to Constantin Reinke .
Ethics declarations
Ethics approval and consent to participate.
The Lifelines study was conducted in accordance with the guidelines of the Declaration of Helsinki and has been approved by Medical ethical committee of the University Medical Center Groningen (The Netherlands) under number 2007/152. All participants signed an informed consent form.
Consent for publication
Not applicable.
Competing interests
The authors declare no competing interests.
Additional information
Publisher’s note.
Springer Nature remains neutral with regard to jurisdictional claims in published maps and institutional affiliations.
Electronic supplementary material
Below is the link to the electronic supplementary material.
Supplementary Material 1
Rights and permissions.
Open Access This article is licensed under a Creative Commons Attribution 4.0 International License, which permits use, sharing, adaptation, distribution and reproduction in any medium or format, as long as you give appropriate credit to the original author(s) and the source, provide a link to the Creative Commons licence, and indicate if changes were made. The images or other third party material in this article are included in the article’s Creative Commons licence, unless indicated otherwise in a credit line to the material. If material is not included in the article’s Creative Commons licence and your intended use is not permitted by statutory regulation or exceeds the permitted use, you will need to obtain permission directly from the copyright holder. To view a copy of this licence, visit http://creativecommons.org/licenses/by/4.0/ .
Reprints and permissions
About this article
Cite this article.
Reinke, C. The effect of diabetes in the multifaceted relationship between education and cognitive function. BMC Public Health 24 , 2584 (2024). https://doi.org/10.1186/s12889-024-20156-x
Download citation
Received : 24 July 2024
Accepted : 23 September 2024
Published : 27 September 2024
DOI : https://doi.org/10.1186/s12889-024-20156-x
Share this article
Anyone you share the following link with will be able to read this content:
Sorry, a shareable link is not currently available for this article.
Provided by the Springer Nature SharedIt content-sharing initiative
- Cognitive function
- Population study
BMC Public Health
ISSN: 1471-2458
- General enquiries: [email protected]

IMAGES
VIDEO
COMMENTS
A 69-year-old man with uncontrolled type 2 diabetes and complex comorbidities is seen by an advanced practice nurse in a diabetes specialty clinic. The nurse performs a comprehensive assessment, prescribes medication, and educates the patient on diabetes self-care and management.
A retrospective case-control study: 4.587 participants: 6: The effect of diabetes self-management education on Hba1c level and fasting blood sugar in type 2 diabetes mellitus patients in primary health care in Binjai City of North Sumatera, Indonesia: Rusdiana, Savira M, Amelia R (2018)
The first 6 weeks consisted of culturally tailored diabetes education, which modified the evidence-based BASICS curriculum developed by the International Diabetes Center and covered basic diabetes knowledge and management skills (Peek, Harmon, et al., 2012). The curriculum was adapted to meet the literacy, adult-learning, and cultural needs of ...
Seven studies focused on diabetes education targeting school personnel, ... Nine studies were theory-based. Case Management (n=3), 68,69,75 the Healthy Learner Model (n=2) 46,66 and the Social Cognitive Theory (n=2) 70,77 served as main theoretical frameworks for the development of the study interventions.
Learn how diabetes care and education specialists (DCESs) can improve quality outcomes and reduce the burden of diabetes through evidence-based standards and quality improvement strategies. DCESs are certified health professionals who provide comprehensive diabetes care, education, and support in various settings and models.
Purpose: The primary purpose of this study is to report a systematic review of evidence and gaps in the literature among well-conducted studies assessing the impact of diabetes education on hypoglycemia outcomes and secondarily reporting the impact on other included target outcomes. Methods: The authors used a modified Cochrane method to systematically search and review English-language titles ...
Diabetes In Practice: Case Studies with Commentary. Edited by: Boris Draznin, MD, PhD. ... Misleading Diabetes: A Case of Type B Insulin Resistance Associated With Lupus Nephritis and Autoimmune Hepatitis in another window. ... National Medical Academy for Postgraduate Education. 2 Center for Innovative Medical Technologies, Kiev, ...
This review is focused on education videos for people diagnosed with T2DM or T1DM. Studies focusing on education videos for the prevention of diabetes or targeted at people at risk of diabetes (e.g., with obesity or heart disease) were not included. Studies focused on diabetes insipidus and gestational diabetes mellitus were also excluded.
Diabetes is a complex and challenging disease that requires daily self-management decisions made by the person with diabetes. Diabetes self-management education and support (DSMES) addresses the comprehensive blend of clinical, educational, psychosocial, and behavioral aspects of care needed for daily self-management and provides the foundation to help all people with diabetes navigate their ...
In some studies, the professionals underwent a period of training before starting the diabetes education (Jutterström et al., 2016; Khunti et al., 2012; Mash et al., 2014; Odnoletkova et al., 2016). The importance of specialization in health personnel to carry out diabetes education is therefore highlighted in these studies.
Find online and in-person training materials for school staff to care for students with diabetes. Learn about diabetes technology, legal protections, case studies, and more from the ADA's Safe at School campaign.
In 2017, a position statement supporting the role of the diabetes educator in inpatient diabetes management was published by the American Association of Diabetes Educators, now known as the Association of Diabetes Care & Education Specialists (ADCES). 1 Since this original publication, the impact of diabetes on the population of the United States has led to new research, policies, and care models.
Introduction. Type 2 diabetes currently affects an estimated 10.5% of the U.S. population, and is a significant cause of life-altering complications. 1 Although it is well established that optimization of glycemic management, smoking cessation, and medication-based interventions to control blood pressure and cholesterol can significantly decrease the likelihood of developing diabetic ...
These asynchronous case studies focus on how behavioral health professionals can help people from different cultures and backgrounds manage diabetes. This program includes interactive modules with several patient videos and allows learners to practice treating people with diabetes. This program will allow learners to:
Case Study Competition Abbott Case Study Competition 2018 The 2018 Abbott Case Study Competition is run to acknowledge and reward case studies that address contemporary issues in the practice of diabetes care, diabetes education and self-management in the use of flash glucose monitoring and ambulatory glucose profile.
One of the key aspects in the process of caring for people with diabetes is Therapeutic Education (TE). TE is a teaching process for training patients so that they can self-manage their care plan. Alongside traditional methods of providing educational content, there are now alternative forms of delivery thanks to the implementation of advanced Information Technologies systems such as ...
Case Study. History • 77 year old Caucasian male here for initial diabetes education • Diabetes Hx: Diagnosed 4 years ago • Complications and Comorbidities: - Hypertension (1988) - Dyslipidemia (2001) - Diabetes Type 2 (2005) - Dementia (2008) - Hx of TIA (March 2009)
Diabetes is a complex and challenging disease that requires daily self-management decisions made by the person with diabetes. Diabetes self-management education and support (DSMES) addresses the comprehensive blend of clinical, educational, psychosocial, and behavioral aspects of care needed for daily self-management and provides the foundation to help all people with diabetes navigate their ...
This case-control study, conducted at the department of Medicine of a tertiary care teaching hospital in north-west India, enrolled 100 diabetics randomized into 50 cases and 50 controls. ... Few studies from India focused on impact of health education on diabetes control. This study highlighted the impact of health education on knowledge ...
In Type 2 Diabetes, blood sugars can run higher than normal pretty consistently, and for a number of reasons. One is that cells can start having trouble using the insulin that is there and available. This is called insulin resistance. A second reason is because the body becomes unable to keep with the demand for insulin.
Evaluating patient education: a case study of a diabetes program Health Educ Q. Summer 1980;7(2):148-58. doi: 10.1177/109019818000700204. Authors J S Legge Jr, V M Massey, C I Vena, B J Reilly. PMID: 7275640 DOI: 10.1177/109019818000700204 Abstract This paper presents an evaluation of a diabetic education program for patients at Stephens County ...
Diabetes Case Studies: Real Problems, Practical Solutions. Edited by: Boris Draznin, MD, PhD, Cecilia C ... Open the PDF Link PDF for Case 1: Maturity-Onset Diabetes of the Young (MODY) as a Diagnostic Possibility in another ... 2 National Medical Academy for Postgraduate Education, Kiev, Ukraine. Search for other works by this author on: This ...
However, because the authors found no interaction effect between education and diabetes, they concluded that the effects were additive. A small retrospective case-control study including 1537 individuals from Japan examined the pathway between socioeconomic status and dementia by evaluating lifestyle-related disease as potential mediators ...
A registered dietitian, BC-ADM, helps a patient with type 1 diabetes transition to insulin pump therapy and improve his blood glucose control. The case study illustrates the advanced practice role of dietitians in diabetes care, including assessment, diagnosis, planning, and intervention.