- Mobile Apps
- Subscribe Now


Secondary Menu
- Art & Leisure
- Classifieds
The nature and meaning of physical education
To define physical education, we need to say that it is an educational process that aims to improve human development and performance through physical activity. It tends to take place at school through formal lessons, but it also includes informal activity such as play.
Physical education is a process of learning, the context being mainly physical. The purpose of this process is to develop specific knowledge, skills, and understanding and to promote physical competence.
Different sporting activities can and do contribute to this learning process, and the learning process enables participation in sports. The focus, however, is on the child and his or her development of physical competence rather than the activity.
Physical education has a holistic view within a societal context that identifies the interdependence of personal health with societal health and environmental health.
On an individual level, physical education is an agent for health and wellness that can promote personal responsibility and control for active lifestyles. However, equally as important, physical education focuses students' attention on understanding the problems of the social environment that may inhibit them and others from pursuing active lifestyles.
Headlines Delivered to Your Inbox
Physical education contributes to individual wellness through the innate 'experience of the moment' and is reinforced on a daily basis through the knowledge, skills, and feelings of enhanced self-esteem and wellness that develop over time.
Physical education is an area in which physical activity is valued and integrated into daily living. It is anchored in three fundamental axioms that lead to these guiding principles:
Individual: It recognises that people are active for all sorts of reasons - work, play, challenge and achievement, health and personal development, contemplation and relaxation, creative and cultural expression, and social interaction.
Social: It focuses on the individual, but it also recognises that social norms and values, available resources, influential learners, and other factors affect our choices and opportunities for participation. Our choices, in turn, affect these factors.
Inclusive: It provides essential ways to express who we are as individuals or groups. It is a right of all, regardless of ability, age, gender, race, ethnic background, religion, socio-economic status, or educational achievement.
Importance of
Physical education.
The importance of physical education and physical activity in our society is encouraged by a number of guiding principles entrenched in active living such as:
1. Promotes a way of life in which physical activity is
valued, enjoyed, and integrated into daily life.
2. Promotes the principle of individual choice by responding to learners' individual needs, interests, and circumstances.
3. Provides a unique contribution to lifelong development of all learners, enhancing their physical, cognitive, social, emotional, and spiritual well-being.
4. Facilitates learning processes, which encourage critical thinking, thereby affecting the learners'
personal wellness and the well-being of society.
5. Nurtures individual self-reflection and consciousness, which preserves human rights and the development of supportive and sustainable environments.
Physical education on a daily basis establishes the foundations for active living by providing experiences, which enhance the learner's knowledge, attitudes, and skills towards a wellness lifestyle. Physical education as a medium for active living in a school setting engages the whole person in the following ways:
Physically - through high-level participation in appropriately selected activities.
Mentally - through concentration and intensity while learning new concepts and skills.
Emotionally - through the confidence that comes from enjoying established skills.
Socially - through associating with others.
Spiritually - through satisfaction, contentment, and a sense of inner peace.
NEXT WEEKS' TOPIC: The nature and meaning of sports.
n Send questions and queries to jenelbro119@hotmail,com
- «Jason Holder B3 photo
- Djokovic overcomes Federer, crowd for 10th major»
View the discussion thread.

Essay on Why Physical Education Is Important
Students are often asked to write an essay on Why Physical Education Is Important in their schools and colleges. And if you’re also looking for the same, we have created 100-word, 250-word, and 500-word essays on the topic.
Let’s take a look…
100 Words Essay on Why Physical Education Is Important
Keeps us healthy.
Physical education is important because it helps us stay healthy. When we move and exercise, our bodies become stronger. Our hearts pump better, and we can breathe easier. This means we get sick less often and can enjoy more activities without getting tired quickly.
Improves Learning
Exercise not only keeps our bodies healthy but also our minds. When we are active, our brain gets more oxygen. This makes it easier for us to think, remember things, and solve problems. So, physical education can help us do better in school.
Makes Us Happy
Being active also makes us feel happier. When we exercise, our body releases chemicals that make us feel good. This can help us feel less stressed and more relaxed. Physical education gives us a chance to have fun, make friends, and enjoy different sports and activities.
Teaches Teamwork
In physical education, we often play games and sports that require teamwork. This teaches us how to work with others, understand the importance of teamwork, and develop leadership skills. These are important skills that can help us in school, work, and other parts of our lives.
250 Words Essay on Why Physical Education Is Important
Physical education enhances physical health, pe fosters teamwork and social skills.
PE classes also provide opportunities for students to learn and practice teamwork, cooperation, and social skills. Team sports and group activities teach students the importance of working together towards a common goal, communicating effectively, and resolving conflicts peacefully. These skills are essential for success in both personal and professional life.
PE Boosts Cognitive Function
Studies have shown that regular physical activity can improve cognitive function, including memory, attention, and problem-solving skills. Exercise increases blood flow to the brain, which helps in the growth and development of brain cells. PE classes that involve physical activity and mental challenges, such as strategy games or obstacle courses, can enhance cognitive function even further.
PE Teaches Healthy Habits
PE classes help students develop healthy habits that they can carry throughout their lives. These habits include regular exercise, proper nutrition, and adequate sleep. By learning about the importance of these habits in PE class, students are more likely to adopt them and maintain a healthy lifestyle as they grow older.
PE Encourages Self-Confidence and Positive Body Image
PE classes provide a safe and supportive environment for students to explore their physical abilities and develop a positive body image. Through physical activity, students learn to appreciate their bodies for what they can do, rather than focusing on how they look. PE also helps students develop self-confidence by providing opportunities to achieve personal goals, overcome challenges, and work as part of a team.
500 Words Essay on Why Physical Education Is Important
Physical education improves overall health and fitness.
Physical education is essential for improving a person’s overall health and fitness. Regular physical activity can help to reduce the risk of chronic diseases such as obesity, heart disease, stroke, and type 2 diabetes. It can also help to improve muscle strength, flexibility, and cardiovascular fitness. Additionally, physical education can help to develop good habits and attitudes towards physical activity that can last a lifetime.
Physical Education Enhances Academic Performance
Physical education teaches important life skills.
Physical education can help students to develop important life skills such as teamwork, cooperation, and sportsmanship. These skills can be learned through a variety of physical activities, including team sports, individual sports, and fitness activities. Physical education can also help students to develop self-confidence, discipline, and perseverance. These skills can be valuable in all aspects of life.
Physical Education Encourages Healthy Lifestyles
Physical education can help to encourage students to adopt healthy lifestyles. By providing students with the opportunity to experience the benefits of physical activity, physical education can help to motivate them to continue participating in physical activity throughout their lives. Additionally, physical education can help students to learn about the importance of healthy eating and making healthy lifestyle choices.
If you’re looking for more, here are essays on other interesting topics:
Leave a Reply Cancel reply
Save my name, email, and website in this browser for the next time I comment.
Physical Education Schools
What is the impact of physical education on students’ well-being and academic success?
Decreasing time for quality phys-ed to allow more instructional time for core curricular subjects – including math, science, social studies and English – is counterproductive, given its positive benefits on health outcomes and school achievement.
by: Lee Schaefer , Derek Wasyliw
date: June 25, 2018
Download and print the Fact Sheet (232.30 kB / pdf)

Research confirms that healthier students make better learners. The term quality physical education is used to describe programs that are catered to a student’s age, skill level, culture and unique needs. They include 90 minutes of physical activity per week, fostering students’ well-being and improving their academic success. However, instructional time for quality phys-ed programs around the world are being decreased to prioritize other subject areas (especially math, science, social studies and English) in hopes to achieve higher academic achievement. However, several studies have identified a significant relationship between physical activity and academic achievement. Research also demonstrates that phys-ed does not have negative impacts on student success and that it offers the following physical, social, emotional and cognitive benefits:
Quality phys-ed helps students understand how exercise helps them to develop a healthy lifestyle, gain a variety of skills that help them to participate in a variety of physical activities and enjoy an active lifestyle.
Quality phys-ed provides students with the opportunity to socialize with others and learn different skills such as communication, tolerance, trust, empathy and respect for others. They also learn positive team skills including cooperation, leadership, cohesion and responsibility. Students who play sports or participate in other physical activities experience a variety of emotions and learn how to better cope in stressful, challenging or painful situations.
Quality phys-ed can be associated with improved mental health, since increased activity provides psychological benefits including reduced stress, anxiety and depression. It also helps students develop strategies to manage their emotions and increases their self-esteem.
Research tends to show that increased blood flow produced by physical activity may stimulate the brain and boost mental performance. Avoiding inactivity may also increase energy and concentration in the classroom.
Therefore, decreasing time for quality phys-ed to allow more instructional time for core curricular subjects – including math, science, social studies and English – is counterproductive, given its positive benefits on health outcomes and school achievement.
Additional Information Resources
PHE Canada (2018). Quality daily physical education . Retrieved from https://phecanada.ca/activate/qdpe
Ontario Ministry of Education. (2005). Healthy schools daily physical activity in schools grades 1 ‐ 3. Retrieved from http://www.edu.gov.on.ca/eng/teachers/dpa1-3.pdf
Ardoy, D. N., Fernández‐Rodríguez, J. M., Jiménez‐Pavón, D., Castillo, R., Ruiz, J. R., & Ortega, F. B. (2014). A Physical Education trial improves adolescents’ cognitive performance and academic achievement: The EDUFIT study. Scandinavian journal of medicine & science in sports , 24 (1).
Bailey, R., Armour, K., Kirk, D., Jess, M., Pickup, I., Sandford, R., & Education, B. P. (2009). The educational benefits claimed for physical education and school sport: An academic review. Research papers in education , 24 (1), 1-27.
Beane, J.A. (1990). Affect in the curriculum: Toward democracy, dignity, and diversity . Columbia: Teachers College Press.
Bedard, C., Bremer, E., Campbell, W., & Cairney, J. (2017). Evaluation of a direct-instruction intervention to improve movement and pre-literacy skills among young children: A within-subject repeated measures design. Frontiers in pediatrics , 5 , 298.
Hellison, D.R., N. Cutforth, J. Kallusky, T. Martinek, M. Parker, and J. Stiel. (2000). Youth development and physical activity: Linking universities and communities. Champaign, IL: Human Kinetics.
Ho, F. K. W., Louie, L. H. T., Wong, W. H. S., Chan, K. L., Tiwari, A., Chow, C. B., & Cheung, Y. F. (2017). A sports-based youth development program, teen mental health, and physical fitness: An RCT. Pediatrics , e20171543.
Keeley, T. J., & Fox, K. R. (2009). The impact of physical activity and fitness on academic achievement and cognitive performance in children. International Review of Sport and Exercise Psychology , 2 (2), 198-214.
Kohl III, H. W., & Cook, H. D. (Eds.). (2013). Educating the student body: Taking physical activity and physical education to school . National Academies Press.
Rasberry, C. N., Lee, S. M., Robin, L., Laris, B. A., Russell, L. A., Coyle, K. K., & Nihiser, A. J. (2011). The association between school-based physical activity, including physical education, and academic performance: a systematic review of the literature. Preventive medicine , 52 , S10-S20.
Sallis, J. F., McKenzie, T. L., Kolody, B., Lewis, M., Marshall, S., & Rosengard, P. (1999). Effects of health-related physical education on academic achievement: Project SPARK. Research quarterly for exercise and sport , 70 (2), 127-134.
Strong WB, Malina RM, Blimkie CJ, Daniels SR, Dishman RK, Gutin B, Hergenroeder AC, Must A, Nixon PA, Pivarnik JM, Rowland T, Trost S, & Trudeau F (2005). Evidence based physical activity for school-age youth. Journal of Pediatrics . 146(6):732–737.
Trudeau, F., & Shephard, R. J. (2008). Physical education, school physical activity, school sports and academic performance. International Journal of Behavioral Nutrition and Physical Activity , 5 (1), 10.
Beane, J. A. (1990). Affect in the curriculum: Toward democracy, dignity, and diversity . Columbia University, New York, NY: Teachers College Press.
Meet the Expert(s)
Lee schaefer.
Assistant Professor in the Kinesiology and Physical Education Department at McGill University
Lee Schaefer is an Assistant Professor in the Kinesiology and Physical Education Department at McGill University. His work is generally focused on teacher education and teacher knowle...
Derek Wasyliw
Master’s student in the Kinesiology and Physical Education Graduate Program at McGill University
Derek Wasyliw is a second-year Master’s student in the Kinesiology and Physical Education Graduate Program at McGill University. He is the proud recipient of the 2017-2018 SSHRC Jo...

IDEAS TO SUPPORT EDUCATORS : Subscribe to the EdCan e-Newsletter
Quality research, reports, and professional learning opportunities.
Language Preference Langue de correspondance English Français English Français
71 Physical Education Essay Topic Ideas & Examples
🏆 best physical education topic ideas & essay examples, 🔎 interesting topics to write about physical education, 📑 good research topics about physical education.
- Keeping Physical Education in Schools Apart from participating in the physical education programs, the students need to be taught on the importance of the various exercises so that they inculcate the culture of physical fitness into their life-time fitness programs.
- The Nature and Values of Physical Education In the past, physical education was considered to consist of only physical and practical activities, however, the recent research has justified that physical education can be included in the curriculum on the basis of scientific […]
- Adaptive Physical Education The value of the brochure developed for the informational purpose is attributed to the need to communicate the importance of APE and point out the value that it could bring to children with special needs.
- Physical Education and Its Benefits Schools in particular know the benefits of physical education in a student’s life and should be able to fight for the children’s rights.
- Race and Gender in Physical Education and Sports These factors create the diversity of cultures and nations, and inclusiveness, giving access to the best talents and disclosing the individual’s potential, abilities, and strengths.
- The Usefulness of Physical Education in Modern Education Varied criticism adds to the debate on the usefulness of PE in modern education and the need to change current approaches. This indicates the need to focus the debate on the meaning of PE to […]
- Physical Education within Elementary Schools One of the benefits of the physical education is the level of physical fitness that it induces to the students. The manner in which these students are introduced to physical education and the way that […]
- Effects of Physical Education on Brain These neurons are usually created in a place called the hippocampus, which happens to be the section of the brain involved in learning and storage of memory.
- Health Teaching and Physical Education Lesson Plan Students will be able to dribble a ball with a hand paying attention to such principles as dribbling on the side, waist-high, pushing the ball down, and eyes lookup.
- Physical Education Curriculum Physical education has significantly contributed towards the realization of the school philosophy as it helps in the development of the physical aspects of the students.
- Physical Education: Effect of Phototherapy Therefore, it is evident that the intensity of an exercise directly influences one’s heart rate, breathing rate, skin coloration, sweating, and recovery.
- Bodies in Physical Education The purpose of this study is to investigate how students view the construction of their bodies in relation to physical education and how students’ meanings of their bodies affect their participation or resistance to physical […]
- Effectiveness of Physical Education Provisions in the UK School The vital need for health promotion, especially in terms of secondary education has been highlighted by the science of epidemiology the study of factors that influence the health and illnesses of people.
- British Development of Sport and Physical Education in the Last 25 Years Sport England wishes to increase participation in sports through community sports activities, sporting completions providing and training coaches and officiators, and closely working with the Youth Sport Trust and UK Sports formed in 1996 to […]
- Physical Education: Personal Physical Exercise Plan Given the necessity of taking fluids, it is good to identify and avail the same before starting a physical exercise session.
- Increase of Physical Education Classes Children are the future of any nation, and their health and well-being are the essential preconditions for the successful development of the United States.
- Physical Educators Attitude to Special Needs Children Sue Combs, together with her colleagues from the University of North Carolina, investigated the attitudes of the physical education teachers towards the inclusion of children with special needs in their lessons.
- Should Public Schools Be Required to Restore Physical Education Classes to the Curriculum? The occurrence of obesity prevalence in children, in the U S, can be associated with the removal of physical education courses in public school curriculum.
- Elementary School Curriculum and Physical Education
- Should Physical Education Be a Required Class in College?
- Physical Education Class: The Perfect Place to Be Bullied
- Pros and Cons of Physical Education
- How Physical Education Should Be Taught
- Physical Education for Elementary School Students
- Weight-Related Barriers for Overweight Students in an Elementary Physical Education Classroom
- Physical Education Lesson Plan and Activity Ideas
- Motivation, Discipline, and Academic Performance in Physical Education
- Adaptive Physical Education for Students With Special Needs
- Physical Education Should Not Be Mandated
- How Technology Enhances the Physical Education Curriculum
- Physical Education: Standards, Cooperative Skills, and Learning Theories
- Physical Education’s Contribution to Public Health
- Physical Education Importance for Child Development
- Reasons to Keep Physical Education in the National Curriculum
- Ethical Relativism and Its Impact on Physical Education
- Inclusive School Physical Education and Physical Activity
- History and Benefits of Physical Education: Why I Want to Be a P.E. Teacher
- Physical Education Beyond the Middle School
- The Importance of Physical Education in Childhood Obesity
- Physical Activity Promotion and School Physical Education
- Implementing the TARGET Model in Physical Education: Effects on Perceived Psychobiosocial and Motivational States in Girls
- Teaching the Nuts and Bolts of Physical Education
- Health-Related Intensity Profiles for Physical Education Classes
- Anticipated Benefits From a Basic College Physical Education Activity Course
- Physical Education Should Be Graded on Effort, Not Ability
- Motivation and Intention to Be Physically Active in Physical Education Students
- Personal Development, Health, and Physical Education
- Why Physical Education Should Be Included in the School Curriculum
- Attitude and Teacher’s Qualification as Factors Affecting Students’ Participation in Physical Education Activities
- Burnout in Physical Education Teachers
- What Benefits Physical Activity Has on Academic Performance
- SPARK Physical Education Curriculum Program
- Changing the National Curriculum for Physical Education
- Physical Education: Official School Policy
- How Physical Education Helps to Develop Your Personality
- Early Childhood Development: Physical Education Program Effects
- Fun Physical Education Games for High School Students
- How Extracurricular Sports Should Satisfy State Physical Education Requirements
- One’s Readiness to Self-Development Through Physical Education
- Would More Physical Education Reduce Obesity in the Youths?
- Goal-Directed Physical Education for Learners With Disabilities
- Health and Physical Education: Volleyball
- Managing the Physical Education Classroom
- Strategies to Accommodate Autism Spectrum Disorder Students in General Physical Education
- Physical Education vs. School Sports: What’s the Difference?
- The Impact of School Budgetary Cuts on Physical Education
- Teaching Health and Physical Education in Australian Schools
- Positive Reinforcement Techniques in Physical Education
- Child Development Research Ideas
- Academic Performance Topics
- Football Topics
- Asthma Paper Topics
- Lifespan Development Essay Titles
- Obesity Ideas
- Soccer Research Topics
- Adolescence Questions
- Chicago (A-D)
- Chicago (N-B)
IvyPanda. (2023, November 9). 71 Physical Education Essay Topic Ideas & Examples. https://ivypanda.com/essays/topic/physical-education-essay-topics/
"71 Physical Education Essay Topic Ideas & Examples." IvyPanda , 9 Nov. 2023, ivypanda.com/essays/topic/physical-education-essay-topics/.
IvyPanda . (2023) '71 Physical Education Essay Topic Ideas & Examples'. 9 November.
IvyPanda . 2023. "71 Physical Education Essay Topic Ideas & Examples." November 9, 2023. https://ivypanda.com/essays/topic/physical-education-essay-topics/.
1. IvyPanda . "71 Physical Education Essay Topic Ideas & Examples." November 9, 2023. https://ivypanda.com/essays/topic/physical-education-essay-topics/.
Bibliography
IvyPanda . "71 Physical Education Essay Topic Ideas & Examples." November 9, 2023. https://ivypanda.com/essays/topic/physical-education-essay-topics/.
Warning: The NCBI web site requires JavaScript to function. more...

An official website of the United States government
The .gov means it's official. Federal government websites often end in .gov or .mil. Before sharing sensitive information, make sure you're on a federal government site.
The site is secure. The https:// ensures that you are connecting to the official website and that any information you provide is encrypted and transmitted securely.
- Publications
- Account settings
- Browse Titles
NCBI Bookshelf. A service of the National Library of Medicine, National Institutes of Health.
Committee on Physical Activity and Physical Education in the School Environment; Food and Nutrition Board; Institute of Medicine; Kohl HW III, Cook HD, editors. Educating the Student Body: Taking Physical Activity and Physical Education to School. Washington (DC): National Academies Press (US); 2013 Oct 30.

Educating the Student Body: Taking Physical Activity and Physical Education to School.
- Hardcopy Version at National Academies Press
4 Physical Activity, Fitness, and Physical Education: Effects on Academic Performance
Key messages.
- Evidence suggests that increasing physical activity and physical fitness may improve academic performance and that time in the school day dedicated to recess, physical education class, and physical activity in the classroom may also facilitate academic performance.
- Available evidence suggests that mathematics and reading are the academic topics that are most influenced by physical activity. These topics depend on efficient and effective executive function, which has been linked to physical activity and physical fitness.
- Executive function and brain health underlie academic performance. Basic cognitive functions related to attention and memory facilitate learning, and these functions are enhanced by physical activity and higher aerobic fitness.
- Single sessions of and long-term participation in physical activity improve cognitive performance and brain health. Children who participate in vigorous- or moderate-intensity physical activity benefit the most.
- Given the importance of time on task to learning, students should be provided with frequent physical activity breaks that are developmentally appropriate.
- Although presently understudied, physically active lessons offered in the classroom may increase time on task and attention to task in the classroom setting.
Although academic performance stems from a complex interaction between intellect and contextual variables, health is a vital moderating factor in a child's ability to learn. The idea that healthy children learn better is empirically supported and well accepted ( Basch, 2010 ), and multiple studies have confirmed that health benefits are associated with physical activity, including cardiovascular and muscular fitness, bone health, psychosocial outcomes, and cognitive and brain health ( Strong et al., 2005 ; see Chapter 3 ). The relationship of physical activity and physical fitness to cognitive and brain health and to academic performance is the subject of this chapter.
Given that the brain is responsible for both mental processes and physical actions of the human body, brain health is important across the life span. In adults, brain health, representing absence of disease and optimal structure and function, is measured in terms of quality of life and effective functioning in activities of daily living. In children, brain health can be measured in terms of successful development of attention, on-task behavior, memory, and academic performance in an educational setting. This chapter reviews the findings of recent research regarding the contribution of engagement in physical activity and the attainment of a health-enhancing level of physical fitness to cognitive and brain health in children. Correlational research examining the relationship among academic performance, physical fitness, and physical activity also is described. Because research in older adults has served as a model for understanding the effects of physical activity and fitness on the developing brain during childhood, the adult research is briefly discussed. The short- and long-term cognitive benefits of both a single session of and regular participation in physical activity are summarized.
Before outlining the health benefits of physical activity and fitness, it is important to note that many factors influence academic performance. Among these are socioeconomic status ( Sirin, 2005 ), parental involvement ( Fan and Chen, 2001 ), and a host of other demographic factors. A valuable predictor of student academic performance is a parent having clear expectations for the child's academic success. Attendance is another factor confirmed as having a significant impact on academic performance ( Stanca, 2006 ; Baxter et al., 2011 ). Because children must be present to learn the desired content, attendance should be measured in considering factors related to academic performance.
- PHYSICAL FITNESS AND PHYSICAL ACTIVITY: RELATION TO ACADEMIC PERFORMANCE
State-mandated academic achievement testing has had the unintended consequence of reducing opportunities for children to be physically active during the school day and beyond. In addition to a general shifting of time in school away from physical education to allow for more time on academic subjects, some children are withheld from physical education classes or recess to participate in remedial or enriched learning experiences designed to increase academic performance ( Pellegrini and Bohn, 2005 ; see Chapter 5 ). Yet little evidence supports the notion that more time allocated to subject matter will translate into better test scores. Indeed, 11 of 14 correlational studies of physical activity during the school day demonstrate a positive relationship to academic performance ( Rasberry et al., 2011 ). Overall, a rapidly growing body of work suggests that time spent engaged in physical activity is related not only to a healthier body but also to a healthier mind ( Hillman et al., 2008 ).
Children respond faster and with greater accuracy to a variety of cognitive tasks after participating in a session of physical activity ( Tomporowski, 2003 ; Budde et al., 2008 ; Hillman et al., 2009 ; Pesce et al., 2009 ; Ellemberg and St-Louis-Deschênes, 2010 ). A single bout of moderate-intensity physical activity has been found to increase neural and behavioral concomitants associated with the allocation of attention to a specific cognitive task ( Hillman et al., 2009 ; Pontifex et al., 2012 ). And when children who participated in 30 minutes of aerobic physical activity were compared with children who watched television for the same amount of time, the former children cognitively outperformed the latter ( Ellemberg and St-Louis-Desêhenes, 2010 ). Visual task switching data among 69 overweight and inactive children did not show differences between cognitive performance after treadmill walking and sitting ( Tomporowski et al., 2008b ).
When physical activity is used as a break from academic learning time, postengagement effects include better attention ( Grieco et al., 2009 ; Bartholomew and Jowers, 2011 ), increased on-task behaviors ( Mahar et al., 2006 ), and improved academic performance ( Donnelly and Lambourne, 2011 ). Comparisons between 1st-grade students housed in a classroom with stand-sit desks where the child could stand at his/her discretion and in classrooms containing traditional furniture showed that the former children were highly likely to stand, thus expending significantly more energy than those who were seated ( Benden et al., 2011 ). More important, teachers can offer physical activity breaks as part of a supplemental curriculum or simply as a way to reset student attention during a lesson ( Kibbe et al., 2011 ; see Chapter 6 ) and when provided with minimal training can efficaciously produce vigorous or moderate energy expenditure in students ( Stewart et al., 2004 ). Further, after-school physical activity programs have demonstrated the ability to improve cardiovascular endurance, and this increase in aerobic fitness has been shown to mediate improvements in academic performance ( Fredericks et al., 2006 ), as well as the allocation of neural resources underlying performance on a working memory task ( Kamijo et al., 2011 ).
Over the past three decades, several reviews and meta-analyses have described the relationship among physical fitness, physical activity, and cognition (broadly defined as all mental processes). The majority of these reviews have focused on the relationship between academic performance and physical fitness—a physiological trait commonly defined in terms of cardiorespiratory capacity (e.g., maximal oxygen consumption; see Chapter 3 ). More recently, reviews have attempted to describe the effects of an acute or single bout of physical activity, as a behavior, on academic performance. These reviews have focused on brain health in older adults ( Colcombe and Kramer, 2003 ), as well as the effects of acute physical activity on cognition in adults ( Tomporowski, 2003 ). Some have considered age as part of the analysis ( Etnier et al., 1997 , 2006 ). Reviews focusing on research conducted in children ( Sibley and Etnier, 2003 ) have examined the relationship among physical activity, participation in sports, and academic performance ( Trudeau and Shephard, 2008 , 2010 ; Singh et al., 2012 ); physical activity and mental and cognitive health ( Biddle and Asare, 2011 ); and physical activity, nutrition, and academic performance ( Burkhalter and Hillman, 2011 ). The findings of most of these reviews align with the conclusions presented in a meta-analytic review conducted by Fedewa and Ahn (2011) . The studies reviewed by Fedewa and Ahn include experimental/quasi-experimental as well as cross-sectional and correlational designs, with the experimental designs yielding the highest effect sizes. The strongest relationships were found between aerobic fitness and achievement in mathematics, followed by IQ and reading performance. The range of cognitive performance measures, participant characteristics, and types of research design all mediated the relationship among physical activity, fitness, and academic performance. With regard to physical activity interventions, which were carried out both within and beyond the school day, those involving small groups of peers (around 10 youth of a similar age) were associated with the greatest gains in academic performance.
The number of peer-reviewed publications on this topic is growing exponentially. Further evidence of the growth of this line of inquiry is its increased global presence. Positive relationships among physical activity, physical fitness, and academic performance have been found among students from the Netherlands ( Singh et al., 2012 ) and Taiwan ( Chih and Chen, 2011 ). Broadly speaking, however, many of these studies show small to moderate effects and suffer from poor research designs ( Biddle and Asare, 2011 ; Singh et al., 2012 ).
Basch (2010) conducted a comprehensive review of how children's health and health disparities influence academic performance and learning. The author's report draws on empirical evidence suggesting that education reform will be ineffective unless children's health is made a priority. Basch concludes that schools may be the only place where health inequities can be addressed and that, if children's basic health needs are not met, they will struggle to learn regardless of the effectiveness of the instructional materials used. More recently, Efrat (2011) conducted a review of physical activity, fitness, and academic performance to examine the achievement gap. He discovered that only seven studies had included socioeconomic status as a variable, despite its known relationship to education ( Sirin, 2005 ).
Physical Fitness as a Learning Outcome of Physical Education and Its Relation to Academic Performance
Achieving and maintaining a healthy level of aerobic fitness, as defined using criterion-referenced standards from the National Health and Nutrition Examination Survey (NHANES; Welk et al., 2011 ), is a desired learning outcome of physical education programming. Regular participation in physical activity also is a national learning standard for physical education, a standard intended to facilitate the establishment of habitual and meaningful engagement in physical activity ( NASPE, 2004 ). Yet although physical fitness and participation in physical activity are established as learning outcomes in all 50 states, there is little evidence to suggest that children actually achieve and maintain these standards (see Chapter 2 ).
Statewide and national datasets containing data on youth physical fitness and academic performance have increased access to student-level data on this subject ( Grissom, 2005 ; Cottrell et al., 2007 ; Carlson et al., 2008 ; Chomitz et al., 2008 ; Wittberg et al., 2010 ; Van Dusen et al., 2011 ). Early research in South Australia focused on quantifying the benefits of physical activity and physical education during the school day; the benefits noted included increased physical fitness, decreased body fat, and reduced risk for cardiovascular disease ( Dwyer et al., 1979 , 1983 ). Even today, Dwyer and colleagues are among the few scholars who regularly include in their research measures of physical activity intensity in the school environment, which is believed to be a key reason why they are able to report differentiated effects of different intensities. A longitudinal study in Trois-Rivières, Québec, Canada, tracked how the academic performance of children from grades 1 through 6 was related to student health, motor skills, and time spent in physical education. The researchers concluded that additional time dedicated to physical education did not inhibit academic performance ( Shephard et al., 1984 ; Shephard, 1986 ; Trudeau and Shephard, 2008 ).
Longitudinal follow-up investigating the long-term benefits of enhanced physical education experiences is encouraging but largely inconclusive. In a study examining the effects of daily physical education during elementary school on physical activity during adulthood, 720 men and women completed the Québec Health Survey ( Trudeau et al., 1999 ). Findings suggest that physical education was associated with physical activity in later life for females but not males ( Trudeau et al., 1999 ); most of the associations were significant but weak ( Trudeau et al., 2004 ). Adult body mass index (BMI) at age 34 was related to childhood BMI at ages 10-12 in females but not males ( Trudeau et al., 2001 ). Longitudinal studies such as those conducted in Sweden and Finland also suggest that physical education experiences may be related to adult engagement in physical activity ( Glenmark, 1994 ; Telama et al., 1997 ). From an academic performance perspective, longitudinal data on men who enlisted for military service imply that cardiovascular fitness at age 18 predicted cognitive performance in later life (Aberg et al., 2009), thereby supporting the idea of offering physical education and physical activity opportunities well into emerging adulthood through secondary and postsecondary education.
Castelli and colleagues (2007) investigated younger children (in 3rd and 5th grades) and the differential contributions of the various subcomponents of the Fitnessgram ® . Specifically, they examined the individual contributions of aerobic capacity, muscle strength, muscle flexibility, and body composition to performance in mathematics and reading on the Illinois Standardized Achievement Test among a sample of 259 children. Their findings corroborate those of the California Department of Education ( Grissom, 2005 ), indicating a general relationship between fitness and achievement test performance. When the individual components of the Fitnessgram were decomposed, the researchers determined that only aerobic capacity was related to test performance. Muscle strength and flexibility showed no relationship, while an inverse association of BMI with test performance was observed, such that higher BMI was associated with lower test performance. Although Baxter and colleagues (2011) confirmed the importance of attending school in relation to academic performance through the use of 4th-grade student recall, correlations with BMI were not significant.
State-mandated implementation of the coordinated school health model requires all schools in Texas to conduct annual fitness testing using the Fitnessgram among students in grades 3-12. In a special issue of Research Quarterly for Exercise and Sport (2010), multiple articles describe the current state of physical fitness among children in Texas; confirm the associations among school performance levels, academic achievement, and physical fitness ( Welk et al., 2010 ; Zhu et al., 2010 ); and demonstrate the ability of qualified physical education teachers to administer physical fitness tests ( Zhu et al., 2010 ). Also using data from Texas schools, Van Dusen and colleagues (2011) found that cardiovascular fitness had the strongest association with academic performance, particularly in mathematics over reading. Unlike previous research, which demonstrated a steady decline in fitness by developmental stage ( Duncan et al., 2007 ), this study found that cardiovascular fitness did decrease but not significantly ( Van Dusen et al., 2011 ). Aerobic fitness, then, may be important to academic performance, as there may be a dose-response relationship ( Van Dusen et al., 2011 ).
Using a large sample of students in grades 4-8, Chomitz and colleagues (2008) found that the likelihood of passing both mathematics and English achievement tests increased with the number of fitness tests passed during physical education class, and the odds of passing the mathematics achievement tests were inversely related to higher body weight. Similar to the findings of Castelli and colleagues (2007) , socioeconomic status and demographic factors explained little of the relationship between aerobic fitness and academic performance; however, socioeconomic status may be an explanatory variable for students of low fitness ( London and Castrechini, 2011 ).
In sum, numerous cross-sectional and correlational studies demonstrate small-to-moderate positive or null associations between physical fitness ( Grissom, 2005 ; Cottrell et al., 2007 ; Edwards et al., 2009; Eveland-Sayers et al., 2009 ; Cooper et al., 2010 ; Welk et al., 2010 ; Wittberg et al., 2010 ; Zhu et al., 2010 ; Van Dusen et al., 2011 ), particularly aerobic fitness, and academic performance ( Castelli et al, 2007 ; Chomitz et al., 2008 ; Roberts et al., 2010 ; Welk et al., 2010 ; Chih and Chen, 2011 ; London and Castrechini, 2011 ; Van Dusen et al., 2011 ). Moreover, the findings may support a dose-response association, suggesting that the more components of physical fitness (e.g., cardiovascular endurance, strength, muscle endurance) considered acceptable for the specific age and gender that are present, the greater the likelihood of successful academic performance. From a public health and policy standpoint, the conclusions these findings support are limited by few causal inferences, a lack of data confirmation, and inadequate reliability because the data were often collected by nonresearchers or through self-report methods. It may also be noted that this research includes no known longitudinal studies and few randomized controlled trials (examples are included later in this chapter in the discussion of the developing brain).
Physical Activity, Physical Education, and Academic Performance
In contrast with the correlational data presented above for physical fitness, more information is needed on the direct effects of participation in physical activity programming and physical education classes on academic performance.
In a meta-analysis, Sibley and Etnier (2003) found a positive relationship between physical activity and cognition in school-age youth (aged 4-18), suggesting that physical activity, as well as physical fitness, may be related to cognitive outcomes during development. Participation in physical activity was related to cognitive performance in eight measurement categories (perceptual skills, IQ, achievement, verbal tests, mathematics tests, memory, developmental level/academic readiness, and “other”), with results indicating a beneficial relationship of physical activity to all cognitive outcomes except memory ( Sibley and Etnier, 2003 ). Since that meta-analysis, however, several papers have reported robust relationships between aerobic fitness and different aspects of memory in children (e.g., Chaddock et al., 2010a , 2011 ; Kamijo et al., 2011 ; Monti et al., 2012 ). Regardless, the comprehensive review of Sibley and Etnier (2003) was important because it helped bring attention to an emerging literature suggesting that physical activity may benefit cognitive development even as it also demonstrated the need for further study to better understand the multifaceted relationship between physical activity and cognitive and brain health.
The regular engagement in physical activity achieved during physical education programming can also be related to academic performance, especially when the class is taught by a physical education teacher. The Sports, Play, and Active Recreation for Kids (SPARK) study examined the effects of a 2-year health-related physical education program on academic performance in children ( Sallis et al., 1999 ). In an experimental design, seven elementary schools were randomly assigned to one of three conditions: (1) a specialist condition in which certified physical education teachers delivered the SPARK curriculum, (2) a trained-teacher condition in which classroom teachers implemented the curriculum, and (3) a control condition in which classroom teachers implemented the local physical education curriculum. No significant differences by condition were found for mathematics testing; however, reading scores were significantly higher in the specialist condition relative to the control condition ( Sallis et al., 1999 ), while language scores were significantly lower in the specialist condition than in the other two conditions. The authors conclude that spending time in physical education with a specialist did not have a negative effect on academic performance. Shortcomings of this research include the amount of data loss from pre- to posttest, the use of results of 2nd-grade testing that exceeded the national average in performance as baseline data, and the use of norm-referenced rather than criterion-based testing.
In seminal research conducted by Gabbard and Barton (1979) , six different conditions of physical activity (no activity; 20, 30, 40, and 50 minutes; and posttest no activity) were completed by 106 2nd graders during physical education. Each physical activity session was followed by 5 minutes of rest and the completion of 36 math problems. The authors found a potential threshold effect whereby only the 50-minute condition improved mathematical performance, with no differences by gender.
A longitudinal study of the kindergarten class of 1998–1999, using data from the Early Childhood Longitudinal Study, investigated the association between enrollment in physical education and academic achievement ( Carlson et al., 2008 ). Higher amounts of physical education were correlated with better academic performance in mathematics among females, but this finding did not hold true for males.
Ahamed and colleagues (2007) found in a cluster randomized trial that, after 16 months of a classroom-based physical activity intervention, there was no significant difference between the treatment and control groups in performance on the standardized Cognitive Abilities Test, Third Edition (CAT-3). Others have found, however, that coordinative exercise ( Budde et al., 2008 ) or bouts of vigorous physical activity during free time ( Coe et al., 2006 ) contribute to higher levels of academic performance. Specifically, Coe and colleagues examined the association of enrollment in physical education and self-reported vigorous- or moderate-intensity physical activity outside school with performance in core academic courses and on the Terra Nova Standardized Achievement Test among more than 200 6th-grade students. Their findings indicate that academic performance was unaffected by enrollment in physical education classes, which were found to average only 19 minutes of vigorous- or moderate-intensity physical activity. When time spent engaged in vigorous- or moderate-intensity physical activity outside of school was considered, however, a significant positive relation to academic performance emerged, with more time engaged in vigorous- or moderate-intensity physical activity being related to better grades but not test scores ( Coe et al., 2006 ).
Studies of participation in sports and academic achievement have found positive associations ( Mechanic and Hansell, 1987 ; Dexter, 1999 ; Crosnoe, 2002 ; Eitle and Eitle, 2002 ; Stephens and Schaben, 2002 ; Eitle, 2005 ; Miller et al., 2005 ; Fox et al., 2010 ; Ruiz et al., 2010 ); higher grade point averages (GPAs) in season than out of season ( Silliker and Quirk, 1997 ); a negative association between cheerleading and science performance ( Hanson and Kraus, 1998 ); and weak and negative associations between the amount of time spent participating in sports and performance in English-language class among 13-, 14-, and 16-year-old students ( Daley and Ryan, 2000 ). Other studies, however, have found no association between participation in sports and academic performance ( Fisher et al., 1996 ). The findings of these studies need to be interpreted with caution as many of their designs failed to account for the level of participation by individuals in the sport (e.g., amount of playing time, type and intensity of physical activity engagement by sport). Further, it is unclear whether policies required students to have higher GPAs to be eligible for participation. Offering sports opportunities is well justified regardless of the cognitive benefits, however, given that adolescents may be less likely to engage in risky behaviors when involved in sports or other extracurricular activities ( Page et al., 1998 ; Elder et al., 2000 ; Taliaferro et al., 2010 ), that participation in sports increases physical fitness, and that affiliation with sports enhances school connectedness.
Although a consensus on the relationship of physical activity to academic achievement has not been reached, the vast majority of available evidence suggests the relationship is either positive or neutral. The meta-analytic review by Fedewa and Ahn (2011) suggests that interventions entailing aerobic physical activity have the greatest impact on academic performance; however, all types of physical activity, except those involving flexibility alone, contribute to enhanced academic performance, as do interventions that use small groups (about 10 students) rather than individuals or large groups. Regardless of the strength of the findings, the literature indicates that time spent engaged in physical activity is beneficial to children because it has not been found to detract from academic performance, and in fact can improve overall health and function ( Sallis et al., 1999 ; Hillman et al., 2008 ; Tomporowski et al., 2008a ; Trudeau and Shephard, 2008 ; Rasberry et al., 2011 ).
Single Bouts of Physical Activity
Beyond formal physical education, evidence suggests that multi-component approaches are a viable means of providing physical activity opportunities for children across the school curriculum (see also Chapter 6 ). Although health-related fitness lessons taught by certified physical education teachers result in greater student fitness gains relative to such lessons taught by other teachers ( Sallis et al., 1999 ), non-physical education teachers are capable of providing opportunities to be physically active within the classroom ( Kibbe et al., 2011 ). Single sessions or bouts of physical activity have independent merit, offering immediate benefits that can enhance the learning experience. Studies have found that single bouts of physical activity result in improved attention ( Hillman et al., 2003 , 2009 ; Pontifex et al., 2012 ), better working memory ( Pontifex et al., 2009 ), and increased academic learning time and reduced off-task behaviors ( Mahar et al., 2006 ; Bartholomew and Jowers, 2011 ). Yet single bouts of physical activity have differential effects, as very vigorous exercise has been associated with cognitive fatigue and even cognitive decline in adults ( Tomporowski, 2003 ). As seen in Figure 4-1 , high levels of effort, arousal, or activation can influence perception, decision making, response preparation, and actual response. For discussion of the underlying constructs and differential effects of single bouts of physical activity on cognitive performance, see Tomporowski (2003) .
Information processing: Diagram of a simplified version of Sanders's (1983) cognitive-energetic model of human information processing (adapted from Jones and Hardy, 1989). SOURCE: Tomporowski, 2003. Reprinted with permission.
For children, classrooms are busy places where they must distinguish relevant information from distractions that emerge from many different sources occurring simultaneously. A student must listen to the teacher, adhere to classroom procedures, focus on a specific task, hold and retain information, and make connections between novel information and previous experiences. Hillman and colleagues (2009) demonstrated that a single bout of moderate-intensity walking (60 percent of maximum heart rate) resulted in significant improvements in performance on a task requiring attentional inhibition (e.g., the ability to focus on a single task). These findings were accompanied by changes in neuroelectric measures underlying the allocation of attention (see Figure 4-2 ) and significant improvements on the reading subtest of the Wide Range Achievement Test. No such effects were observed following a similar duration of quiet rest. These findings were later replicated and extended to demonstrate benefits for both mathematics and reading performance in healthy children and those diagnosed with attention deficit hyperactivity disorder ( Pontifex et al., 2013 ). Further replications of these findings demonstrated that a single bout of moderate-intensity exercise using a treadmill improved performance on a task of attention and inhibition, but similar benefits were not derived from moderate-intensity exercise that involved exergaming ( O'Leary et al., 2011 ). It was also found that such benefits were derived following cessation of, but not during, the bout of exercise ( Drollette et al., 2012 ). The applications of such empirical findings within the school setting remain unclear.
Effects of a single session of exercise in preadolescent children. SOURCE: Hillman et al., 2009. Reprinted with permission.
A randomized controlled trial entitled Physical Activity Across the Curriculum (PAAC) used cluster randomization among 24 schools to examine the effects of physically active classroom lessons on BMI and academic achievement ( Donnelly et al., 2009 ). The academically oriented physical activities were intended to be of vigorous or moderate intensity (3–6 metabolic equivalents [METs]) and to last approximately 10 minutes and were specifically designed to supplement content in mathematics, language arts, geography, history, spelling, science, and health. The study followed 665 boys and 677 girls for 3 years as they rose from 2nd or 3rd to 4th or 5th grades. Changes in academic achievement, fitness, and blood screening were considered secondary outcomes. During a 3-year period, students who engaged in physically active lessons, on average, improved their academic achievement by 6 percent, while the control groups exhibited a 1 percent decrease. In students who experienced at least 75 minutes of PAAC lessons per week, BMI remained stable (see Figure 4-3 ).
Change in academic scores from baseline after physically active classroom lessons in elementary schools in northeast Kansas (2003–2006). NOTE: All differences between the Physical Activity Across the Curriculum (PAAC) group ( N = 117) and control (more...)
It is important to note that cognitive tasks completed before, during, and after physical activity show varying effects, but the effects were always positive compared with sedentary behavior. In a study carried out by Drollette and colleagues (2012) , 36 preadolescent children completed two cognitive tasks—a flanker task to assess attention and inhibition and a spatial nback task to assess working memory—before, during, and after seated rest and treadmill walking conditions. The children sat or walked on different days for an average of 19 minutes. The results suggest that the physical activity enhanced cognitive performance for the attention task but not for the task requiring working memory. Accordingly, although more research is needed, the authors suggest that the acute effects of exercise may be selective to certain cognitive processes (i.e., attentional inhibition) while unrelated to others (e.g., working memory). Indeed, data collected using a task-switching paradigm (i.e., a task designed to assess multitasking and requiring the scheduling of attention to multiple aspects of the environment) among 69 overweight and inactive children did not show differences in cognitive performance following acute bouts of treadmill walking or sitting ( Tomporowski et al., 2008b ). Thus, findings to date indicate a robust relationship of acute exercise to transient improvements in attention but appear inconsistent for other aspects of cognition.
Academic Learning Time and On- and Off-Task Behaviors
Excessive time on task, inattention to task, off-task behavior, and delinquency are important considerations in the learning environment given the importance of academic learning time to academic performance. These behaviors are observable and of concern to teachers as they detract from the learning environment. Systematic observation by trained observers may yield important insight regarding the effects of short physical activity breaks on these behaviors. Indeed, systematic observations of student behavior have been used as an alternative means of measuring academic performance ( Mahar et al., 2006 ; Grieco et al., 2009 ).
After the development of classroom-based physical activities, called Energizers, teachers were trained in how to implement such activities in their lessons at least twice per week ( Mahar et al., 2006 ). Measurements of baseline physical activity and on-task behaviors were collected in two 3rd-grade and two 4th-grade classes, using pedometers and direct observation. The intervention included 243 students, while 108 served as controls by not engaging in the activities. A subgroup of 62 3rd and 4th graders was observed for on-task behavior in the classroom following the physical activity. Children who participated in Energizers took more steps during the school day than those who did not; they also increased their on-task behaviors by more than 20 percent over baseline measures.
A systematic review of a similar in-class, academically oriented, physical activity plan—Take 10!—was conducted to identify the effects of its implementation after it had been in use for 10 years ( Kibbe et al., 2011 ). The findings suggest that children who experienced Take 10! in the classroom engaged in moderate to vigorous physical activity (6.16 to 6.42 METs) and had lower BMIs than those who did not. Further, children in the Take 10! classrooms had better fluid intelligence ( Reed et al., 2010 ) and higher academic achievement scores ( Donnelly et al., 2009 ).
Some have expressed concern that introducing physical activity into the classroom setting may be distracting to students. Yet in one study it was sedentary students who demonstrated a decrease in time on task, while active students returned to the same level of on-task behavior after an active learning task ( Grieco et al., 2009 ). Among the 97 3rd-grade students in this study, a small but nonsignificant increase in on-task behaviors was seen immediately following these active lessons. Additionally, these improvements were not mediated by BMI.
In sum, although presently understudied, physically active lessons may increase time on task and attention to task in the classroom setting. Given the complexity of the typical classroom, the strategy of including content-specific lessons that incorporate physical activity may be justified.
It is recommended that every child have 20 minutes of recess each day and that this time be outdoors whenever possible, in a safe activity ( NASPE, 2006 ). Consistent engagement in recess can help students refine social skills, learn social mediation skills surrounding fair play, obtain additional minutes of vigorous- or moderate-intensity physical activity that contribute toward the recommend 60 minutes or more per day, and have an opportunity to express their imagination through free play ( Pellegrini and Bohn, 2005 ; see also Chapter 6 ). When children participate in recess before lunch, additional benefits accrue, such as less food waste, increased incidence of appropriate behavior in the cafeteria during lunch, and greater student readiness to learn upon returning to the classroom after lunch ( Getlinger et al., 1996 ; Wechsler et al., 2001 ).
To examine the effects of engagement in physical activity during recess on classroom behavior, Barros and colleagues (2009) examined data from the Early Childhood Longitudinal Study on 10,000 8- to 9-year-old children. Teachers provided the number of minutes of recess as well as a ranking of classroom behavior (ranging from “misbehaves frequently” to “behaves exceptionally well”). Results indicate that children who had at least 15 minutes of recess were more likely to exhibit appropriate behavior in the classroom ( Barros et al., 2009 ). In another study, 43 4th-grade students were randomly assigned to 1 or no days of recess to examine the effects on classroom behavior ( Jarrett et al., 1998 ). The researchers concluded that on-task behavior was better among the children who had recess. A moderate effect size (= 0.51) was observed. In a series of studies examining kindergartners' attention to task following a 20-minute recess, increased time on task was observed during learning centers and story reading ( Pellegrini et al., 1995 ). Despite these positive findings centered on improved attention, it is important to note that few of these studies actually measured the intensity of the physical activity during recess.
From a slightly different perspective, survey data from 547 Virginia elementary school principals suggest that time dedicated to student participation in physical education, art, and music did not negatively influence academic performance ( Wilkins et al., 2003 ). Thus, the strategy of reducing time spent in physical education to increase academic performance may not have the desired effect. The evidence on in-school physical activity supports the provision of physical activity breaks during the school day as a way to increase fluid intelligence, time on task, and attention. However, it remains unclear what portion of these effects can be attributed to a break from academic time and what portion is a direct result of the specific demands/characteristics of the physical activity.
- THE DEVELOPING bRAIN, PHYSICAL ACTIVITY, AND BRAIN HEALTH
The study of brain health has grown beyond simply measuring behavioral outcomes such as task performance and reaction time (e.g., cognitive processing speed). New technology has emerged that has allowed scientists to understand the impact of lifestyle factors on the brain from the body systems level down to the molecular level. A greater understanding of the cognitive components that subserve academic performance and may be amenable to intervention has thereby been gained. Research conducted in both laboratory and field settings has helped define this line of inquiry and identify some preliminary underlying mechanisms.
The Evidence Base on the Relationship of Physical Activity to Brain Health and Cognition in Older Adults
Despite the current focus on the relationship of physical activity to cognitive development, the evidence base is larger on the association of physical activity with brain health and cognition during aging. Much can be learned about how physical activity affects childhood cognition and scholastic achievement through this work. Despite earlier investigations into the relationship of physical activity to cognitive aging (see Etnier et al., 1997 , for a review), the field was shaped by the findings of Kramer and colleagues (1999) , who examined the effects of aerobic fitness training on older adults using a randomized controlled design. Specifically, 124 older adults aged 60 and 75 were randomly assigned to a 6-month intervention of either walking (i.e., aerobic training) or flexibility (i.e., nonaerobic) training. The walking group but not the flexibility group showed improved cognitive performance, measured as a shorter response time to the presented stimulus. Results from a series of tasks that tapped different aspects of cognitive control indicated that engagement in physical activity is a beneficial means of combating cognitive aging ( Kramer et al., 1999 ).
Cognitive control, or executive control, is involved in the selection, scheduling, and coordination of computational processes underlying perception, memory, and goal-directed action. These processes allow for the optimization of behavioral interactions within the environment through flexible modulation of the ability to control attention ( MacDonald et al., 2000 ; Botvinick et al., 2001 ). Core cognitive processes that make up cognitive control or executive control include inhibition, working memory, and cognitive flexibility ( Diamond, 2006 ), processes mediated by networks that involve the prefrontal cortex. Inhibition (or inhibitory control) refers to the ability to override a strong internal or external pull so as to act appropriately within the demands imposed by the environment ( Davidson et al., 2006 ). For example, one exerts inhibitory control when one stops speaking when the teacher begins lecturing. Working memory refers to the ability to represent information mentally, manipulate stored information, and act on the information ( Davidson et al., 2006 ). In solving a difficult mathematical problem, for example, one must often remember the remainder. Finally, cognitive flexibility refers to the ability to switch perspectives, focus attention, and adapt behavior quickly and flexibly for the purposes of goal-directed action ( Blair et al., 2005 ; Davidson et al., 2006 ; Diamond, 2006 ). For example, one must shift attention from the teacher who is teaching a lesson to one's notes to write down information for later study.
Based on their earlier findings on changes in cognitive control induced by aerobic training, Colcombe and Kramer (2003) conducted a meta-analysis to examine the relationship between aerobic training and cognition in older adults aged 55-80 using data from 18 randomized controlled exercise interventions. Their findings suggest that aerobic training is associated with general cognitive benefits that are selectively and disproportionately greater for tasks or task components requiring greater amounts of cognitive control. A second and more recent meta-analysis ( Smith et al., 2010 ) corroborates the findings of Colcombe and Kramer, indicating that aerobic exercise is related to attention, processing speed, memory, and cognitive control; however, it should be noted that smaller effect sizes were observed, likely a result of the studies included in the respective meta-analyses. In older adults, then, aerobic training selectively improves cognition.
Hillman and colleagues (2006) examined the relationship between physical activity and inhibition (one aspect of cognitive control) using a computer-based stimulus-response protocol in 241 individuals aged 15-71. Their results indicate that greater amounts of physical activity are related to decreased response speed across task conditions requiring variable amounts of inhibition, suggesting a generalized relationship between physical activity and response speed. In addition, the authors found physical activity to be related to better accuracy across conditions in older adults, while no such relationship was observed for younger adults. Of interest, this relationship was disproportionately larger for the condition requiring greater amounts of inhibition in the older adults, suggesting that physical activity has both a general and selective association with task performance ( Hillman et al., 2006 ).
With advances in neuroimaging techniques, understanding of the effects of physical activity and aerobic fitness on brain structure and function has advanced rapidly over the past decade. In particular, a series of studies ( Colcombe et al., 2003 , 2004 , 2006 ; Kramer and Erickson, 2007 ; Hillman et al., 2008 ) of older individuals has been conducted to elucidate the relation of aerobic fitness to the brain and cognition. Normal aging results in the loss of brain tissue ( Colcombe et al., 2003 ), with markedly larger loss evidenced in the frontal, temporal, and parietal regions ( Raz, 2000 ). Thus cognitive functions subserved by these brain regions (such as those involved in cognitive control and aspects of memory) are expected to decay more dramatically than other aspects of cognition.
Colcombe and colleagues (2003) investigated the relationship of aerobic fitness to gray and white matter tissue loss using magnetic resonance imaging (MRI) in 55 healthy older adults aged 55-79. They observed robust age-related decreases in tissue density in the frontal, temporal, and parietal regions using voxel-based morphometry, a technique used to assess brain volume. Reductions in the amount of tissue loss in these regions were observed as a function of fitness. Given that the brain structures most affected by aging also demonstrated the greatest fitness-related sparing, these initial findings provide a biological basis for fitness-related benefits to brain health during aging.
In a second study, Colcombe and colleagues (2006) examined the effects of aerobic fitness training on brain structure using a randomized controlled design with 59 sedentary healthy adults aged 60-79. The treatment group received a 6-month aerobic exercise (i.e., walking) intervention, while the control group received a stretching and toning intervention that did not include aerobic exercise. Results indicated that gray and white matter brain volume increased for those who received the aerobic fitness training intervention. No such results were observed for those assigned to the stretching and toning group. Specifically, those assigned to the aerobic training intervention demonstrated increased gray matter in the frontal lobes, including the dorsal anterior cingulate cortex, the supplementary motor area, the middle frontal gyrus, the dorsolateral region of the right inferior frontal gyrus, and the left superior temporal lobe. White matter volume changes also were evidenced following the aerobic fitness intervention, with increases in white matter tracts being observed within the anterior third of the corpus callosum. These brain regions are important for cognition, as they have been implicated in the cognitive control of attention and memory processes. These findings suggest that aerobic training not only spares age-related loss of brain structures but also may in fact enhance the structural health of specific brain regions.
In addition to the structural changes noted above, research has investigated the relationship between aerobic fitness and changes in brain function. That is, aerobic fitness training has also been observed to induce changes in patterns of functional activation. Functional MRI (fMRI) measures, which make it possible to image activity in the brain while an individual is performing a cognitive task, have revealed that aerobic training induces changes in patterns of functional activation. This approach involves inferring changes in neuronal activity from alteration in blood flow or metabolic activity in the brain. In a seminal paper, Colcombe and colleagues (2004) examined the relationship of aerobic fitness to brain function and cognition across two studies with older adults. In the first study, 41 older adult participants (mean age ~66) were divided into higher- and lower-fit groups based on their performance on a maximal exercise test. In the second study, 29 participants (aged 58-77) were recruited and randomly assigned to either a fitness training (i.e., walking) or control (i.e., stretching and toning) intervention. In both studies, participants were given a task requiring variable amounts of attention and inhibition. Results indicated that fitness (study 1) and fitness training (study 2) were related to greater activation in the middle frontal gyrus and superior parietal cortex; these regions of the brain are involved in attentional control and inhibitory functioning, processes entailed in the regulation of attention and action. These changes in neural activation were related to significant improvements in performance on the cognitive control task of attention and inhibition.
Taken together, the findings across studies suggest that an increase in aerobic fitness, derived from physical activity, is related to improvements in the integrity of brain structure and function and may underlie improvements in cognition across tasks requiring cognitive control. Although developmental differences exist, the general paradigm of this research can be applied to early stages of the life span, and some early attempts to do so have been made, as described below. Given the focus of this chapter on childhood cognition, it should be noted that this section has provided only a brief and arguably narrow look at the research on physical activity and cognitive aging. Considerable work has detailed the relationship of physical activity to other aspects of adult cognition using behavioral and neuroimaging tools (e.g., Boecker, 2011 ). The interested reader is referred to a number of review papers and meta-analyses describing the relationship of physical activity to various aspects of cognitive and brain health ( Etnier et al., 1997 ; Colcombe and Kramer, 2003 ; Tomporowski, 2003 ; Thomas et al., 2012 ).
Child Development, Brain Structure, and Function
Certain aspects of development have been linked with experience, indicating an intricate interplay between genetic programming and environmental influences. Gray matter, and the organization of synaptic connections in particular, appears to be at least partially dependent on experience (NRC/IOM, 2000; Taylor, 2006 ), with the brain exhibiting a remarkable ability to reorganize itself in response to input from sensory systems, other cortical systems, or insult ( Huttenlocher and Dabholkar, 1997 ). During typical development, experience shapes the pruning process through the strengthening of neural networks that support relevant thoughts and actions and the elimination of unnecessary or redundant connections. Accordingly, the brain responds to experience in an adaptive or “plastic” manner, resulting in the efficient and effective adoption of thoughts, skills, and actions relevant to one's interactions within one's environmental surroundings. Examples of neural plasticity in response to unique environmental interaction have been demonstrated in human neuroimaging studies of participation in music ( Elbert et al., 1995 ; Chan et al., 1998 ; Münte et al., 2001 ) and sports ( Hatfield and Hillman, 2001 ; Aglioti et al., 2008 ), thus supporting the educational practice of providing music education and opportunities for physical activity to children.
Effects of Regular Engagement in Physical Activity and Physical Fitness on Brain Structure
Recent advances in neuroimaging techniques have rapidly advanced understanding of the role physical activity and aerobic fitness may have in brain structure. In children a growing body of correlational research suggests differential brain structure related to aerobic fitness. Chaddock and colleagues (2010a , b ) showed a relationship among aerobic fitness, brain volume, and aspects of cognition and memory. Specifically, Chaddock and colleagues (2010a) assigned 9- to 10-year-old preadolescent children to lower- and higher-fitness groups as a function of their scores on a maximal oxygen uptake (VO 2 max) test, which is considered the gold-standard measure of aerobic fitness. They observed larger bilateral hippocampal volume in higher-fit children using MRI, as well as better performance on a task of relational memory. It is important to note that relational memory has been shown to be mediated by the hippocampus ( Cohen and Eichenbaum, 1993 ; Cohen et al., 1999 ). Further, no differences emerged for a task condition requiring item memory, which is supported by structures outside the hippocampus, suggesting selectivity among the aspects of memory that benefit from higher amounts of fitness. Lastly, hippocampal volume was positively related to performance on the relational memory task but not the item memory task, and bilateral hippocampal volume was observed to mediate the relationship between fitness and relational memory ( Chaddock et al., 2010a ). Such findings are consistent with behavioral measures of relational memory in children ( Chaddock et al., 2011 ) and neuroimaging findings in older adults ( Erickson et al., 2009 , 2011 ) and support the robust nonhuman animal literature demonstrating the effects of exercise on cell proliferation ( Van Praag et al., 1999 ) and survival ( Neeper et al., 1995 ) in the hippocampus.
In a second investigation ( Chaddock et al., 2010b ), higher- and lower-fit children (aged 9-10) underwent an MRI to determine whether structural differences might be found that relate to performance on a cognitive control task that taps attention and inhibition. The authors observed differential findings in the basal ganglia, a subcortical structure involved in the interplay of cognition and willed action. Specifically, higher-fit children exhibited greater volume in the dorsal striatum (i.e., caudate nucleus, putamen, globus pallidus) relative to lower-fit children, while no differences were observed in the ventral striatum. Such findings are not surprising given the role of the dorsal striatum in cognitive control and response resolution ( Casey et al., 2008 ; Aron et al., 2009 ), as well as the growing body of research in children and adults indicating that higher levels of fitness are associated with better control of attention, memory, and cognition ( Colcombe and Kramer, 2003 ; Hillman et al., 2008 ; Chang and Etnier, 2009 ). Chaddock and colleagues (2010b) further observed that higher-fit children exhibited increased inhibitory control and response resolution and that higher basal ganglia volume was related to better task performance. These findings indicate that the dorsal striatum is involved in these aspects of higher-order cognition and that fitness may influence cognitive control during preadolescent development. It should be noted that both studies described above were correlational in nature, leaving open the possibility that other factors related to fitness and/or the maturation of subcortical structures may account for the observed group differences.
Effects of Regular Engagement in Physical Activity and Physical Fitness on Brain Function
Other research has attempted to characterize fitness-related differences in brain function using fMRI and event-related brain potentials (ERPs), which are neuroelectric indices of functional brain activation in the electro-encephalographic time series. To date, few randomized controlled interventions have been conducted. Notably, Davis and colleagues (2011) conducted one such intervention lasting approximately 14 weeks that randomized 20 sedentary overweight preadolescent children into an after-school physical activity intervention or a nonactivity control group. The fMRI data collected during an antisaccade task, which requires inhibitory control, indicated increased bilateral activation of the prefrontal cortex and decreased bilateral activation of the posterior parietal cortex following the physical activity intervention relative to the control group. Such findings illustrate some of the neural substrates influenced by participation in physical activity. Two additional correlational studies ( Voss et al., 2011 ; Chaddock et al., 2012 ) compared higher- and lower-fit preadolescent children and found differential brain activation and superior task performance as a function of fitness. That is, Chaddock and colleagues (2012) observed increased activation in prefrontal and parietal brain regions during early task blocks and decreased activation during later task blocks in higher-fit relative to lower-fit children. Given that higher-fit children outperformed lower-fit children on the aspects of the task requiring the greatest amount of cognitive control, the authors reason that the higher-fit children were more capable of adapting neural activity to meet the demands imposed by tasks that tapped higher-order cognitive processes such as inhibition and goal maintenance. Voss and colleagues (2011) used a similar task to vary cognitive control requirements and found that higher-fit children outperformed their lower-fit counterparts and that such differences became more pronounced during task conditions requiring the upregulation of control. Further, several differences emerged across various brain regions that together make up the network associated with cognitive control. Collectively, these differences suggest that higher-fit children are more efficient in the allocation of resources in support of cognitive control operations.
Other imaging research has examined the neuroelectric system (i.e., ERPs) to investigate which cognitive processes occurring between stimulus engagement and response execution are influenced by fitness. Several studies ( Hillman et al., 2005 , 2009 ; Pontifex et al., 2011 ) have examined the P3 component of the stimulus-locked ERP and demonstrated that higher-fit children have larger-amplitude and shorter-latency ERPs relative to their lower-fit peers. Classical theory suggests that P3 relates to neuronal activity associated with revision of the mental representation of the previous event within the stimulus environment ( Donchin, 1981 ). P3 amplitude reflects the allocation of attentional resources when working memory is updated ( Donchin and Coles, 1988 ) such that P3 is sensitive to the amount of attentional resources allocated to a stimulus ( Polich, 1997 ; Polich and Heine, 2007 ). P3 latency generally is considered to represent stimulus evaluation and classification speed ( Kutas et al., 1977 ; Duncan-Johnson, 1981 ) and thus may be considered a measure of stimulus detection and evaluation time ( Magliero et al., 1984 ; Ila and Polich, 1999 ). Therefore the above findings suggest that higher-fit children allocate greater attentional resources and have faster cognitive processing speed relative to lower-fit children ( Hillman et al., 2005 , 2009 ), with additional research suggesting that higher-fit children also exhibit greater flexibility in the allocation of attentional resources, as indexed by greater modulation of P3 amplitude across tasks that vary in the amount of cognitive control required ( Pontifex et al., 2011 ). Given that higher-fit children also demonstrate better performance on cognitive control tasks, the P3 component appears to reflect the effectiveness of a subset of cognitive systems that support willed action ( Hillman et al., 2009 ; Pontifex et al., 2011 ).
Two ERP studies ( Hillman et al., 2009 ; Pontifex et al., 2011 ) have focused on aspects of cognition involved in action monitoring. That is, the error-related negativity (ERN) component was investigated in higher- and lower-fit children to determine whether differences in evaluation and regulation of cognitive control operations were influenced by fitness level. The ERN component is observed in response-locked ERP averages. It is often elicited by errors of commission during task performance and is believed to represent either the detection of errors during task performance ( Gehring et al., 1993 ; Holroyd and Coles, 2002 ) or more generally the detection of response conflict ( Botvinick et al., 2001 ; Yeung et al., 2004 ), which may be engendered by errors in response production. Several studies have reported that higher-fit children exhibit smaller ERN amplitude during rapid-response tasks (i.e., instructions emphasizing speed of responding; Hillman et al., 2009 ) and more flexibility in the allocation of these resources during tasks entailing variable cognitive control demands, as evidenced by changes in ERN amplitude for higher-fit children and no modulation of ERN in lower-fit children ( Pontifex et al., 2011 ). Collectively, this pattern of results suggests that children with lower levels of fitness allocate fewer attentional resources during stimulus engagement (P3 amplitude) and exhibit slower cognitive processing speed (P3 latency) but increased activation of neural resources involved in the monitoring of their actions (ERN amplitude). Alternatively, higher-fit children allocate greater resources to environmental stimuli and demonstrate less reliance on action monitoring (increasing resource allocation only to meet the demands of the task). Under more demanding task conditions, the strategy of lower-fit children appears to fail since they perform more poorly under conditions requiring the upregulation of cognitive control.
Finally, only one randomized controlled trial published to date has used ERPs to assess neurocognitive function in children. Kamijo and colleagues (2011) studied performance on a working memory task before and after a 9-month physical activity intervention compared with a wait-list control group. They observed better performance following the physical activity intervention during task conditions that required the upregulation of working memory relative to the task condition requiring lesser amounts of working memory. Further, increased activation of the contingent negative variation (CNV), an ERP component reflecting cognitive and motor preparation, was observed at posttest over frontal scalp sites in the physical activity intervention group. No differences in performance or brain activation were noted for the wait-list control group. These findings suggest an increase in cognitive preparation processes in support of a more effective working memory network resulting from prolonged participation in physical activity. For children in a school setting, regular participation in physical activity as part of an after-school program is particularly beneficial for tasks that require the use of working memory.
Adiposity and Risk for Metabolic Syndrome as It Relates to Cognitive Health
A related and emerging literature that has recently been popularized investigates the relationship of adiposity to cognitive and brain health and academic performance. Several reports ( Datar et al., 2004 ; Datar and Sturm, 2006 ; Judge and Jahns, 2007 ; Gable et al., 2012 ) on this relationship are based on large-scale datasets derived from the Early Child Longitudinal Study. Further, nonhuman animal research has been used to elucidate the relationships between health indices and cognitive and brain health (see Figure 4-4 for an overview of these relationships). Collectively, these studies observed poorer future academic performance among children who entered school overweight or moved from a healthy weight to overweight during the course of development. Corroborating evidence for a negative relationship between adiposity and academic performance may be found in smaller but more tightly controlled studies. As noted above, Castelli and colleagues (2007) observed poorer performance on the mathematics and reading portions of the Illinois Standardized Achievement Test in 3rd- and 5th-grade students as a function of higher BMI, and Donnelly and colleagues (2009) used a cluster randomized trial to demonstrate that physical activity in the classroom decreased BMI and improved academic achievement among pre-adolescent children.
Relationships between health indices and cognitive and brain health. NOTE: AD = Alzheimer's disease; PD = Parkinson's disease. SOURCE: Cotman et al., 2007. Reprinted with permission.
Recently published reports describe the relationship between adiposity and cognitive and brain health to advance understanding of the basic cognitive processes and neural substrates that may underlie the adiposity-achievement relationship. Bolstered by findings in adult populations (e.g., Debette et al., 2010 ; Raji et al., 2010 ; Carnell et al., 2011 ), researchers have begun to publish data on preadolescent populations indicating differences in brain function and cognitive performance related to adiposity (however, see Gunstad et al., 2008 , for an instance in which adiposity was unrelated to cognitive outcomes). Specifically, Kamijo and colleagues (2012a) examined the relationship of weight status to cognitive control and academic achievement in 126 children aged 7-9. The children completed a battery of cognitive control tasks, and their body composition was assessed using dual X-ray absorptiometry (DXA). The authors found that higher BMI and greater amounts of fat mass (particularly in the midsection) were related to poorer performance on cognitive control tasks involving inhibition, as well as lower academic achievement. In follow-up studies, Kamijo and colleagues (2012b) investigated whether neural markers of the relationship between adiposity and cognition may be found through examination of ERP data. These studies compared healthy-weight and obese children and found a differential distribution of the P3 potential (i.e., less frontally distributed) and larger N2 amplitude, as well as smaller ERN magnitude, in obese children during task conditions that required greater amounts of inhibitory control ( Kamijo et al., 2012c ). Taken together, the above results suggest that obesity is associated with less effective neural processes during stimulus capture and response execution. As a result, obese children perform tasks more slowly ( Kamijo et al., 2012a ) and are less accurate ( Kamijo et al., 2012b , c ) in response to tasks requiring variable amounts of cognitive control. Although these data are correlational, they provide a basis for further study using other neuroimaging tools (e.g., MRI, fMRI), as well as a rationale for the design and implementation of randomized controlled studies that would allow for causal interpretation of the relationship of adiposity to cognitive and brain health. The next decade should provide a great deal of information on this relationship.
- LIMITATIONS
Despite the promising findings described in this chapter, it should be noted that the study of the relationship of childhood physical activity, aerobic fitness, and adiposity to cognitive and brain health and academic performance is in its early stages. Accordingly, most studies have used designs that afford correlation rather than causation. To date, in fact, only two randomized controlled trials ( Davis et al., 2011 ; Kamijo et al., 2011 ) on this relationship have been published. However, several others are currently ongoing, and it was necessary to provide evidence through correlational studies before investing the effort, time, and funding required for more demanding causal studies. Given that the evidence base in this area has grown exponentially in the past 10 years through correlational studies and that causal evidence has accumulated through adult and nonhuman animal studies, the next step will be to increase the amount of causal evidence available on school-age children.
Accomplishing this will require further consideration of demographic factors that may moderate the physical activity–cognition relationship. For instance, socioeconomic status has a unique relationship with physical activity ( Estabrooks et al., 2003 ) and cognitive control ( Mezzacappa, 2004 ). Although many studies have attempted to control for socioeconomic status (see Hillman et al., 2009 ; Kamijo et al., 2011 , 2012a , b , c ; Pontifex et al., 2011 ), further inquiry into its relationship with physical activity, adiposity, and cognition is warranted to determine whether it may serve as a potential mediator or moderator for the observed relationships. A second demographic factor that warrants further consideration is gender. Most authors have failed to describe gender differences when reporting on the physical activity–cognition literature. However, studies of adiposity and cognition have suggested that such a relationship may exist (see Datar and Sturm, 2006 ). Additionally, further consideration of age is warranted. Most studies have examined a relatively narrow age range, consisting of a few years. Such an approach often is necessary because of maturation and the need to develop comprehensive assessment tools that suit the various stages of development. However, this approach has yielded little understanding of how the physical activity–cognition relationship may change throughout the course of maturation.
Finally, although a number of studies have described the relationship of physical activity, fitness, and adiposity to standardized measures of academic performance, few attempts have been made to observe the relationship within the context of the educational environment. Standardized tests, although necessary to gauge knowledge, may not be the most sensitive measures for (the process of) learning. Future research will need to do a better job of translating promising laboratory findings to the real world to determine the value of this relationship in ecologically valid settings.
From an authentic and practical to a mechanistic perspective, physically active and aerobically fit children consistently outperform their inactive and unfit peers academically on both a short- and a long-term basis. Time spent engaged in physical activity is related not only to a healthier body but also to enriched cognitive development and lifelong brain health. Collectively, the findings across the body of literature in this area suggest that increases in aerobic fitness, derived from physical activity, are related to improvements in the integrity of brain structure and function that underlie academic performance. The strongest relationships have been found between aerobic fitness and performance in mathematics, reading, and English. For children in a school setting, regular participation in physical activity is particularly beneficial with respect to tasks that require working memory and problem solving. These findings are corroborated by the results of both authentic correlational studies and experimental randomized controlled trials. Overall, the benefits of additional time dedicated to physical education and other physical activity opportunities before, during, and after school outweigh the benefits of exclusive utilization of school time for academic learning, as physical activity opportunities offered across the curriculum do not inhibit academic performance.
Both habitual and single bouts of physical activity contribute to enhanced academic performance. Findings indicate a robust relationship of acute exercise to increased attention, with evidence emerging for a relationship between participation in physical activity and disciplinary behaviors, time on task, and academic performance. Specifically, higher-fit children allocate greater resources to a given task and demonstrate less reliance on environmental cues or teacher prompting.
- Åberg MA, Pedersen NL, Torén K, Svartengren M, Bäckstrand B, Johnsson T, Cooper-Kuhn CM, Åberg ND, Nilsson M, Kuhn HG. Cardiovascular fitness is associated with cognition in young adulthood. Proceedings of the National Academy of Sciences of the United States of America. 2009; 106 (49):20906–20911. [ PMC free article : PMC2785721 ] [ PubMed : 19948959 ]
- Aglioti SM, Cesari P, Romani M, Urgesi C. Action anticipation and motor resonance in elite basketball players. Nature Neuroscience. 2008; 11 (9):1109–1116. [ PubMed : 19160510 ]
- Ahamed Y, Macdonald H, Reed K, Naylor PJ, Liu-Ambrose T, McKay H. School-based physical activity does not compromise children's academic performance. Medicine and Science in Sports and Exercise. 2007; 39 (2):371–376. [ PubMed : 17277603 ]
- Aron A, Poldrack R, Wise S. Cognition: Basal ganglia role. Encyclopedia of Neuroscience. 2009; 2 :1069–1077.
- Barros RM, Silver EJ, Stein REK. School recess and group classroom behavior. Pediatrics. 2009; 123 (2):431–436. [ PubMed : 19171606 ]
- Bartholomew JB, Jowers EM. Physically active academic lessons in elementary children. Preventive Medicine. 2011; 52 (Suppl 1):S51–S54. [ PMC free article : PMC3116963 ] [ PubMed : 21281672 ]
- Basch C. Healthier children are better learners: A missing link in school reforms to close the achievement gap. 2010. [October 11, 2011]. http://www .equitycampaign .org/i/a/document /12557_EquityMattersVol6_Web03082010 .pdf . [ PubMed : 21923870 ]
- Baxter SD, Royer JA, Hardin JW, Guinn CH, Devlin CM. The relationship of school absenteeism with body mass index, academic achievement, and socioeconomic status among fourth grade children. Journal of School Health. 2011; 81 (7):417–423. [ PMC free article : PMC3972016 ] [ PubMed : 21668882 ]
- Benden ME, Blake JJ, Wendel ML, Huber JC Jr. The impact of stand-biased desks in classrooms on calorie expenditure in children. American Journal of Public Health. 2011; 101 (8):1433–1436. [ PMC free article : PMC3134494 ] [ PubMed : 21421945 ]
- Biddle SJ, Asare M. Physical activity and mental health in children and adolescents: A review of reviews. British Journal of Sports Medicine. 2011; 45 (11):886–895. [ PubMed : 21807669 ]
- Blair C, Zelazo PD, Greenberg MT. The measurement of executive function in early childhood. Developmental Neuropsychology. 2005; 28 (2):561–571. [ PubMed : 16144427 ]
- Boecker H. On the emerging role of neuroimaging in determining functional and structural brain integrity induced by physical exercise: Impact for predictive, preventive, and personalized medicine. EPMA Journal. 2011; 2 (3):277–285. [ PMC free article : PMC3405390 ] [ PubMed : 23199163 ]
- Botvinick MM, Braver TS, Barch DM, Carter CS, Cohen JD. Conflict monitoring and cognitive control. Psychological Review. 2001; 108 (3):624. [ PubMed : 11488380 ]
- Budde H, Voelcker-Rehage C, S-Pietrabyk Kendziorra, Ribeiro P, Tidow G. Acute coordinative exercise improves attentional performance in adolescents. Neuroscience Letters. 2008; 441 (2):219–223. [ PubMed : 18602754 ]
- Burkhalter TM, Hillman CH. A narrative review of physical activity, nutrition, and obesity to cognition and scholastic performance across the human lifespan. Advances in Nutrition. 2011; 2 (2):201S–206S. [ PMC free article : PMC3065760 ] [ PubMed : 22332052 ]
- Carlson SA, Fulton JE, Lee SM, Maynard LM, Brown DR, Kohl HW III, Dietz WH. Physical education and academic achievement in elementary school: Data from the Early Childhood Longitudinal Study. American Journal of Public Health. 2008; 98 (4):721–727. [ PMC free article : PMC2377002 ] [ PubMed : 18309127 ]
- Carnell S, Gibson C, Benson L, Ochner C, Geliebter A. Neuroimaging and obesity: Current knowledge and future directions. Obesity Reviews. 2011; 13 (1):43–56. [ PMC free article : PMC3241905 ] [ PubMed : 21902800 ]
- Casey B, Jones RM, Hare TA. The adolescent brain. Annals of the New York Academy of Sciences. 2008; 1124 (1):111–126. [ PMC free article : PMC2475802 ] [ PubMed : 18400927 ]
- Castelli DM, Hillman CH, Buck SM, Erwin HE. Physical fitness and academic achievement in third- and fifth-grade students. Journal of Sport and Exercise Psychology. 2007; 29 (2):239–252. [ PubMed : 17568069 ]
- Chaddock L, Erickson KI, Prakash RS, Kim JS, Voss MW, VanPatter M, Pontifex MB, Raine LB, Konkel A, Hillman CH. A neuroimaging investigation of the association between aerobic fitness, hippocampal volume, and memory performance in preadolescent children. Brain Research. 2010a; 1358 :172–183. [ PMC free article : PMC3953557 ] [ PubMed : 20735996 ]
- Chaddock L, Erickson KI, Prakash RS, VanPatter M, Voss MW, Pontifex MB, Raine LB, Hillman CH, Kramer AF. Basal ganglia volume is associated with aerobic fitness in preadolescent children. Developmental Neuroscience. 2010b; 32 (3):249–256. [ PMC free article : PMC3696376 ] [ PubMed : 20693803 ]
- Chaddock L, Hillman CH, Buck SM, Cohen NJ. Aerobic fitness and executive control of relational memory in preadolescent children. Medicine and Science in Sports and Exercise. 2011; 43 (2):344. [ PubMed : 20508533 ]
- Chaddock L, Erickson KI, Prakash RS, Voss MW, VanPatter M, Pontifex MB, Hillman CH, Kramer AF. A functional MRI investigation of the association between childhood aerobic fitness and neurocognitive control. Biological Psychology. 2012; 89 (1):260–268. [ PubMed : 22061423 ]
- Chan AS, Ho YC, Cheung MC. Music training improves verbal memory. Nature. 1998; 396 (6707):128. [ PubMed : 9823892 ]
- Chang YK, Etnier JL. Effects of an acute bout of localized resistance exercise on cognitive performance in middle-aged adults: A randomized controlled trial study. Psychology of Sport and Exercise. 2009; 10 (1):19–24.
- Chih CH, Chen JF. The relationship between physical education performance, fitness tests, and academic achievement in elementary school. International Journal of Sport and Society. 2011; 2 (1):65–73.
- Chomitz VR, Slining MM, McGowan RJ, Mitchell SE, Dawson GF, Hacker KA. Is there a relationship between physical fitness and academic achievement? Positive results from public school children in the northeastern United States. Journal of School Health. 2008; 79 (1):30–37. [ PubMed : 19149783 ]
- Coe DP, Pivarnik JM, Womack CJ, Reeves MJ, Malina RM. Effect of physical education and activity levels on academic achievement in children. Medicine and Science in Sports and Exercise. 2006; 38 (8):1515–1519. [ PubMed : 16888468 ]
- Cohen NJ, Eichenbaum H. Memory, amnesia, and the hippocampal system. Cambridge, MA: MIT Press; 1993.
- Cohen NJ, Ryan J, Hunt C, Romine L, Wszalek T, Nash C. Hippocampal system and declarative (relational) memory: Summarizing the data from functional neuroimaging studies. Hippocampus. 1999; 9 (1):83–98. [ PubMed : 10088903 ]
- Colcombe SJ, Kramer AF. Fitness effects on the cognitive function of older adults a meta-analytic study. Psychological Science. 2003; 14 (2):125–130. [ PubMed : 12661673 ]
- Colcombe SJ, Erickson KI, Raz N, Webb AG, Cohen NJ, McAuley E, Kramer AF. Aerobic fitness reduces brain tissue loss in aging humans. Journals of Gerontology Series A: Biological Sciences and Medical Sciences. 2003; 58 (2):M176–M180. [ PubMed : 12586857 ]
- Colcombe SJ, Kramer AF, Erickson KI, Scalf P, McAuley E, Cohen NJ, Webb A, Jerome GJ, Marquez DX, Elavsky S. Cardiovascular fitness, cortical plasticity, and aging. Proceedings of the National Academy of Sciences of the United States of America. 2004; 101 (9):3316–3321. [ PMC free article : PMC373255 ] [ PubMed : 14978288 ]
- Colcombe SJ, Erickson KI, Scalf PE, Kim JS, Prakash R, McAuley E, Elavsky S, Marquez DX, Hu L, Kramer AF. Aerobic exercise training increases brain volume in aging humans. Journals of Gerontology Series A: Biological Sciences and Medical Sciences. 2006; 61 (11):1166–1170. [ PubMed : 17167157 ]
- Cooper K, Everett D, Kloster J, Meredith MD, Rathbone M, Read K. Preface: Texas statewide assessment of youth fitness. Research Quarterly for Exercise and Sport. 2010; 81 (3):ii. [ PubMed : 21049831 ]
- Cotman CW, Berchtold NC, Christie LA. Exercise builds brain health: Key roles of growth factor cascades and inflammation. Trends in Neurosciences. 2007; 30 (9):464–472. [ PubMed : 17765329 ]
- Cottrell LA, Northrup K, Wittberg R. The extended relationship between child cardiovascular risks and academic performance measures. Obesity (Silver Spring). 2007; 15 (12):3170–3177. [ PubMed : 18198328 ]
- Crosnoe R. Academic and health-related trajectories in high school: The intersection of gender and athletics. Journal of Health and Social Behavior. 2002; 43 :317–335. [ PubMed : 12467256 ]
- Daley AJ, Ryan J. Academic performance and participation in physical activity by secondary school adolescents. Perceptual and Motor Skills. 2000; 91 (2):531–534. [ PubMed : 11065314 ]
- Datar A, Sturm R. Physical education in elementary school and body mass index: Evidence from the Early Childhood Longitudinal Study. Journal Information. 2004; 94 (9):1501–1509. [ PMC free article : PMC1448481 ] [ PubMed : 15333302 ]
- Datar A, Sturm R. Childhood overweight and elementary school outcomes. International Journal of Obesity. 2006; 30 (9):1449–1460. [ PubMed : 16534518 ]
- Datar A, Sturm R, Magnabosco JL. Childhood overweight and academic performance: National study of kindergartners and first-graders. Obesity Research. 2004; 12 (1):58–68. [ PubMed : 14742843 ]
- Davidson MC, Amso D, Anderson LC, Diamond A. Development of cognitive control and executive functions from 4 to 13 years: Evidence from manipulations of memory, inhibition, and task switching. Neuropsychologia. 2006; 44 (11):2037. [ PMC free article : PMC1513793 ] [ PubMed : 16580701 ]
- Davis CL, Tomporowski PD, McDowell JE, Austin BP, Miller PH, Yanasak NE, Allison JD, Naglieri JA. Exercise improves executive function and achievement and alters brain activation in overweight children: A randomized, controlled trial. Health Psychology. 2011; 30 (1):91–98. [ PMC free article : PMC3057917 ] [ PubMed : 21299297 ]
- Dawson P, Guare R. Executive skills in children and adolescents: A practical guide to assessment and intervention. New York: Guilford Press; 2004. pp. 2–8.
- Debette S, Beiser A, Hoffmann U, DeCarli C, O'Donnell CJ, Massaro JM, Au R, Himali JJ, Wolf PA, Fox CS, Seshadri S. Visceral fat is associated with lower brain volume in healthy middle-aged adults. Annals of Neurology. 2010; 68 :136–144. [ PMC free article : PMC2933649 ] [ PubMed : 20695006 ]
- Dexter TT. Relationships between sport knowledge, sport performance and academic ability: Empirical evidence from GCSE physical education. Journal of Sports Sciences. 1999; 17 (4):283–295. [ PubMed : 10373038 ]
- Diamond A. The early development of executive functions. In: Bialystok E, Craik FIM, editors. In Lifespan cognition: Mechanisms of change. New York: Oxford University Press; 2006. pp. 70–95.
- Donchin E. Surprise! … surprise. Psychophysiology. 1981; 18 (5):493–513. [ PubMed : 7280146 ]
- Donchin E, Coles MGH. Is the P300 component a manifestation of context updating. Behavioral and Brain Sciences. 1988; 11 (03):357–374.
- Donnelly JE, Lambourne K. Classroom-based physical activity, cognition, and academic achievement. Preventive Medicine. 2011; 52 (Suppl 1):S36–S42. [ PubMed : 21281666 ]
- Donnelly JE, Greene JL, Gibson CA, Smith BK, Washburn RA, Sullivan DK, DuBose K, Mayo MS, Schmelzle KH, Ryan JJ. Physical Activity Across the Curriculum (PAAC): A randomized controlled trial to promote physical activity and diminish overweight and obesity in elementary school children. Preventive Medicine. 2009; 49 (4):336–341. [ PMC free article : PMC2766439 ] [ PubMed : 19665037 ]
- Drollette ES, Shishido T, Pontifex MB, Hillman CH. Maintenance of cognitive control during and after walking in preadolescent children. Medicine and Science in Sports and Exercise. 2012; 44 (10):2017–2024. [ PubMed : 22525770 ]
- Duncan SC, Duncan TE, Strycker LA, Chaumeton NR. A cohort-sequential latent growth model of physical activity from ages 12 to 17 years. Annals of Behavioral Medicine. 2007; 33 (1):80–89. [ PMC free article : PMC2729662 ] [ PubMed : 17291173 ]
- Duncan-Johnson CC. P3 latency: A new metric of information processing. Psychophysiology. 1981; 18 :207–215. [ PubMed : 7291436 ]
- Dwyer T, Coonan W, Worsley A, Leitch D. An assessment of the effects of two physical activity programmes on coronary heart disease risk factors in primary school children. Community Health Studies. 1979; 3 (3):196–202.
- Dwyer T, Coonan WE, Leitch DR, Hetzel BS, Baghurst R. An investigation of the effects of daily physical activity on the health of primary school students in south Australia. International Journal of Epidemiology. 1983; 12 (3):308–313. [ PubMed : 6629620 ]
- Edwards JU, Mauch L, Winkleman MR. Relationship of nutrition and physical activity behaviors and fitness measures to academic performance for sixth graders in a Midwest city school district. Journal of School Health. 2011; 81 :65–73. [ PubMed : 21223273 ]
- Efrat M. The relationship between low-income and minority children's physical activity and academic-related outcomes: A review of the literature. Health Education and Behavior. 2011; 38 (5):441–451. [ PubMed : 21285376 ]
- Eitle TM. Do gender and race matter? Explaining the relationship between sports participation and achievement. Sociological Spectrum. 2005; 25 (2):177–195.
- Eitle TM, Eitle DJ. Sociology of Education. 2002. Race, cultural capital, and the educational effects of participation in sports; pp. 123–146.
- Elbert T, Pantev C, Wienbruch C, Rockstroh B, Taub E. Increased cortical representation of the fingers of the left hand in string players. Science. 1995; 270 (5234):305–307. [ PubMed : 7569982 ]
- Elder C, Leaver-Dunn D, Wang MQ, Nagy S, Green L. Organized group activity as a protective factor against adolescent substance use. American Journal of Health Behavior. 2000; 24 (2):108–113.
- Ellemberg D, St-Louis-Deschênes M. The effect of acute physical exercise on cognitive function during development. Psychology of Sport and Exercise. 2010; 11 (2):122–126.
- Erickson KI, Prakash RS, Voss MW, Chaddock L, Hu L, Morris KS, White SM, Wójcicki TR, McAuley E, Kramer AF. Aerobic fitness is associated with hippocampal volume in elderly humans. Hippocampus. 2009; 19 (10):1030–1039. [ PMC free article : PMC3072565 ] [ PubMed : 19123237 ]
- Erickson KI, Voss MW, Prakash RS, Basak C, Szabo A, Chaddock L, Kim JS, Heo S, Alves H, White SM. Exercise training increases size of hippocampus and improves memory. Proceedings of the National Academy of Sciences of the United States of America. 2011; 108 (7):3017–3022. [ PMC free article : PMC3041121 ] [ PubMed : 21282661 ]
- Ericsson KA, Charness N. Expert performance: Its structure and acquisition. American Psychologist. 1994; 49 (8):725.
- Estabrooks AP, Lee RE, Gyurcsik NC. Resources for physical activity participation: Does availability and accessibility differ by neighborhood socioeconomic status. Annals of Behavioral Medicine. 2003; 25 (2):100–104. [ PubMed : 12704011 ]
- Etnier JL, Salazar W, Landers DM, Petruzzello SJ, Han M, Nowell P. The influence of physical fitness and exercise upon cognitive functioning: A meta-analysis. Journal of Sport and Exercise Psychology. 1997; 19 (3):249–277.
- Etnier JL, Nowell PM, Landers DM, Sibley BA. A meta-regression to examine the relationship between aerobic fitness and cognitive performance. Brain Research Reviews. 2006; 52 (1):119–130. [ PubMed : 16490256 ]
- Eveland-Sayers BM, Farley RS, Fuller DK, Morgan DW, Caputo JL. Physical fitness and academic achievement in elementary school children. Journal of Physical Activity and Health. 2009; 6 (1):99. [ PubMed : 19211963 ]
- Fan X, Chen M. Parental involvement and students' academic achievement: A meta-analysis. Educational Psychology Review. 2001; 13 (1):1–22.
- Fedewa AL, Ahn S. The effects of physical activity and physical fitness on children's achievement and cognitive outcomes: A meta-analysis. Research Quarterly for Exercise and Sport. 2011; 82 (3):521–535. [ PubMed : 21957711 ]
- Fisher M, Juszczak L, Friedman SB. Sports participation in an urban high school: Academic and psychologic correlates. Journal of Adolescent Health. 1996; 18 (5):329–334. [ PubMed : 9156545 ]
- Fox CK, Barr-Anderson D, D-Neumark Sztainer, Wall M. Physical activity and sports team participation: Associations with academic outcomes in middle school and high school students. Journal of School Health. 2010; 80 (1):31–37. [ PubMed : 20051088 ]
- Fredericks CR, Kokot SJ, Krog S. Using a developmental movement programme to enhance academic skills in grade 1 learners. South African Journal for Research in Sport, Physical Education and Recreation. 2006; 28 (1):29–42.
- Gabbard C, Barton J. Effects of physical activity on mathematical computation among young children. Journal of Psychology. 1979; 103 :287–288.
- Gable S, Krull JL, Chang Y. Boys' and girls' weight status and math performance from kindergarten entry through fifth grade: A mediated analysis. Child Development. 2012; 83 (5):1822–1839. [ PubMed : 22694240 ]
- Gehring WJ, Goss B, Coles MG, Meyer DE, Donchin E. A neural system for error detection and compensation. Psychological Science. 1993; 4 (6):385–390.
- Getlinger MJ, Laughlin V, Bell E, Akre C, Arjmandi BH. Food waste is reduced when elementary-school children have recess before lunch. Journal of the American Dietetic Association. 1996; 96 (9):906. [ PubMed : 8784336 ]
- Glenmark B. Skeletal muscle fiber types, physical performance, physical activity and attitude to physical activity in women and men: A follow-up from age 16-27. Acta Physiologica Scandinavica Supplementum. 1994; 623 :1–47. [ PubMed : 7942046 ]
- Grieco LA, Jowers EM, Bartholomew JB. Physically active academic lessons and time on task: The moderating effect of body mass index. Medicine and Science in Sports and Exercise. 2009; 41 (10):1921–1926. [ PubMed : 19727020 ]
- Grissom JB. Physical fitness and academic achievement. Journal of Exercise Physiology Online. 2005; 8 (1):11–25.
- Gunstad J, Spitznagel MB, Paul RH, Cohen RA, Kohn M, Luyster FS, Clark R, Williams LM, Gordon E. Body mass index and neuropsychological function in healthy children and adolescents. Appetite. 2008; 5 (2):246–51. [ PubMed : 17761359 ]
- Hanson SL, Kraus RS. Women, sports, and science: Do female athletes have an advantage. Sociology of Education. 1998; 71 :93–110.
- Hatfield BD, Hillman CH. The psychophysiology of sport: A mechanistic understanding of the psychology of superior performance. In: Singer RN, Hausenblas HA, Janelle C, editors. In The handbook of research on sport psychology. 2nd. New York: John Wiley; 2001. pp. 362–386.
- Hillman CH, Snook EM, Jerome GJ. Acute cardiovascular exercise and executive control function. International Journal of Psychophysiology. 2003; 48 (3):307–314. [ PubMed : 12798990 ]
- Hillman CH, Castelli DM, Buck SM. Aerobic fitness and neurocognitive function in healthy preadolescent children. Medicine and Science in Sports and Exercise. 2005; 37 (11):1967. [ PubMed : 16286868 ]
- Hillman CH, Motl RW, Pontifex MB, Posthuma D, Stubbe JH, Boomsma DI, De Geus EJC. Physical activity and cognitive function in a cross-section of younger and older community-dwelling individuals. Health Psychology. 2006; 25 (6):678. [ PubMed : 17100496 ]
- Hillman CH, Erickson KI, Kramer AF. Be smart, exercise your heart: Exercise effects on brain and cognition. Nature Reviews Neuroscience. 2008; 9 (1):58–65. [ PubMed : 18094706 ]
- Hillman CH, Pontifex MB, Raine LB, Castelli DM, Hall EE, Kramer AF. The effect of acute treadmill walking on cognitive control and academic achievement in preadolescent children. Neuroscience. 2009; 159 (3):1044. [ PMC free article : PMC2667807 ] [ PubMed : 19356688 ]
- Holroyd CB, Coles MG. The neural basis of human error processing: Reinforcement learning, dopamine, and the error-related negativity. Psychological Review. 2002; 109 (4):679. [ PubMed : 12374324 ]
- Huttenlocher PR, Dabholkar AS. Regional differences in synaptogenesis in human cerebral cortex. Journal of Comparative Neurology. 1997; 387 (2):167–178. [ PubMed : 9336221 ]
- Ila AB, Polich J. P300 and response time from a manual Stroop task. Clinical Neurophysiology. 1999; 110 (2):367–373. [ PubMed : 10210626 ]
- Jarrett OS, Maxwell DM, Dickerson C, Hoge P, Davies G, Yetley A. Impact of recess on classroom behavior: Group effects and individual differences. Journal of Educational Research. 1998; 92 (2):121–126.
- Jones JG, Hardy L. Stress and cognitive functioning in sport. Journal of Sports Sciences. 1989; 7 (1):41–63. [ PubMed : 2659817 ]
- Judge S, Jahns L. Association of overweight with academic performance and social and behavioral problems: An update from the Early Childhood Longitudinal Study. Journal of School Health. 2007; 77 :672–678. [ PubMed : 18076412 ]
- Kamijo K, Pontifex MB, O'Leary KC, Scudder MR, Wu CT, Castelli DM, Hillman CH. The effects of an afterschool physical activity program on working memory in preadolescent children. Developmental Science. 2011; 14 (5):1046–1058. [ PMC free article : PMC3177170 ] [ PubMed : 21884320 ]
- Kamijo K, Khan NA, Pontifex MB, Scudder MR, Drollette ES, Raine LB, Evans EM, Castelli DM, Hillman CH. The relation of adiposity to cognitive control and scholastic achievement in preadolescent children. Obesity. 2012a; 20 (12):2406–2411. [ PMC free article : PMC3414677 ] [ PubMed : 22546743 ]
- Kamijo K, Pontifex MB, Khan NA, Raine LB, Scudder MR, Drollette ES, Evans EM, Castelli DM, Hillman CH. The association of childhood obesity to neuroelectric indices of inhibition. Psychophysiology. 2012b; 49 (10):1361–1371. [ PubMed : 22913478 ]
- Kamijo K, Pontifex MB, Khan NA, Raine LB, Scudder MR, Drollette ES, Evans EM, Castelli DM, Hillman CH. Cerebral Cortex. 2012c. [October 4, 2013]. The negative association of childhood obesity to the cognitive control of action monitoring. Epub ahead of print, November 11. cercor .oxfordjournals .org/content/early/2012/11/09/cercor .bhs349.long . [ PMC free article : PMC3920765 ] [ PubMed : 23146965 ]
- Kibbe DL, Hackett J, Hurley M, McFarland A, Schubert KG, Schultz A, Harris S. Ten years of TAKE 10! ® : Integrating physical activity with academic concepts in elementary school classrooms. Preventive Medicine. 2011; 52 (Suppl):S43–S50. [ PubMed : 21281670 ]
- Kramer AF, Erickson KI. Capitalizing on cortical plasticity: Influence of physical activity on cognition and brain function. Trends in Cognitive Sciences. 2007; 11 (8):342–348. [ PubMed : 17629545 ]
- Kramer AF, Hahn S, Cohen NJ, Banich MT, McAuley E, Harrison CR, Chason J, Vakil E, Bardell L, Boileau RA. Ageing, fitness and neurocognitive function. Nature. 1999; 400 (6743):418–419. [ PubMed : 10440369 ]
- Kutas M, McCarthy G, Donchin E. Augmenting mental chronometry: The P300 as a measure of stimulus evaluation time. Science. 1977; 197 (4305):792–795. [ PubMed : 887923 ]
- London RA, Castrechini S. A longitudinal examination of the link between youth physical fitness and academic achievement. Journal of School Health. 2011; 81 (7):400–408. [ PubMed : 21668880 ]
- MacDonald AW, Cohen JD, Stenger VA, Carter CS. Dissociating the role of the dorsolateral prefrontal and anterior cingulate cortex in cognitive control. Science. 2000; 288 (5472):1835–1838. [ PubMed : 10846167 ]
- Magliero A, Bashore TR, Coles MG, Donchin E. On the dependence of P300 latency on stimulus evaluation processes. Psychophysiology. 1984; 21 (2):171–186. [ PubMed : 6728983 ]
- Mahar MT, Murphy SK, Rowe DA, Golden J, Shields AT, Raedeke TD. Effects of a classroom-based program on physical activity and on-task behavior. Medicine and Science in Sports and Exercise. 2006; 38 (12):2086. [ PubMed : 17146314 ]
- Mechanic D, Hansell S. Adolescent competence, psychological well-being, and self-assessed physical health. Journal of Health and Social Behavior. 1987; 28 (4):364–374. [ PubMed : 3429806 ]
- Mezzacappa E. Alerting, orienting, and executive attention: Developmental properties and sociodemographic correlates in an epidemiological sample of young, urban children. Child Development. 2004; 75 (5):1373–1386. [ PubMed : 15369520 ]
- Miller KE, Melnick MJ, Barnes GM, Farrell MP, Sabo D. Untangling the links among the athletic involvement, gender, race, and adolescent academic outcomes. Sociology of Sport. 2005; 22 (2):178–193. [ PMC free article : PMC1343519 ] [ PubMed : 16467902 ]
- Monti JM, Hillman CH, Cohen NJ. Aerobic fitness enhances relational memory in preadolescent children: The FITKids randomized control trial. Hippocampus. 2012; 22 (9):1876–1882. [ PMC free article : PMC3404196 ] [ PubMed : 22522428 ]
- Münte TF, Kohlmetz C, Nager W, Altenmüller E. Superior auditory spatial tuning in conductors. Nature. 2001; 409 (6820):580. [ PubMed : 11214309 ]
- NASPE (National Association for Sport and Physical Education). Moving into the future: National Physical Education Content Standards. 2nd. Reston, VA: NASPE; 2004.
- NASPE. Recess for elementary school students. 2006. [December 1, 2012]. http://www .aahperd.org /naspe/standards/upload /recess-for-elementary-school-students-2006.pdf .
- Neeper SA, Gomez-Pinilla F, Choi J, Cotman C. Exercise and brain neuro-trophins. Nature. 1995; 373 (6510):109. [ PubMed : 7816089 ]
- NRC (National Research Council)/IOM (Institute of Medicine). From neurons to neighborhoods: The science of early childhood development. Washington, DC: National Academy Press; 2000. [ PubMed : 25077268 ]
- O'Leary KC, Pontifex MB, Scudder MR, Brown ML, Hillman CH. The effects of single bouts of aerobic exercise, exergaming, and videogame play on cognitive control. Clinical Neurophysiology. 2011; 122 (8):1518–1525. [ PubMed : 21353635 ]
- Page RM, Hammermeister J, Scanlan A, Gilbert L. Is school sports participation a protective factor against adolescent health risk behaviors. Journal of Health Education. 1998; 29 (3):186–192.
- Pellegrini AD, Bohn CM. The role of recess in children's cognitive performance and school adjustment. Educational Researcher. 2005; 34 (1):13–19.
- Pellegrini AD, Huberty PD, Jones I. The effects of recess timing on children's playground and classroom behaviors. American Educational Research Journal. 1995; 32 (4):845–864.
- Pesce C, Crova C, Cereatti L, Casella R, Bellucci M. Physical activity and mental performance in preadolescents: Effects of acute exercise on free-recall memory. Mental Health and Physical Activity. 2009; 2 (1):16–22.
- Polich J. EEG and ERP assessment of normal aging. Electroencephalography and Clinical Neurophysiology/Evoked Potentials Section. 1997; 104 (3):244–256. [ PubMed : 9186239 ]
- Polich J, Heine MR. P300 topography and modality effects from a single-stimulus paradigm. Psychophysiology. 2007; 33 (6):747–752. [ PubMed : 8961797 ]
- Pontifex MB, Raine LB, Johnson CR, Chaddock L, Voss MW, Cohen NJ, Kramer AF, Hillman CH. Cardiorespiratory fitness and the flexible modulation of cognitive control in preadolescent children. Journal of Cognitive Neuroscience. 2011; 23 (6):1332–1345. [ PubMed : 20521857 ]
- Pontifex MB, Scudder MR, Drollette ES, Hillman CH. Fit and vigilant: The relationship between sedentary behavior and failures in sustained attention during preadolescence. Neuropsychology. 2012; 26 (4):407–413. [ PMC free article : PMC3390762 ] [ PubMed : 22746307 ]
- Pontifex MB, Saliba BJ, Raine LB, Picchietti DL, Hillman CH. Exercise improves behavioral, neurophysiologic, and scholastic performance in children with ADHD. Journal of Pediatrics. 2013; 162 :543–551. [ PMC free article : PMC3556380 ] [ PubMed : 23084704 ]
- Raji CA, Ho AJ, Parikshak NN, Becker JT, Lopez OL, Kuller LH, Hua X, Leow AD, Toga AW, Thompson PM. Brain structure and obesity. Human Brain Mapping. 2010; 31 (3):353–364. [ PMC free article : PMC2826530 ] [ PubMed : 19662657 ]
- Rasberry CN, Lee SM, Robin L, Laris BA, Russell LA, Coyle KK, Nihiser AJ. The association between school-based physical activity, including physical education, and academic performance: A systematic review of the literature. Preventive Medicine. 2011; 52 (Suppl 1):S10–S20. [ PubMed : 21291905 ]
- Raz N. Aging of the brain and its impact on cognitive performance: Integration of structural and functional findings. In: Craik FM, Salthouse TA, editors. In The handbook of aging and cognition. Vol. 2. Mahweh, NJ: Lawrence Erlbaum Associates; 2000. pp. 1–90.
- Reed JA, Einstein G, Hahn E, Hooker SP, Gross VP, Kravitz J. Examining the impact of integrating physical activity on fluid intelligence and academic performance in an elementary school setting: A preliminary investigation. Journal of Physical Activity and Health. 2010; 7 (3):343–351. [ PubMed : 20551490 ]
- Ruiz JR, Ortega FB, Castillo R, Martin-Matillas M, Kwak L, Vicente-Rodriguez G, Noriega J, Tercedor P, Sjostrom M, Moreno LA. Journal of Pediatrics. 2010; 157 (6):917–922. [ PubMed : 20673915 ]
- Sallis JF, McKenzie TL, Kolody B, Lewis M, Marshall S, Rosengard P. Effects of health-related physical education on academic achievement: Project SPARK. Research Quarterly for Exercise and Sport. 1999; 70 (2):127–134. [ PubMed : 10380244 ]
- Sanders A. Towards a model of stress and human performance. Acta Psychologica. 1983; 53 (1):61–97. [ PubMed : 6869047 ]
- Shephard RJ. Habitual physical activity and academic performance. Nutrition Reviews. 1986; 54 (4):S32–S36. [ PubMed : 8700451 ]
- Shephard RJ, Volle M, Lavallee H, LaBarre R, Jequier J, Rajic M. In Children and Sport. Berlin, Germany: Springer-Verlag; 1984. Required physical activity and academic grades: A controlled study; pp. 58–63.
- Sibley BA, Etnier JL. The relationship between physical activity and cognition in children: A meta-analysis. Pediatric Exercise Science. 2003; 15 :243–256.
- Silliker SA, Quirk JT. The effect of extracurricular activity participation on the academic performance of male and female high school students. School Counselor. 1997; 44 (4):288–293.
- Singh A, Uijtdewilligen L, Twisk JWR, van Mechelen W, Chinapaw MJM. Physical activity and performance at school: A systematic review of the literature including a methodological quality assessment. Archives of Pediatrics and Adolescent Medicine. 2012; 166 (1):49–55. [ PubMed : 22213750 ]
- Sirin SR. Socioeconomic status and academic achievement: A meta-analytic review of research. Review of Educational Research. 2005; 75 (3):417–453.
- Smith PJ, Blumenthal JA, Hoffman BM, Cooper H, Strauman TA, Welsh-Bohmer K, Browndyke JN, Sherwood A. Aerobic exercise and neuro-cognitive performance: A meta-analytic review of randomized controlled trials. Psychosomatic Medicine. 2010; 72 (3):239–252. [ PMC free article : PMC2897704 ] [ PubMed : 20223924 ]
- Stanca L. The effects of attendance on academic performance: Panel data evidence for introductory microeconomics. Journal of Economic Education. 2006; 37 (3):251–266.
- Stephens LJ, Schaben LA. The effect of interscholastic sports participation on academic achievement of middle level school activities. National Association of Secondary School Principals Bulletin. 2002; 86 :34–42.
- Stewart JA, Dennison DA, Kohl HW III, Doyle JA. Exercise level and energy expenditure in the TAKE 10! ® in-class physical activity program. Journal of School Health. 2004; 74 (10):397–400. [ PubMed : 15724566 ]
- Strong WB, Malina RM, Blimkie CJ, Daniels SR, Dishman RK, Gutin B, Hergenroeder AC, Must A, Nixon PA, Pivarnik JM, Rowland T, Trost S, Trudeau F. Evidence based physical activity for school-age youth. Journal of Pediatrics. 2005; 146 (6):732–737. [ PubMed : 15973308 ]
- Taliaferro LA, Rienzo BA, Donovan KA. Relationships between youth sport participation and selected health risk behaviors from 1999 to 2007. Journal of School Health. 2010; 80 (8):399–410. [ PubMed : 20618623 ]
- Taylor MJ. Neural bases of cognitive development. In: Bialystok E, Craik FIM, editors. In Lifespan cognition: Mechanisms of change. Oxford, UK: Oxford University Press; 2006. pp. 15–26.
- Telama R, Yang X, Laakso L, Viikari J. Physical activity in childhood and adolescence as predictor of physical activity in young adulthood. American Journal of Preventive Medicine. 1997; 13 (4):317–323. [ PubMed : 9236971 ]
- Thomas AG, Dennis A, Bandettini PA, Johansen-Berg H. The effects of aerobic activity on brain structure. Frontiers in Psychology. 2012; 3 :1–9. [ PMC free article : PMC3311131 ] [ PubMed : 22470361 ]
- Tomporowski PD. Effects of acute bouts of exercise on cognition. Acta Psychologica. 2003; 112 (3):297–324. [ PubMed : 12595152 ]
- Tomporowski PD, Davis CL, Miller PH, Naglieri JA. Exercise and children's intelligence, cognition, and academic achievement. Educational Psychology Review. 2008a; 20 (2):111–131. [ PMC free article : PMC2748863 ] [ PubMed : 19777141 ]
- Tomporowski PD, Davis CL, Lambourne K, Gregoskis M, Tkacz J. Task switching in overweight children: Effects of acute exercise and age. Journal of Sport and Exercise Psychology. 2008b; 30 (5):497–511. [ PMC free article : PMC2705951 ] [ PubMed : 18971509 ]
- Trudeau F, Shephard RJ. Physical education, school physical activity, school sports and academic performance. International Journal of Behavioral Nutrition and Physical Activity. 2008; 5 [ PMC free article : PMC2329661 ] [ PubMed : 18298849 ]
- Trudeau F, Shephard RJ. Relationships of physical activity to brain health and the academic performance of school children. American Journal of Lifestyle Medicine. 2010; 4 :138–150.
- Trudeau F, Laurencelle L, Tremblay J, Rajic M, Shephard R. Daily primary school physical education: Effects on physical activity during adult life. Medicine and Science in Sports and Exercise. 1999; 31 (1):111. [ PubMed : 9927018 ]
- Trudeau F, Shephard RJ, Arsenault F, Laurencelle L. Changes in adiposity and body mass index from late childhood to adult life in the Trois-Rivières study. American Journal of Human Biology. 2001; 13 (3):349–355. [ PubMed : 11460900 ]
- Trudeau F, Laurencelle L, Shephard RJ. Tracking of physical activity from childhood to adulthood. Medicine and Science in Sports and Exercise. 2004; 36 (11):1937. [ PubMed : 15514510 ]
- Van Dusen DP, Kelder SH, Kohl HW III, Ranjit N, Perry CL. Associations of physical fitness and academic performance among schoolchildren. Journal of School Health. 2011; 81 (12):733–740. [ PubMed : 22070504 ]
- Van Praag H, Kempermann G, Gage FH. Running increases cell proliferation and neurogenesis in the adult mouse dentate gyrus. Nature Neuroscience. 1999; 2 (3):266–270. [ PubMed : 10195220 ]
- Voss MW, Chaddock L, Kim JS, VanPatter M, Pontifex MB, Raine LB, Cohen NJ, Hillman CH, Kramer AF. Aerobic fitness is associated with greater efficiency of the network underlying cognitive control in preadolescent children. Neuroscience. 2011; 199 :166–176. [ PMC free article : PMC3237764 ] [ PubMed : 22027235 ]
- Wechsler H, Brener ND, Kuester S, Miller C. Food service and food and beverage available at school: Results from the School Health Policies and Programs Study. Journal of School Health. 2001; 71 (7):313–324. [ PubMed : 11586874 ]
- Welk GJ, Jackson AW, Morrow J, James R, Haskell WH, Meredith MD, Cooper KH. The association of health-related fitness with indicators of academic performance in Texas schools. Research Quarterly for Exercise and Sport. 2010; 81 (Suppl 2):16S–23S. [ PubMed : 21049834 ]
- Welk GJ, Going SB, Morrow JR, Meredith MD. Development of new criterion-referenced fitness standards in the Fitnessgram ® program. American Journal of Preventive Medicine. 2011; 41 (2):6. [ PubMed : 21961614 ]
- Wilkins J, Graham G, Parker S, Westfall S, Fraser R, Tembo M. Time in the arts and physical education and school achievement. Journal of Curriculum Studies. 2003; 35 (6):721–734.
- Wittberg R, Cottrell LA, Davis CL, Northrup KL. Aerobic fitness thresholds associated with fifth grade academic achievement. American Journal of Health Education. 2010; 41 (5):284–291.
- Yeung N, Botvinick MM, Cohen JD. The neural basis of error detection: Conflict monitoring and the error-related negativity. Psychological Review. 2004; 111 (4):931. [ PubMed : 15482068 ]
- Zhu W, Welk GJ, Meredith MD, Boiarskaia EA. A survey of physical education programs and policies in Texas schools. Research Quarterly for Exercise and Sport. 2010; 81 (Suppl 2):42S–52S. [ PubMed : 21049837 ]
- Cite this Page Committee on Physical Activity and Physical Education in the School Environment; Food and Nutrition Board; Institute of Medicine; Kohl HW III, Cook HD, editors. Educating the Student Body: Taking Physical Activity and Physical Education to School. Washington (DC): National Academies Press (US); 2013 Oct 30. 4, Physical Activity, Fitness, and Physical Education: Effects on Academic Performance.
- PDF version of this title (4.4M)
In this Page
Related information.
- PMC PubMed Central citations
- PubMed Links to PubMed
Recent Activity
- Physical Activity, Fitness, and Physical Education: Effects on Academic Performa... Physical Activity, Fitness, and Physical Education: Effects on Academic Performance - Educating the Student Body
Your browsing activity is empty.
Activity recording is turned off.
Turn recording back on
Connect with NLM
National Library of Medicine 8600 Rockville Pike Bethesda, MD 20894
Web Policies FOIA HHS Vulnerability Disclosure
Help Accessibility Careers
24/7 writing help on your phone
To install StudyMoose App tap and then “Add to Home Screen”
The Importance of Physical Education in Schools
Save to my list
Remove from my list
Introduction
Benefits of physical education, challenges and solutions.

Fostering Lifelong Healthy Habits
Integration with overall education.
The Importance of Physical Education in Schools. (2024, Feb 21). Retrieved from https://studymoose.com/the-importance-of-physical-education-in-schools-essay
"The Importance of Physical Education in Schools." StudyMoose , 21 Feb 2024, https://studymoose.com/the-importance-of-physical-education-in-schools-essay
StudyMoose. (2024). The Importance of Physical Education in Schools . [Online]. Available at: https://studymoose.com/the-importance-of-physical-education-in-schools-essay [Accessed: 31 Aug. 2024]
"The Importance of Physical Education in Schools." StudyMoose, Feb 21, 2024. Accessed August 31, 2024. https://studymoose.com/the-importance-of-physical-education-in-schools-essay
"The Importance of Physical Education in Schools," StudyMoose , 21-Feb-2024. [Online]. Available: https://studymoose.com/the-importance-of-physical-education-in-schools-essay. [Accessed: 31-Aug-2024]
StudyMoose. (2024). The Importance of Physical Education in Schools . [Online]. Available at: https://studymoose.com/the-importance-of-physical-education-in-schools-essay [Accessed: 31-Aug-2024]
- Importance of Physical Education in Schools Pages: 7 (2072 words)
- Mandatory Physical Education In Schools Pages: 7 (1993 words)
- Physical Education In Schools: Pros And Cons Pages: 2 (502 words)
- The Importance of Sex Education in Today's Schools Pages: 8 (2368 words)
- Public Schools vs Private Schools: Pros & Cons Research Pages: 8 (2371 words)
- Public Schools vs. Private Schools Pages: 2 (443 words)
- Private Schools vs Public Schools Pages: 1 (297 words)
- Single Sex schools and Mixed Schools Pages: 2 (566 words)
- Relation of Physical Education to the Personality Development of the Students Pages: 10 (2876 words)
- Sport Science, Coaching and Physical Education Pages: 6 (1570 words)
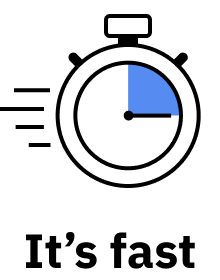
👋 Hi! I’m your smart assistant Amy!
Don’t know where to start? Type your requirements and I’ll connect you to an academic expert within 3 minutes.
Promotion of Physical Activity and Health in the School Setting
- © 2024
- Antonio García-Hermoso ORCID: https://orcid.org/0000-0002-1397-7182 0
Navarrabiomed, Hospital Universitario de Navarra, Universidad Pública de Navarra (UPNA), IdiSNA, Pamplona, Spain
You can also search for this editor in PubMed Google Scholar
- Discusses new approaches to promote physical activity and sports in the school environment
- Presents activities to help children feel strong and enjoy lifelong physical activity
- Shows how to plan, implement, evaluate and monitor the promotion of physical activity of school children and adolescents
991 Accesses
38 Altmetric
This is a preview of subscription content, log in via an institution to check access.
Access this book
Subscribe and save.
- Get 10 units per month
- Download Article/Chapter or eBook
- 1 Unit = 1 Article or 1 Chapter
- Cancel anytime
- Available as EPUB and PDF
- Read on any device
- Instant download
- Own it forever
- Durable hardcover edition
- Dispatched in 3 to 5 business days
- Free shipping worldwide - see info
Tax calculation will be finalised at checkout
Other ways to access
Licence this eBook for your library
Institutional subscriptions
About this book
This book is the first-known contributed volume focused exclusively on physical activity in the school setting. It describes the importance of regular physical activity for all preschoolers, children and adolescents, which contributes to preventing concerning public health problems such as childhood obesity and physical inactivity.
Children and adolescents spend more time in school than anywhere else other than the home, making schools an excellent setting in which to offer quality physical activity education and possibilities for an active school day. Physical activity is associated with physiological, physical, and mental health benefits. School-based physical activity initiatives can also positively impact learning and classroom behavior.
This book comprehensively reviews the latest research on physical activity and health in the school setting, presenting different perspectives from prestigious international authors representing 12 countries and involving 40 different researchers. It provides an in-depth description on how the school environment can be used to develop, implement, and evaluate strategies that promote physical activity and reduce sedentary behavior among these populations according to the educational stage (i.e., pre-primary, primary and secondary school). It also details how to implement each individual initiative based on successful investigations and how to plan, implement and evaluate a school policy. By implementing these strategies, schools can help create a culture of physical activity that promotes healthy habits and reduces the risk of chronic diseases.
The volume's 16 chapters are organized in three parts, providing an overview of current knowledge. The authors discuss advances in theory and research and demonstrate how they are using that evidence to improve professional practice and collaborate with families.
- Part I: Movement Guidelines and Recommendations
- Part II: Monitoring and Evaluation in School Settings
- Part III: Physical Activity During School Hours
Promotion of Physical Activity and Health in the School Setting makes an important contribution to the youth physical activity field for scholars and practitioners. The book is essential reading for advanced students, researchers, practitioners, and policy-makers with an interest in physical activity, youth sport, public health, physical education, or child and adolescent development and education.
- Physical activity and sports in schools
- Healthy schools
- Active school evaluation
- HIIT (high-intensity interval training programs) at schools
- Health-related physical fitness assessment in schools
- Active travel to and from school
- Multi-component school-based physical activity programs
- Muscle-strengthening activities at schools
- Health promotion in the school setting
- School fitness tests
- Recess and recreation time
- Commuting to school
- Physical activity and health guidelines and recommendations
- School-aged children and adolescents
- Motor skill assessment
- Physical literacy assessment
- Active classrooms in school curricula
- Inclusive physical activity for youth with special needs
- Active school activities and breaks
- Active learning
Table of contents (16 chapters)
Front matter, movement guidelines and recommendations, physical activity and sedentary behavior in children and adolescents: recommendations and health impacts.
- Yang Liu, Danqing Zhang, Youzhi Ke, Yiping Yan, Yangyang Shen, Zhenghan Wang
Twenty-Four-Hour Movement Behaviors for School-Aged Children and Adolescents
- José Francisco López-Gil
Assessment and Evaluation of Physical Health and Skills in School Settings
Physical literacy assessment: a conceptualization and tools.
- Andreas Fröberg, Suzanne Lundvall
Assessment of Physical Activity in Children and Adolescents
- Jairo H. Migueles, Patricio Solis-Urra
Health-Related Physical Fitness Assessment in School Settings
- Kai Zhang, Cristina Cadenas-Sanchez, Brooklyn Fraser, Justin J. Lang
Motor Skill Assessment in Children and Adolescents
- Nadia Cristina Valentini
Physical Activity During School Hours
Physical activity and health through physical education.
- Adrià Muntaner-Mas
Active Travel to and from School
- Adilson Marques, Tiago Ribeiro, Miguel Peralta
Physical Activity Opportunities During School Recess
Antonio García-Hermoso
Active Classrooms in School Curricula and Active Breaks
- Abel Ruiz-Hermosa, David Sánchez-Oliva, Mairena Sánchez-López
Multicomponent School-Based Physical Activity Programs
- Collin A. Webster
School-Based Before-School Physical Activity Programs
- Michalis Stylianou, James Woodforde
School-Based After-School Physical Activity and Sports Programs
- Hyungsik Min, Donetta Cothran, Pamela Hodges Kulinna
Integrating High-Intensity Interval Training (HIIT) into the School Setting: Benefits, Criticisms, and Recommendations
- Angus A. Leahy, Jordan J. Smith, Narelle Eather, Nigel Harris, David R. Lubans
How Can Muscle-Strengthening Activities Be Promoted in School Settings?
Inclusive physical activity practices for disabled children and adolescents.
- Thi Nancy Huynh, Justin Haegele, Maeghan E. James, Kelly P. Arbour-Nicitopoulos
Editors and Affiliations
About the editor.
Antonio García-Hermoso, PhD, MSc , is a native of Plasencia, Spain, and a graduate of the University of Extremadura, Spain. Since 2019, Dr. García-Hermoso has been a member of the Navarrabiomed Research Center in Pamplona, Spain, where he serves as the head of the Physical Activity, Children, and Youth Unit. This unit is dedicated to analyzing the impact of exercise and physical activity on the physical and mental health of both ill and apparently healthy young people.
Dr. García-Hermoso has contributed to over 300 publications in peer-reviewed and PubMed-listed journals. He is a member of several international professional societies and serves on the editorial boards of Translational Pediatrics journal and The Scandinavian Journal of Medicine & Science in Sports .
Bibliographic Information
Book Title : Promotion of Physical Activity and Health in the School Setting
Editors : Antonio García-Hermoso
DOI : https://doi.org/10.1007/978-3-031-65595-1
Publisher : Springer Cham
eBook Packages : Medicine , Medicine (R0)
Copyright Information : The Editor(s) (if applicable) and The Author(s), under exclusive license to Springer Nature Switzerland AG 2024
Hardcover ISBN : 978-3-031-65594-4 Published: 25 August 2024
Softcover ISBN : 978-3-031-65597-5 Due: 08 September 2025
eBook ISBN : 978-3-031-65595-1 Published: 24 August 2024
Edition Number : 1
Number of Pages : XV, 387
Number of Illustrations : 1 b/w illustrations, 18 illustrations in colour
Topics : Health Promotion and Disease Prevention , Sport Education and Didactics , Sport Science , Health Policy , Education, general
- Publish with us
Policies and ethics
- Find a journal
- Track your research
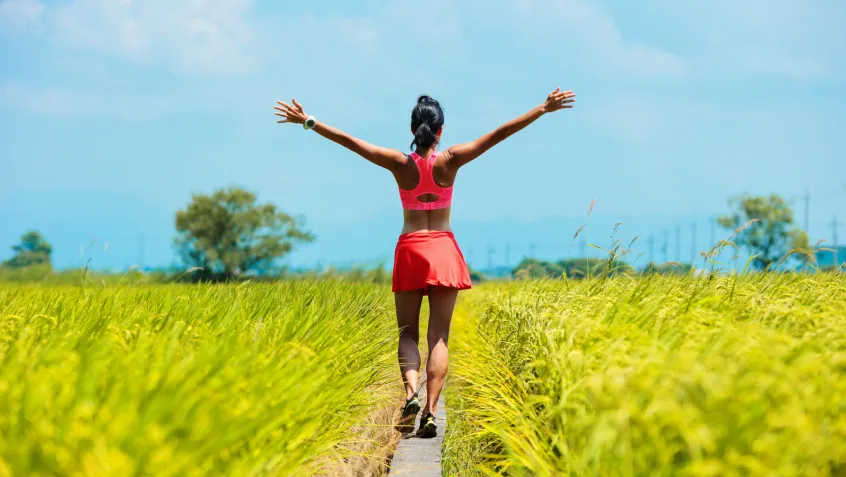

Similar content being viewed by others
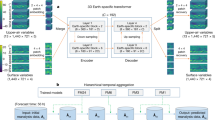
Accurate medium-range global weather forecasting with 3D neural networks
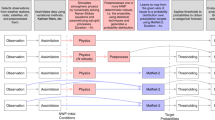
Deep learning for twelve hour precipitation forecasts
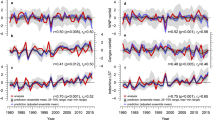
Skilful predictions of the Asian summer monsoon one year ahead
Solving the equations for Earth’s atmosphere with general circulation models (GCMs) is the basis of weather and climate prediction 1 , 2 . Over the past 70 years, GCMs have been steadily improved with better numerical methods and more detailed physical models, while exploiting faster computers to run at higher resolution. Inside GCMs, the unresolved physical processes such as clouds, radiation and precipitation are represented by semi-empirical parameterizations. Tuning GCMs to match historical data remains a manual process 5 , and GCMs retain many persistent errors and biases 6 , 7 , 8 . The difficulty of reducing uncertainty in long-term climate projections 9 and estimating distributions of extreme weather events 10 presents major challenges for climate mitigation and adaptation 11 .
Recent advances in machine learning have presented an alternative for weather forecasting 3 , 4 , 12 , 13 . These models rely solely on machine-learning techniques, using roughly 40 years of historical data from the European Center for Medium-Range Weather Forecasts (ECMWF) reanalysis v5 (ERA5) 14 for model training and forecast initialization. Machine-learning methods have been remarkably successful, demonstrating state-of-the-art deterministic forecasts for 1- to 10-day weather prediction at a fraction of the computational cost of traditional models 3 , 4 . Machine-learning atmospheric models also require considerably less code, for example GraphCast 3 has 5,417 lines versus 376,578 lines for the National Oceanic and Atmospheric Administration’s FV3 atmospheric model 15 (see Supplementary Information section A for details).
Nevertheless, machine-learning approaches have noteworthy limitations compared with GCMs. Existing machine-learning models have focused on deterministic prediction, and surpass deterministic numerical weather prediction in terms of the aggregate metrics for which they are trained 3 , 4 . However, they do not produce calibrated uncertainty estimates 4 , which is essential for useful weather forecasts 1 . Deterministic machine-learning models using a mean-squared-error loss are rewarded for averaging over uncertainty, producing unrealistically blurry predictions when optimized for multi-day forecasts 3 , 13 . Unlike physical models, machine-learning models misrepresent derived (diagnostic) variables such as geostrophic wind 16 . Furthermore, although there has been some success in using machine-learning approaches on longer timescales 17 , 18 , these models have not demonstrated the ability to outperform existing GCMs.
Hybrid models that combine GCMs with machine learning are appealing because they build on the interpretability, extensibility and successful track record of traditional atmospheric models 19 , 20 . In the hybrid model approach, a machine-learning component replaces or corrects the traditional physical parameterizations of a GCM. Until now, the machine-learning component in such models has been trained ‘offline’, by learning parameterizations independently of their interaction with dynamics. These components are then inserted into an existing GCM. The lack of coupling between machine-learning components and the governing equations during training potentially causes serious problems, such as instability and climate drift 21 . So far, hybrid models have mostly been limited to idealized scenarios such as aquaplanets 22 , 23 . Under realistic conditions, machine-learning corrections have reduced some biases of very coarse GCMs 24 , 25 , 26 , but performance remains considerably worse than state-of-the-art models.
Here we present NeuralGCM, a fully differentiable hybrid GCM of Earth’s atmosphere. NeuralGCM is trained on forecasting up to 5-day weather trajectories sampled from ERA5. Differentiability enables end-to-end ‘online training’ 27 , with machine-learning components optimized in the context of interactions with the governing equations for large-scale dynamics, which we find enables accurate and stable forecasts. NeuralGCM produces physically consistent forecasts with accuracy comparable to best-in-class models across a range of timescales, from 1- to 15-day weather to decadal climate prediction.
Neural GCMs
A schematic of NeuralGCM is shown in Fig. 1 . The two key components of NeuralGCM are a differentiable dynamical core for solving the discretized governing dynamical equations and a learned physics module that parameterizes physical processes with a neural network, described in full detail in Methods , Supplementary Information sections B and C , and Supplementary Table 1 . The dynamical core simulates large-scale fluid motion and thermodynamics under the influence of gravity and the Coriolis force. The learned physics module (Supplementary Fig. 1 ) predicts the effect of unresolved processes, such as cloud formation, radiative transport, precipitation and subgrid-scale dynamics, on the simulated fields using a neural network.
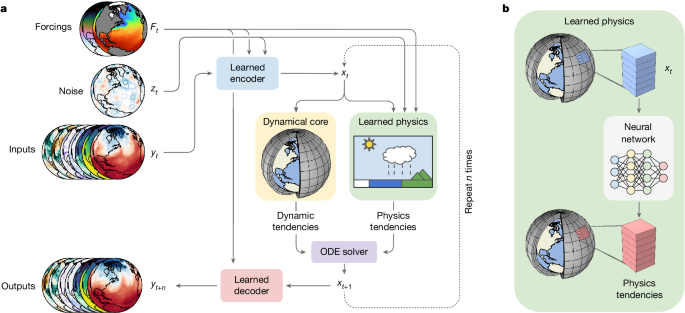
a , Overall model structure, showing how forcings F t , noise z t (for stochastic models) and inputs y t are encoded into the model state x t . The model state is fed into the dynamical core, and alongside forcings and noise into the learned physics module. This produces tendencies (rates of change) used by an implicit–explicit ordinary differential equation (ODE) solver to advance the state in time. The new model state x t +1 can then be fed back into another time step, or decoded into model predictions. b , The learned physics module, which feeds data for individual columns of the atmosphere into a neural network used to produce physics tendencies in that vertical column.
The differentiable dynamical core in NeuralGCM allows an end-to-end training approach, whereby we advance the model multiple time steps before employing stochastic gradient descent to minimize discrepancies between model predictions and reanalysis (Supplementary Information section G.2 ). We gradually increase the rollout length from 6 hours to 5 days (Supplementary Information section G and Supplementary Table 5 ), which we found to be critical because our models are not accurate for multi-day prediction or stable for long rollouts early in training (Supplementary Information section H.6.2 and Supplementary Fig. 23 ). The extended back-propagation through hundreds of simulation steps enables our neural networks to take into account interactions between the learned physics and the dynamical core. We train deterministic and stochastic NeuralGCM models, each of which uses a distinct training protocol, described in full detail in Methods and Supplementary Table 4 .
We train a range of NeuralGCM models at horizontal resolutions with grid spacing of 2.8°, 1.4° and 0.7° (Supplementary Fig. 7 ). We evaluate the performance of NeuralGCM at a range of timescales appropriate for weather forecasting and climate simulation. For weather, we compare against the best-in-class conventional physics-based weather models, ECMWF’s high-resolution model (ECMWF-HRES) and ensemble prediction system (ECMWF-ENS), and two of the recent machine-learning-based approaches, GraphCast 3 and Pangu 4 . For climate, we compare against a global cloud-resolving model and Atmospheric Model Intercomparison Project (AMIP) runs.
Medium-range weather forecasting
Our evaluation set-up focuses on quantifying accuracy and physical consistency, following WeatherBench2 12 . We regrid all forecasts to a 1.5° grid using conservative regridding, and average over all 732 forecasts made at noon and midnight UTC in the year 2020, which was held-out from training data for all machine-learning models. NeuralGCM, GraphCast and Pangu compare with ERA5 as the ground truth, whereas ECMWF-ENS and ECMWF-HRES compare with the ECMWF operational analysis (that is, HRES at 0-hour lead time), to avoid penalizing the operational forecasts for different biases than ERA5.
Model accuracy
We use ECMWF’s ensemble (ENS) model as a reference baseline as it achieves the best performance across the majority of lead times 12 . We assess accuracy using (1) root-mean-squared error (RMSE), (2) root-mean-squared bias (RMSB), (3) continuous ranked probability score (CRPS) and (4) spread-skill ratio, with the results shown in Fig. 2 . We provide more in-depth evaluations including scorecards, metrics for additional variables and levels and maps in Extended Data Figs. 1 and 2 , Supplementary Information section H and Supplementary Figs. 9 – 22 .
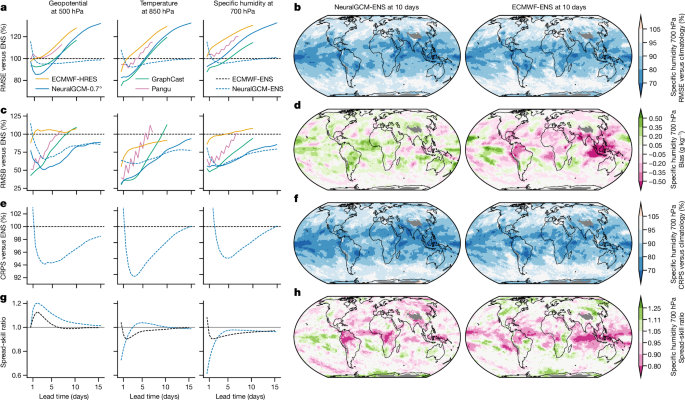
a , c , RMSE ( a ) and RMSB ( c ) for ECMWF-ENS, ECMWF-HRES, NeuralGCM-0.7°, NeuralGCM-ENS, GraphCast 3 and Pangu 4 on headline WeatherBench2 variables, as a percentage of the error of ECMWF-ENS. Deterministic and stochastic models are shown in solid and dashed lines respectively. e , g , CRPS relative to ECMWF-ENS ( e ) and spread-skill ratio for the ENS and NeuralGCM-ENS models ( g ). b , d , f , h , Spatial distributions of RMSE ( b ), bias ( d ), CRPS ( f ) and spread-skill ratio ( h ) for NeuralGCM-ENS and ECMWF-ENS models for 10-day forecasts of specific humidity at 700 hPa. Spatial plots of RMSE and CRPS show skill relative to a probabilistic climatology 12 with an ensemble member for each of the years 1990–2019. The grey areas indicate regions where climatological surface pressure on average is below 700 hPa.
Deterministic models that produce a single weather forecast for given initial conditions can be compared effectively using RMSE skill at short lead times. For the first 1–3 days, depending on the atmospheric variable, RMSE is minimized by forecasts that accurately track the evolution of weather patterns. At this timescale we find that NeuralGCM-0.7° and GraphCast achieve best results, with slight variations across different variables (Fig. 2a ). At longer lead times, RMSE rapidly increases owing to chaotic divergence of nearby weather trajectories, making RMSE less informative for deterministic models. RMSB calculates persistent errors over time, which provides an indication of how models would perform at much longer lead times. Here NeuralGCM models also compare favourably against previous approaches (Fig. 2c ), with notably much less bias for specific humidity in the tropics (Fig. 2d ).
Ensembles are essential for capturing intrinsic uncertainty of weather forecasts, especially at longer lead times. Beyond about 7 days, the ensemble means of ECMWF-ENS and NeuralGCM-ENS forecasts have considerably lower RMSE than the deterministic models, indicating that these models better capture the average of possible weather. A better metric for ensemble models is CRPS, which is a proper scoring rule that is sensitive to full marginal probability distributions 28 . Our stochastic model (NeuralGCM-ENS) running at 1.4° resolution has lower error compared with ECMWF-ENS across almost all variables, lead times and vertical levels for ensemble-mean RMSE, RSMB and CRPS (Fig. 2a,c,e and Supplementary Information section H ), with similar spatial patterns of skill (Fig. 2b,f ). Like ECMWF-ENS, NeuralGCM-ENS has a spread-skill ratio of approximately one (Fig. 2d ), which is a necessary condition for calibrated forecasts 29 .
An important characteristic of forecasts is their resemblance to realistic weather patterns. Figure 3 shows a case study that illustrates the performance of NeuralGCM on three types of important weather phenomenon: tropical cyclones, atmospheric rivers and the Intertropical Convergence Zone. Figure 3a shows that all the machine-learning models make significantly blurrier forecasts than the source data ERA5 and physics-based ECMWF-HRES forecast, but NeuralCGM-0.7° outperforms the pure machine-learning models, despite its coarser resolution (0.7° versus 0.25° for GraphCast and Pangu). Blurry forecasts correspond to physically inconsistent atmospheric conditions and misrepresent extreme weather. Similar trends hold for other derived variables of meteorological interest (Supplementary Information section H.2 ). Ensemble-mean predictions, from both NeuralGCM and ECMWF, are closer to ERA5 in an average sense, and thus are inherently smooth at long lead times. In contrast, as shown in Fig. 3 and in Supplementary Information section H.3 , individual realizations from the ECMWF and NeuralGCM ensembles remain sharp, even at long lead times. Like ECMWF-ENS, NeuralGCM-ENS produces a statistically representative range of future weather scenarios for each weather phenomenon, despite its eight-times-coarser resolution.
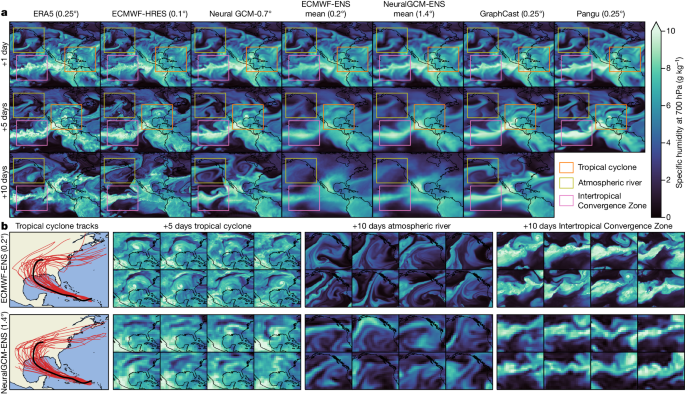
All forecasts are initialized at 2020-08-22T12z, chosen to highlight Hurricane Laura, the most damaging Atlantic hurricane of 2020. a , Specific humidity at 700 hPa for 1-day, 5-day and 10-day forecasts over North America and the Northeast Pacific Ocean from ERA5 14 , ECMWF-HRES, NeuralGCM-0.7°, ECMWF-ENS (mean), NeuralGCM-ENS (mean), GraphCast 3 and Pangu 4 . b , Forecasts from individual ensemble members from ECMWF-ENS and NeuralGCM-ENS over regions of interest, including predicted tracks of Hurricane Laura from each of the 50 ensemble members (Supplementary Information section I.2 ). The track from ERA5 is plotted in black.
We can quantify the blurriness of different forecast models via their power spectra. Supplementary Figs. 17 and 18 show that the power spectra of NeuralCGM-0.7° is consistently closer to ERA5 than the other machine-learning forecast methods, but is still blurrier than ECMWF’s physical forecasts. The spectra of NeuralGCM forecasts is also roughly constant over the forecast period, in stark contrast to GraphCast, which worsens with lead time. The spectrum of NeuralGCM becomes more accurate with increased resolution (Supplementary Fig. 22 ), which suggests the potential for further improvements of NeuralGCM models trained at higher resolutions.
Water budget
In NeuralGCM, advection is handled by the dynamical core, while the machine-learning parameterization models local processes within vertical columns of the atmosphere. Thus, unlike pure machine-learning methods, local sources and sinks can be isolated from tendencies owing to horizontal transport and other resolved dynamics (Supplementary Fig. 3 ). This makes our results more interpretable and facilitates the diagnosis of the water budget. Specifically, we diagnose precipitation minus evaporation (Supplementary Information section H.5 ) rather than directly predicting these as in machine-learning-based approaches 3 . For short weather forecasts, the mean of precipitation minus evaporation has a realistic spatial distribution that is very close to ERA5 data (Extended Data Fig. 4c–e ). The precipitation-minus-evaporation rate distribution of NeuralGCM-0.7° closely matches the ERA5 distribution in the extratropics (Extended Data Fig. 4b ), although it underestimates extreme events in the tropics (Extended Data Fig. 4a ). It is noted that the current version of NeuralGCM directly predicts tendencies for an atmospheric column, and thus cannot distinguish between precipitation and evaporation.
Geostrophic wind balance
We examined the extent to which NeuralGCM, GraphCast and ECMWF-HRES capture the geostrophic wind balance, the near-equilibrium between the dominant forces that drive large-scale dynamics in the mid-latitudes 30 . A recent study 16 highlighted that Pangu misrepresents the vertical structure of the geostrophic and ageostrophic winds and noted a deterioration at longer lead times. Similarly, we observe that GraphCast shows an error that worsens with lead time. In contrast, NeuralGCM more accurately depicts the vertical structure of the geostrophic and ageostrophic winds, as well as their ratio, compared with GraphCast across various rollouts, when compared against ERA5 data (Extended Data Fig. 3 ). However, ECMWF-HRES still shows a slightly closer alignment to ERA5 data than NeuralGCM does. Within NeuralGCM, the representation of the geostrophic wind’s vertical structure only slightly degrades in the initial few days, showing no noticeable changes thereafter, particularly beyond day 5.
Generalizing to unseen data
Physically consistent weather models should still perform well for weather conditions for which they were not trained. We expect that NeuralGCM may generalize better than machine-learning-only atmospheric models, because NeuralGCM employs neural networks that act locally in space, on individual vertical columns of the atmosphere. To explore this hypothesis, we compare versions of NeuralCGM-0.7° and GraphCast trained to 2017 on 5 years of weather forecasts beyond the training period (2018–2022) in Supplementary Fig. 36 . Unlike GraphCast, NeuralGCM does not show a clear trend of increasing error when initialized further into the future from the training data. To extend this test beyond 5 years, we trained a NeuralGCM-2.8° model using only data before 2000, and tested its skill for over 21 unseen years (Supplementary Fig. 35 ).
Climate simulations
Although our deterministic NeuralGCM models are trained to predict weather up to 3 days ahead, they are generally capable of simulating the atmosphere far beyond medium-range weather timescales. For extended climate simulations, we prescribe historical sea surface temperature (SST) and sea-ice concentration. These simulations feature many emergent phenomena of the atmosphere on timescales from months to decades.
For climate simulations with NeuralGCM, we use 2.8° and 1.4° deterministic models, which are relatively inexpensive to train (Supplementary Information section G.7 ) and allow us to explore a larger parameter space to find stable models. Previous studies found that running extended simulations with hybrid models is challenging due to numerical instabilities and climate drift 21 . To quantify stability in our selected models, we run multiple initial conditions and report how many of them finish without instability.
Seasonal cycle and emergent phenomena
To assess the capability of NeuralGCM to simulate various aspects of the seasonal cycle, we run 2-year simulations with NeuralGCM-1.4°. for 37 different initial conditions spaced every 10 days for the year 2019. Out of these 37 initial conditions, 35 successfully complete the full 2 years without instability; for case studies of instability, see Supplementary Information section H.7 , and Supplementary Figs. 26 and 27 . We compare results from NeuralGCM-1.4° for 2020 with ERA5 data and with outputs from the X-SHiELD global cloud-resolving model, which is coupled to an ocean model nudged towards reanalysis 31 . This X-SHiELD run has been used as a target for training machine-learning climate models 24 . For comparison, we evaluate models after regridding predictions to 1.4° resolution. This comparison slightly favours NeuralGCM because NeuralGCM was tuned to match ERA5, but the discrepancy between ERA5 and the actual atmosphere is small relative to model error.
Figure 4a shows the temporal variation of the global mean temperature to 2020, as captured by 35 simulations from NeuralGCM, in comparison with the ERA5 reanalysis and standard climatology benchmarks. The seasonality and variability of the global mean temperature from NeuralGCM are quantitatively similar to those observed in ERA5. The ensemble-mean temperature RMSE for NeuralGCM stands at 0.16 K when benchmarked against ERA5, which is a significant improvement over the climatology’s RMSE of 0.45 K. We find that NeuralGCM accurately simulates the seasonal cycle, as evidenced by metrics such as the annual cycle of the global precipitable water (Supplementary Fig. 30a ) and global total kinetic energy (Supplementary Fig. 30b ). Furthermore, the model captures essential atmospheric dynamics, including the Hadley circulation and the zonal-mean zonal wind (Supplementary Fig. 28 ), as well as the spatial patterns of eddy kinetic energy in different seasons (Supplementary Fig. 31 ), and the distinctive seasonal behaviours of monsoon circulation (Supplementary Fig. 29 ; additional details are provided in Supplementary Information section I.1 ).
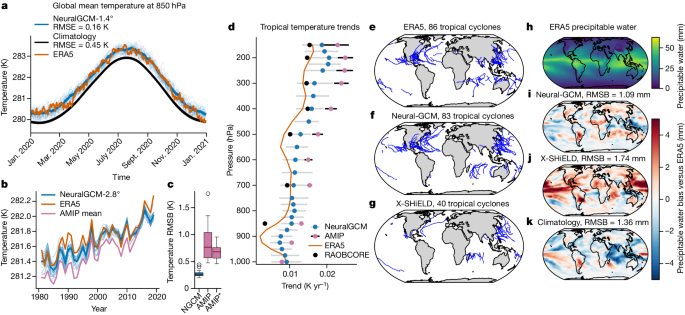
a , Global mean temperature for ERA5 14 (orange), 1990–2019 climatology (black) and NeuralGCM-1.4° (blue) for 2020 using 35 simulations initialized every 10 days during 2019 (thick line, ensemble mean; thin lines, different initial conditions). b , Yearly global mean temperature for ERA5 (orange), mean over 22 CMIP6 AMIP experiments 34 (violet; model details are in Supplementary Information section I.3 ) and NeuralGCM-2.8° for 22 AMIP-like simulations with prescribed SST initialized every 10 days during 1980 (thick line, ensemble mean; thin lines, different initial conditions). c , The RMSB of the 850-hPa temperature averaged between 1981 and 2014 for 22 NeuralGCM-2.8° AMIP runs (labelled NGCM), 22 CMIP6 AMIP experiments (labelled AMIP) and debiased 22 CMIP6 AMIP experiments (labelled AMIP*; bias was removed by removing the 850-hPa global temperature bias). In the box plots, the red line represents the median. The box delineates the first to third quartiles; the whiskers extend to 1.5 times the interquartile range (Q1 − 1.5IQR and Q3 + 1.5IQR), and outliers are shown as individual dots. d , Vertical profiles of tropical (20° S–20° N) temperature trends for 1981–2014. Orange, ERA5; black dots, Radiosonde Observation Correction using Reanalyses (RAOBCORE) 41 ; blue dots, mean trends for NeuralGCM; purple dots, mean trends from CMIP6 AMIP runs (grey and black whiskers, 25th and 75th percentiles for NeuralGCM and CMIP6 AMIP runs, respectively). e – g , Tropical cyclone tracks for ERA5 ( e ), NeuralGCM-1.4° ( f ) and X-SHiELD 31 ( g ). h – k , Mean precipitable water for ERA5 ( h ) and the precipitable water bias in NeuralGCM-1.4° ( i ), initialized 90 days before mid-January 2020 similarly to X-SHiELD, X-SHiELD ( j ) and climatology ( k ; averaged between 1990 and 2019). In d – i , quantities are calculated between mid-January 2020 and mid-January 2021 and all models were regridded to a 256 × 128 Gaussian grid before computation and tracking.
Next, we compare the annual biases of a single NeuralGCM realization with a single realization of X-SHiELD (the only one available), both initiated in mid-October 2019. We consider 19 January 2020 to 17 January 2021, the time frame for which X-SHiELD data are available. Global cloud-resolving models, such as X-SHiELD, are considered state of the art, especially for simulating the hydrological cycle, owing to their resolution being capable of resolving deep convection 32 . The annual bias in precipitable water for NeuralGCM (RMSE of 1.09 mm) is substantially smaller than the biases of both X-SHiELD (RMSE of 1.74 mm) and climatology (RMSE of 1.36 mm; Fig. 4i–k ). Moreover, NeuralGCM shows a lower temperature bias in the upper and lower troposphere than X-SHiELD (Extended Data Fig. 6 ). We also indirectly compare precipitation bias in X-SHiELD with precipitation-minus-evaporation bias in NeuralGCM-1.4°, which shows slightly larger bias and grid-scale artefacts for NeuralGCM (Extended Data Fig. 5 ).
Finally, to assess the capability of NeuralGCM to generate tropical cyclones in an annual model integration, we use the tropical cyclone tracker TempestExtremes 33 , as described in Supplementary Information section I.2 , Supplementary Fig. 34 and Supplementary Table 6 . Figure 4e–g shows that NeuralGCM, even at a coarse resolution of 1.4°, produces realistic trajectories and counts of tropical cyclone (83 versus 86 in ERA5 for the corresponding period), whereas X-SHiELD, when regridded to 1.4° resolution, substantially underestimates the tropical cyclone count (40). Additional statistical analyses of tropical cyclones can be found in Extended Data Figs. 7 and 8 .
Decadal simulations
To assess the capability of NeuralGCM to simulate historical temperature trends, we conduct AMIP-like simulations over a duration of 40 years with NeuralGCM-2.8°. Out of 37 different runs with initial conditions spaced every 10 days during the year 1980, 22 simulations were stable for the entire 40-year period, and our analysis focuses on these results. We compare with 22 simulations run with prescribed SST from the Coupled Model Intercomparison Project Phase 6 (CMIP6) 34 , listed in Supplementary Information section I.3 .
We find that all 40-year simulations of NeuralGCM, as well as the mean of the 22 AMIP runs, accurately capture the global warming trends observed in ERA5 data (Fig. 4b ). There is a strong correlation in the year-to-year temperature trends with ERA5 data, suggesting that NeuralGCM effectively captures the impact of SST forcing on climate. When comparing spatial biases averaged over 1981–2014, we find that all 22 NeuralGCM-2.8° runs have smaller bias than the CMIP6 AMIP runs, and this result remains even when removing the global temperature bias in CMIP6 AMIP runs (Fig. 4c and Supplementary Figs. 32 and 33 ).
Next, we investigated the vertical structure of tropical warming trends, which climate models tend to overestimate in the upper troposphere 35 . As shown in Fig. 4d , the trends, calculated by linear regression, of NeuralGCM are closer to ERA5 than those of AMIP runs. In particular, the bias in the upper troposphere is reduced. However, NeuralGCM does show a wider spread in its predictions than the AMIP runs, even at levels near the surface where temperatures are typically more constrained by prescribed SST.
Lastly, we evaluated NeuralGCM’s capability to generalize to unseen warmer climates by conducting AMIP simulations with increased SST (Supplementary Information section I.4.2 ). We find that NeuralGCM shows some of the robust features of climate warming response to modest SST increases (+1 K and +2 K); however, for more substantial SST increases (+4 K), NeuralGCM’s response diverges from expectations (Supplementary Fig. 37 ). In addition, AMIP simulations with increased SST show climate drift, underscoring NeuralGCM’s limitations in this context (Supplementary Fig. 38 ).
NeuralGCM is a differentiable hybrid atmospheric model that combines the strengths of traditional GCMs with machine learning for weather forecasting and climate simulation. To our knowledge, NeuralGCM is the first machine-learning-based model to make accurate ensemble weather forecasts, with better CRPS than state-of-the-art physics-based models. It is also, to our knowledge, the first hybrid model that achieves comparable spatial bias to global cloud-resolving models, can simulate realistic tropical cyclone tracks and can run AMIP-like simulations with realistic historical temperature trends. Overall, NeuralGCM demonstrates that incorporating machine learning is a viable alternative to building increasingly detailed physical models 32 for improving GCMs.
Compared with traditional GCMs with similar skill, NeuralGCM is computationally efficient and low complexity. NeuralGCM runs at 8- to 40-times-coarser horizontal resolution than ECMWF’s Integrated Forecasting System and global cloud-resolving models, which enables 3 to 5 orders of magnitude savings in computational resources. For example, NeuralGCM-1.4° simulates 70,000 simulation days in 24 hours using a single tensor-processing-unit versus 19 simulated days on 13,824 central-processing-unit cores with X-SHiELD (Extended Data Table 1 ). This can be leveraged for previously impractical tasks such as large ensemble forecasting. NeuralGCM’s dynamical core uses global spectral methods 36 , and learned physics is parameterized with fully connected neural networks acting on single vertical columns. Substantial headroom exists to pursue higher accuracy using advanced numerical methods and machine-learning architectures.
Our results provide strong evidence for the disputed hypothesis 37 , 38 , 39 that learning to predict short-term weather is an effective way to tune parameterizations for climate. NeuralGCM models trained on 72-hour forecasts are capable of realistic multi-year simulation. When provided with historical SSTs, they capture essential atmospheric dynamics such as seasonal circulation, monsoons and tropical cyclones. However, we will probably need alternative training strategies 38 , 39 to learn important processes for climate with subtle impacts on weather timescales, such as a cloud feedback.
The NeuralGCM approach is compatible with incorporating either more physics or more machine learning, as required for operational weather forecasts and climate simulations. For weather forecasting, we expect that end-to-end learning 40 with observational data will allow for better and more relevant predictions, including key variables such as precipitation. Such models could include neural networks acting as corrections to traditional data assimilation and model diagnostics. For climate projection, NeuralGCM will need to be reformulated to enable coupling with other Earth-system components (for example, ocean and land), and integrating data on the atmospheric chemical composition (for example, greenhouse gases and aerosols). There are also research challenges common to current machine-learning-based climate models 19 , including the capability to simulate unprecedented climates (that is, generalization), adhering to physical constraints, and resolving numerical instabilities and climate drift. NeuralGCM’s flexibility to incorporate physics-based models (for example, radiation) offers a promising avenue to address these challenges.
Models based on physical laws and empirical relationships are ubiquitous in science. We believe the differentiable hybrid modelling approach of NeuralGCM has the potential to transform simulation for a wide range of applications, such as materials discovery, protein folding and multiphysics engineering design.
Differentiable atmospheric model
NeuralGCM combines components of the numerical solver and flexible neural network parameterizations. Simulation in time is carried out in a coordinate system suitable for solving the dynamical equations of the atmosphere, describing large-scale fluid motion and thermodynamics under the influence of gravity and the Coriolis force.
Our differentiable dynamical core is implemented in JAX, a library for high-performance code in Python that supports automatic differentiation 42 . The dynamical core solves the hydrostatic primitive equations with moisture, using a horizontal pseudo-spectral discretization and vertical sigma coordinates 36 , 43 . We evolve seven prognostic variables: vorticity and divergence of horizontal wind, temperature, surface pressure, and three water species (specific humidity, and specific ice and liquid cloud water content).
Our learned physics module uses the single-column approach of GCMs 2 , whereby information from only a single atmospheric column is used to predict the impact of unresolved processes occurring within that column. These effects are predicted using a fully connected neural network with residual connections, with weights shared across all atmospheric columns (Supplementary Information section C.4 ).
The inputs to the neural network include the prognostic variables in the atmospheric column, total incident solar radiation, sea-ice concentration and SST (Supplementary Information section C.1 ). We also provide horizontal gradients of the prognostic variables, which we found improves performance 44 . All inputs are standardized to have zero mean and unit variance using statistics precomputed during model initialization. The outputs are the prognostic variable tendencies scaled by the fixed unconditional standard deviation of the target field (Supplementary Information section C.5 ).
To interface between ERA5 14 data stored in pressure coordinates and the sigma coordinate system of our dynamical core, we introduce encoder and decoder components (Supplementary Information section D ). These components perform linear interpolation between pressure levels and sigma coordinate levels. We additionally introduce learned corrections to both encoder and decoder steps (Supplementary Figs. 4–6 ), using the same column-based neural network architecture as the learned physics module. Importantly, the encoder enables us to eliminate the gravity waves from initialization shock 45 , which otherwise contaminate forecasts.
Figure 1a shows the sequence of steps that NeuralGCM takes to make a forecast. First, it encodes ERA5 data at t = t 0 on pressure levels to initial conditions on sigma coordinates. To perform a time step, the dynamical core and learned physics (Fig. 1b ) then compute tendencies, which are integrated in time using an implicit–explicit ordinary differential equation solver 46 (Supplementary Information section E and Supplementary Table 2 ). This is repeated to advance the model from t = t 0 to t = t final . Finally, the decoder converts predictions back to pressure levels.
The time-step size of the ODE solver (Supplementary Table 3 ) is limited by the Courant–Friedrichs–Lewy condition on dynamics, and can be small relative to the timescale of atmospheric change. Evaluating learned physics is approximately 1.5 times as expensive as a time step of the dynamical core. Accordingly, following the typical practice for GCMs, we hold learned physics tendencies constant for multiple ODE time steps to reduce computational expense, typically corresponding to 30 minutes of simulation time.
Deterministic and stochastic models
We train deterministic NeuralGCM models using a combination of three loss functions (Supplementary Information section G.4 ) to encourage accuracy and sharpness while penalizing bias. During the main training phase, all losses are defined in a spherical harmonics basis. We use a standard mean squared error loss for prompting accuracy, modified to progressively filter out contributions from higher total wavenumbers at longer lead times (Supplementary Fig. 8 ). This filtering approach tackles the ‘double penalty problem’ 47 as it prevents the model from being penalized for predicting high-wavenumber features in incorrect locations at later times, especially beyond the predictability horizon. A second loss term encourages the spectrum to match the training data using squared loss on the total wavenumber spectrum of prognostic variables. These first two losses are evaluated on both sigma and pressure levels. Finally, a third loss term discourages bias by adding mean squared error on the batch-averaged mean amplitude of each spherical harmonic coefficient. For analysis of the impact that various loss functions have, refer to Supplementary Information section H.6.1 , and Supplementary Figs. 23 and 24 . The combined action of the three training losses allow the resulting models trained on 3-day rollouts to remain stable during years-to-decades-long climate simulations. Before final evaluations, we perform additional fine-tuning of just the decoder component on short rollouts of 24 hours (Supplementary Information section G.5 ).
Stochastic NeuralGCM models incorporate inherent randomness in the form of additional random fields passed as inputs to neural network components. Our stochastic loss is based on the CRPS 28 , 48 , 49 . CRPS consists of mean absolute error that encourages accuracy, balanced by a similar term that encourages ensemble spread. For each variable we use a sum of CRPS in grid space and CRPS in the spherical harmonic basis below a maximum cut-off wavenumber (Supplementary Information section G.6 ). We compute CRPS on rollout lengths from 6 hours to 5 days. As illustrated in Fig. 1 , we inject noise to the learned encoder and the learned physics module by sampling from Gaussian random fields with learned spatial and temporal correlation (Supplementary Information section C.2 and Supplementary Fig. 2 ). For training, we generate two ensemble members per forecast, which suffices for an unbiased estimate of CRPS.
Data availability
For training and evaluating the NeuralGCM models, we used the publicly available ERA5 dataset 14 , originally downloaded from https://cds.climate.copernicus.eu/ and available via Google Cloud Storage in Zarr format at gs://gcp-public-data-arco-era5/ar/full_37-1h-0p25deg-chunk-1.zarr-v3. To compare NeuralGCM with operational and data-driven weather models, we used forecast datasets distributed as part of WeatherBench2 12 at https://weatherbench2.readthedocs.io/en/latest/data-guide.html , to which we have added NeuralGCM forecasts for 2020. To compare NeuralGCM with atmospheric models in climate settings, we used CMIP6 data available at https://catalog.pangeo.io/browse/master/climate/ , as well as X-SHiELD 24 outputs available on Google Cloud storage in a ‘requester pays’ bucket at gs://ai2cm-public-requester-pays/C3072-to-C384-res-diagnostics. The Radiosonde Observation Correction using Reanalyses (RAOBCORE) V1.9 that was used as reference tropical temperature trends was downloaded from https://webdata.wolke.img.univie.ac.at/haimberger/v1.9/ . Base maps use freely available data from https://www.naturalearthdata.com/downloads/ .
Code availability
The NeuralGCM code base is separated into two open source projects: Dinosaur and NeuralGCM, both publicly available on GitHub at https://github.com/google-research/dinosaur (ref. 50 ) and https://github.com/google-research/neuralgcm (ref. 51 ). The Dinosaur package implements a differentiable dynamical core used by NeuralGCM, whereas the NeuralGCM package provides machine-learning models and checkpoints of trained models. Evaluation code for NeuralGCM weather forecasts is included in WeatherBench2 12 , available at https://github.com/google-research/weatherbench2 (ref. 52 ).
Bauer, P., Thorpe, A. & Brunet, G. The quiet revolution of numerical weather prediction. Nature 525 , 47–55 (2015).
Article ADS CAS PubMed Google Scholar
Balaji, V. et al. Are general circulation models obsolete? Proc. Natl Acad. Sci. USA 119 , e2202075119 (2022).
Article CAS PubMed PubMed Central Google Scholar
Lam, R. et al. Learning skillful medium-range global weather forecasting. Science 382 , 1416–1421 (2023).
Article ADS MathSciNet CAS PubMed Google Scholar
Bi, K. et al. Accurate medium-range global weather forecasting with 3D neural networks. Nature 619 , 533–538 (2023).
Article ADS CAS PubMed PubMed Central Google Scholar
Hourdin, F. et al. The art and science of climate model tuning. Bull. Am. Meteorol. Soc. 98 , 589–602 (2017).
Article ADS Google Scholar
Bony, S. & Dufresne, J.-L. Marine boundary layer clouds at the heart of tropical cloud feedback uncertainties in climate models. Geophys. Res. Lett. 32 , L20806 (2005).
Webb, M. J., Lambert, F. H. & Gregory, J. M. Origins of differences in climate sensitivity, forcing and feedback in climate models. Clim. Dyn. 40 , 677–707 (2013).
Article Google Scholar
Sherwood, S. C., Bony, S. & Dufresne, J.-L. Spread in model climate sensitivity traced to atmospheric convective mixing. Nature 505 , 37–42 (2014).
Article ADS PubMed Google Scholar
Palmer, T. & Stevens, B. The scientific challenge of understanding and estimating climate change. Proc. Natl Acad. Sci. USA 116 , 24390–24395 (2019).
Fischer, E. M., Beyerle, U. & Knutti, R. Robust spatially aggregated projections of climate extremes. Nat. Clim. Change 3 , 1033–1038 (2013).
Field, C. B. Managing the Risks of Extreme Events and Disasters to Advance Climate Change Adaptation: Special Report of the Intergovernmental Panel on Climate Change (Cambridge Univ. Press, 2012).
Rasp, S. et al. WeatherBench 2: A benchmark for the next generation of data-driven global weather models. J. Adv. Model. Earth Syst. 16 , e2023MS004019 (2024).
Keisler, R. Forecasting global weather with graph neural networks. Preprint at https://arxiv.org/abs/2202.07575 (2022).
Hersbach, H. et al. The ERA5 global reanalysis. Q. J. R. Meteorol. Soc. 146 , 1999–2049 (2020).
Zhou, L. et al. Toward convective-scale prediction within the next generation global prediction system. Bull. Am. Meteorol. Soc. 100 , 1225–1243 (2019).
Bonavita, M. On some limitations of current machine learning weather prediction models. Geophys. Res. Lett. 51 , e2023GL107377 (2024).
Weyn, J. A., Durran, D. R. & Caruana, R. Improving data-driven global weather prediction using deep convolutional neural networks on a cubed sphere. J. Adv. Model. Earth Syst. 12 , e2020MS002109 (2020).
Watt-Meyer, O. et al. ACE: a fast, skillful learned global atmospheric model for climate prediction. Preprint at https://arxiv.org/abs/2310.02074 (2023).
Bretherton, C. S. Old dog, new trick: reservoir computing advances machine learning for climate modeling. Geophys. Res. Lett. 50 , e2023GL104174 (2023).
Reichstein, M. et al. Deep learning and process understanding for data-driven Earth system science. Nature 566 , 195–204 (2019).
Brenowitz, N. D. & Bretherton, C. S. Spatially extended tests of a neural network parametrization trained by coarse-graining. J. Adv. Model. Earth Syst. 11 , 2728–2744 (2019).
Rasp, S., Pritchard, M. S. & Gentine, P. Deep learning to represent subgrid processes in climate models. Proc. Natl Acad. Sci. USA 115 , 9684–9689 (2018).
Yuval, J. & O’Gorman, P. A. Stable machine-learning parameterization of subgrid processes for climate modeling at a range of resolutions. Nat. Commun. 11 , 3295 (2020).
Kwa, A. et al. Machine-learned climate model corrections from a global storm-resolving model: performance across the annual cycle. J. Adv. Model. Earth Syst. 15 , e2022MS003400 (2023).
Arcomano, T., Szunyogh, I., Wikner, A., Hunt, B. R. & Ott, E. A hybrid atmospheric model incorporating machine learning can capture dynamical processes not captured by its physics-based component. Geophys. Res. Lett. 50 , e2022GL102649 (2023).
Han, Y., Zhang, G. J. & Wang, Y. An ensemble of neural networks for moist physics processes, its generalizability and stable integration. J. Adv. Model. Earth Syst. 15 , e2022MS003508 (2023).
Gelbrecht, M., White, A., Bathiany, S. & Boers, N. Differentiable programming for Earth system modeling. Geosci. Model Dev. 16 , 3123–3135 (2023).
Gneiting, T. & Raftery, A. E. Strictly proper scoring rules, prediction, and estimation. J. Am. Stat. Assoc. 102 , 359–378 (2007).
Article MathSciNet CAS Google Scholar
Fortin, V., Abaza, M., Anctil, F. & Turcotte, R. Why should ensemble spread match the RMSE of the ensemble mean? J. Hydrometeorol. 15 , 1708–1713 (2014).
Holton, J. R. An introduction to Dynamic Meteorology 5th edn (Elsevier, 2004).
Cheng, K.-Y. et al. Impact of warmer sea surface temperature on the global pattern of intense convection: insights from a global storm resolving model. Geophys. Res. Lett. 49 , e2022GL099796 (2022).
Stevens, B. et al. DYAMOND: the dynamics of the atmospheric general circulation modeled on non-hydrostatic domains. Prog. Earth Planet. Sci. 6 , 61 (2019).
Ullrich, P. A. et al. TempestExtremes v2.1: a community framework for feature detection, tracking, and analysis in large datasets. Geosc. Model Dev. 14 , 5023–5048 (2021).
Eyring, V. et al. Overview of the Coupled Model Intercomparison Project Phase 6 (CMIP6) experimental design and organization. Geosci. Model Dev. 9 , 1937–1958 (2016).
Mitchell, D. M., Lo, Y. E., Seviour, W. J., Haimberger, L. & Polvani, L. M. The vertical profile of recent tropical temperature trends: persistent model biases in the context of internal variability. Environ. Res. Lett. 15 , 1040b4 (2020).
Bourke, W. A multi-level spectral model. I. Formulation and hemispheric integrations. Mon. Weather Rev. 102 , 687–701 (1974).
Ruiz, J. J., Pulido, M. & Miyoshi, T. Estimating model parameters with ensemble-based data assimilation: a review. J. Meteorol. Soc. Jpn Ser. II 91 , 79–99 (2013).
Schneider, T., Lan, S., Stuart, A. & Teixeira, J. Earth system modeling 2.0: a blueprint for models that learn from observations and targeted high-resolution simulations. Geophys. Res. Lett. 44 , 12–396 (2017).
Schneider, T., Leung, L. R. & Wills, R. C. J. Opinion: Optimizing climate models with process knowledge, resolution, and artificial intelligence. Atmos. Chem. Phys. 24 , 7041–7062 (2024).
Sutskever, I., Vinyals, O. & Le, Q. V. Sequence to sequence learning with neural networks. Adv. Neural Inf. Process. Syst. 27 , 3104–3112 (2014).
Haimberger, L., Tavolato, C. & Sperka, S. Toward elimination of the warm bias in historic radiosonde temperature records—some new results from a comprehensive intercomparison of upper-air data. J. Clim. 21 , 4587–4606 (2008).
Bradbury, J. et al. JAX: composable transformations of Python+NumPy programs. GitHub http://github.com/google/jax (2018).
Durran, D. R. Numerical Methods for Fluid Dynamics: With Applications to Geophysics Vol. 32, 2nd edn (Springer, 2010).
Wang, P., Yuval, J. & O’Gorman, P. A. Non-local parameterization of atmospheric subgrid processes with neural networks. J. Adv. Model. Earth Syst. 14 , e2022MS002984 (2022).
Daley, R. Normal mode initialization. Rev. Geophys. 19 , 450–468 (1981).
Whitaker, J. S. & Kar, S. K. Implicit–explicit Runge–Kutta methods for fast–slow wave problems. Mon. Weather Rev. 141 , 3426–3434 (2013).
Gilleland, E., Ahijevych, D., Brown, B. G., Casati, B. & Ebert, E. E. Intercomparison of spatial forecast verification methods. Weather Forecast. 24 , 1416–1430 (2009).
Rasp, S. & Lerch, S. Neural networks for postprocessing ensemble weather forecasts. Month. Weather Rev. 146 , 3885–3900 (2018).
Pacchiardi, L., Adewoyin, R., Dueben, P. & Dutta, R. Probabilistic forecasting with generative networks via scoring rule minimization. J. Mach. Learn. Res. 25 , 1–64 (2024).
Smith, J. A., Kochkov, D., Norgaard, P., Yuval, J. & Hoyer, S. google-research/dinosaur: 1.0.0. Zenodo https://doi.org/10.5281/zenodo.11376145 (2024).
Kochkov, D. et al. google-research/neuralgcm: 1.0.0. Zenodo https://doi.org/10.5281/zenodo.11376143 (2024).
Rasp, S. et al. google-research/weatherbench2: v0.2.0. Zenodo https://doi.org/10.5281/zenodo.11376271 (2023).
Download references
Acknowledgements
We thank A. Kwa, A. Merose and K. Shah for assistance with data acquisition and handling; L. Zepeda-Núñez for feedback on the paper; and J. Anderson, C. Van Arsdale, R. Chemke, G. Dresdner, J. Gilmer, J. Hickey, N. Lutsko, G. Nearing, A. Paszke, J. Platt, S. Ponda, M. Pritchard, D. Rothenberg, F. Sha, T. Schneider and O. Voicu for discussions.
Author information
These authors contributed equally: Dmitrii Kochkov, Janni Yuval, Ian Langmore, Peter Norgaard, Jamie Smith, Stephan Hoyer
Authors and Affiliations
Google Research, Mountain View, CA, USA
Dmitrii Kochkov, Janni Yuval, Ian Langmore, Peter Norgaard, Jamie Smith, Griffin Mooers, James Lottes, Stephan Rasp, Michael P. Brenner & Stephan Hoyer
Earth, Atmospheric and Planetary Sciences, Massachusetts Institute of Technology, Cambridge, MA, USA
Milan Klöwer
European Centre for Medium-Range Weather Forecasts, Reading, UK
Peter Düben & Sam Hatfield
Google DeepMind, London, UK
Peter Battaglia, Alvaro Sanchez-Gonzalez & Matthew Willson
School of Engineering and Applied Sciences, Harvard University, Cambridge, MA, USA
Michael P. Brenner
You can also search for this author in PubMed Google Scholar
Contributions
D.K., J.Y., I.L., P.N., J.S. and S. Hoyer contributed equally to this work. D.K., J.Y., I.L., P.N., J.S., G.M., J.L. and S. Hoyer wrote the code. D.K., J.Y., I.L., P.N., G.M. and S. Hoyer trained models and analysed the data. M.P.B. and S. Hoyer managed and oversaw the research project. M.K., S.R., P.D., S. Hatfield, P.B. and M.P.B. contributed technical advice and ideas. M.W. ran experiments with GraphCast for comparison with NeuralGCM. A.S.-G. assisted with data preparation. D.K., J.Y., I.L., P.N. and S. Hoyer wrote the paper. All authors gave feedback and contributed to editing the paper.
Corresponding authors
Correspondence to Dmitrii Kochkov , Janni Yuval or Stephan Hoyer .
Ethics declarations
Competing interests.
D.K., J.Y., I.L., P.N., J.S., J.L., S.R., P.B., A.S.-G., M.W., M.P.B. and S. Hoyer are employees of Google. S. Hoyer, D.K., I.L., J.Y., G.M., P.N., J.S. and M.B. have filed international patent application PCT/US2023/035420 in the name of Google LLC, currently pending, relating to neural general circulation models.
Peer review
Peer review information.
Nature thanks Karthik Kashinath and the other, anonymous, reviewer(s) for their contribution to the peer review of this work. Peer reviewer reports are available.
Additional information
Publisher’s note Springer Nature remains neutral with regard to jurisdictional claims in published maps and institutional affiliations.
Extended data figures and tables
Extended data fig. 1 maps of bias for neuralgcm-ens and ecmwf-ens forecasts..
Bias is averaged over all forecasts initialized in 2020.
Extended Data Fig. 2 Maps of spread-skill ratio for NeuralGCM-ENS and ECMWF-ENS forecasts.
Spread-skill ratio is averaged over all forecasts initialized in 2020.
Extended Data Fig. 3 Geostrophic balance in NeuralGCM, GraphCast 3 and ECMWF-HRES.
Vertical profiles of the extratropical intensity (averaged between latitude 30°–70° in both hemispheres) and over all forecasts initialized in 2020 of (a,d,g) geostrophic wind, (b,e,h) ageostrophic wind and (c,f,i) the ratio of the intensity of ageostrophic wind over geostrophic wind for ERA5 (black continuous line in all panels), (a,b,c) NeuralGCM-0.7°, (d,e,f) GraphCast and (g,h,i) ECMWF-HRES at lead times of 1 day, 5 days and 10 days.
Extended Data Fig. 4 Precipitation minus evaporation calculated from the third day of weather forecasts.
(a) Tropical (latitudes −20° to 20°) precipitation minus evaporation (P minus E) rate distribution, (b) Extratropical (latitudes 30° to 70° in both hemispheres) P minus E, (c) mean P minus E for 2020 ERA5 14 and (d) NeuralGCM-0.7° (calculated from the third day of forecasts and averaged over all forecasts initialized in 2020), (e) the bias between NeuralGCM-0.7° and ERA5, (f-g) Snapshot of daily precipitation minus evaporation for 2020-01-04 for (f) NeuralGCM-0.7° (forecast initialized on 2020-01-02) and (g) ERA5.
Extended Data Fig. 5 Indirect comparison between precipitation bias in X-SHiELD and precipitation minus evaporation bias in NeuralGCM-1.4°.
Mean precipitation calculated between 2020-01-19 and 2021-01-17 for (a) ERA5 14 (c) X-SHiELD 31 and the biases in (e) X-SHiELD and (g) climatology (ERA5 data averaged over 1990-2019). Mean precipitation minus evaporation calculated between 2020-01-19 and 2021-01-17 for (b) ERA5 (d) NeuralGCM-1.4° (initialized in October 18th 2019) and the biases in (f) NeuralGCM-1.4° and (h) climatology (data averaged over 1990–2019).
Extended Data Fig. 6 Yearly temperature bias for NeuralGCM and X-SHiELD 31 .
Mean temperature between 2020-01-19 to 2020-01-17 for (a) ERA5 at 200hPa and (b) 850hPa. (c,d) the bias in the temperature for NeuralGCM-1.4°, (e,f) the bias in X-SHiELD and (g,h) the bias in climatology (calculated from 1990–2019). NeuralGCM-1.4° was initialized in 18th of October (similar to X-SHiELD).
Extended Data Fig. 7 Tropical Cyclone densities and annual regional counts.
(a) Tropical Cyclone (TC) density from ERA5 14 data spanning 1987–2020. (b) TC density from NeuralGCM-1.4° for 2020, generated using 34 different initial conditions all initialized in 2019. (c) Box plot depicting the annual number of TCs across different regions, based on ERA5 data (1987–2020), NeuralGCM-1.4° for 2020 (34 initial conditions), and orange markers show ERA5 for 2020. In the box plots, the red line represents the median; the box delineates the first to third quartiles; the whiskers extend to 1.5 times the interquartile range (Q1 − 1.5IQR and Q3 + 1.5IQR), and outliers are shown as individual dots. Each year is defined from January 19th to January 17th of the following year, aligning with data availability from X-SHiELD. For NeuralGCM simulations, the 3 initial conditions starting in January 2019 exclude data for January 17th, 2021, as these runs spanned only two years.
Extended Data Fig. 8 Tropical Cyclone maximum wind distribution in NeuralGCM vs. ERA5 14 .
Number of Tropical Cyclones (TCs) as a function of maximum wind speed at 850hPa across different regions, based on ERA5 data (1987–2020; in orange), and NeuralGCM-1.4° for 2020 (34 initial conditions; in blue). Each year is defined from January 19th to January 17th of the following year, aligning with data availability from X-SHiELD. For NeuralGCM simulations, the 3 initial conditions starting in January 2019 exclude data for January 17th, 2021, as these runs spanned only two years.
Supplementary information
Supplementary information.
Supplementary Information (38 figures, 6 tables): (A) Lines of code in atmospheric models; (B) Dynamical core of NeuralGCM; (C) Learned physics of NeuralGCM; (D) Encoder and decoder of NeuralGCM; (E) Time integration; (F) Evaluation metrics; (G) Training; (H) Additional weather evaluations; (I) Additional climate evaluations.
Peer Review File
Rights and permissions.
Open Access This article is licensed under a Creative Commons Attribution 4.0 International License, which permits use, sharing, adaptation, distribution and reproduction in any medium or format, as long as you give appropriate credit to the original author(s) and the source, provide a link to the Creative Commons licence, and indicate if changes were made. The images or other third party material in this article are included in the article’s Creative Commons licence, unless indicated otherwise in a credit line to the material. If material is not included in the article’s Creative Commons licence and your intended use is not permitted by statutory regulation or exceeds the permitted use, you will need to obtain permission directly from the copyright holder. To view a copy of this licence, visit http://creativecommons.org/licenses/by/4.0/ .
Reprints and permissions
About this article
Cite this article.
Kochkov, D., Yuval, J., Langmore, I. et al. Neural general circulation models for weather and climate. Nature 632 , 1060–1066 (2024). https://doi.org/10.1038/s41586-024-07744-y
Download citation
Received : 13 November 2023
Accepted : 15 June 2024
Published : 22 July 2024
Issue Date : 29 August 2024
DOI : https://doi.org/10.1038/s41586-024-07744-y
Share this article
Anyone you share the following link with will be able to read this content:
Sorry, a shareable link is not currently available for this article.
Provided by the Springer Nature SharedIt content-sharing initiative
This article is cited by
Google ai predicts long-term climate trends and weather — in minutes.
- Helena Kudiabor
Nature (2024)
Weather and climate predicted accurately — without using a supercomputer
- Oliver Watt-Meyer
By submitting a comment you agree to abide by our Terms and Community Guidelines . If you find something abusive or that does not comply with our terms or guidelines please flag it as inappropriate.
Quick links
- Explore articles by subject
- Guide to authors
- Editorial policies
Sign up for the Nature Briefing: AI and Robotics newsletter — what matters in AI and robotics research, free to your inbox weekly.

Home — Essay Samples — Education — Studying Process — Physical Education

Essays on Physical Education
Prompt examples for physical education essays, the importance of physical education in schools.
Discuss the significance of including physical education as a part of the school curriculum. How does physical education benefit students' overall development?
Physical Education and Health
Explore the relationship between physical education and students' health. How does regular physical activity in schools contribute to the well-being of students?
The Role of Physical Education Teachers
Examine the responsibilities and impact of physical education teachers. How do they help students develop physical skills and a lifelong love for fitness?
Innovations in Physical Education
Discuss innovative approaches and technologies used in modern physical education programs. How have these innovations improved the learning experience?
Physical Education and Academic Performance
Explore the potential links between physical education and academic success. How does regular physical activity impact students' cognitive abilities and classroom performance?
The Role of Team Sports in Physical Education
Analyze the benefits of incorporating team sports into physical education programs. How do team sports promote cooperation, leadership, and physical fitness?
Physical Education for Special Populations
Discuss the importance of adapting physical education programs for students with special needs. How can inclusive physical education benefit all students?
Challenges and Solutions in Physical Education
Examine the challenges faced by physical education programs, such as limited resources or lack of facilities. What solutions can be implemented to overcome these challenges?
Physical Education and Lifelong Fitness
Explore the role of physical education in promoting lifelong fitness habits. How can physical education programs instill a love for physical activity beyond the school years?
Evaluating the Effectiveness of Physical Education
Discuss methods for assessing the effectiveness of physical education programs. How can schools measure the impact of these programs on students' health and well-being?
The Importance of Being Physically Active
The benefits of physical education: develop skills & confidence, made-to-order essay as fast as you need it.
Each essay is customized to cater to your unique preferences
+ experts online
The Importance of Physical Education to Maintain a Healthy and Happy Life
Physical education should always be included in the school curriculum, the benefits of taking a physical education course during school, the importance of compulsory physical education classes, let us write you an essay from scratch.
- 450+ experts on 30 subjects ready to help
- Custom essay delivered in as few as 3 hours
Gender Issues in Physical Education
Different types of play as an important part of young children’s development, game play participation of amotivated students during sport education, using videos in teaching physical education, get a personalized essay in under 3 hours.
Expert-written essays crafted with your exact needs in mind
The Importance of Sports for Students
Myers v. peel county board of education, the wingate test: peak power output and anaerobic systems in the college-aged population, social, mental, and physical benefits of sports for young adolescents, physical education in school as a tool for motor development, benefits of physical education in school for body development, the importance of physical education: a comprehensive analysis, the impact of physical education on student grades, importance of physical education in schools, ethics, education, and responsible practices in running, running as a conduit for physical and mental well-being, the sequence of cephalocaudal development, importance of physical education, physical education in schools.
Physical Education is an education which brings improvement in human performance with the help of physical activities.
Physical activities range from simple walking to jogging, running, sprinting, hopping, jumping, climbing, throwing, pushing, pulling, kicking, etc.
Physical education helps students develop physical skills and confidence. It develops fitness and fosters the desire for lifelong participation in physical activity. School curriculum prepares students to become highly proficient in one or more sport and/or fitness activity of their choice.
Relevant topics
- Critical Thinking
- Studying Abroad
- Academic Challenges
- Academic Interests
- High School
- Middle School
- School Uniform
- Academic Achievements
- Stem Education
By clicking “Check Writers’ Offers”, you agree to our terms of service and privacy policy . We’ll occasionally send you promo and account related email
No need to pay just yet!
We use cookies to personalyze your web-site experience. By continuing we’ll assume you board with our cookie policy .
- Instructions Followed To The Letter
- Deadlines Met At Every Stage
- Unique And Plagiarism Free
Blog The Education Hub
https://educationhub.blog.gov.uk/2024/08/20/gcse-results-day-2024-number-grading-system/
GCSE results day 2024: Everything you need to know including the number grading system
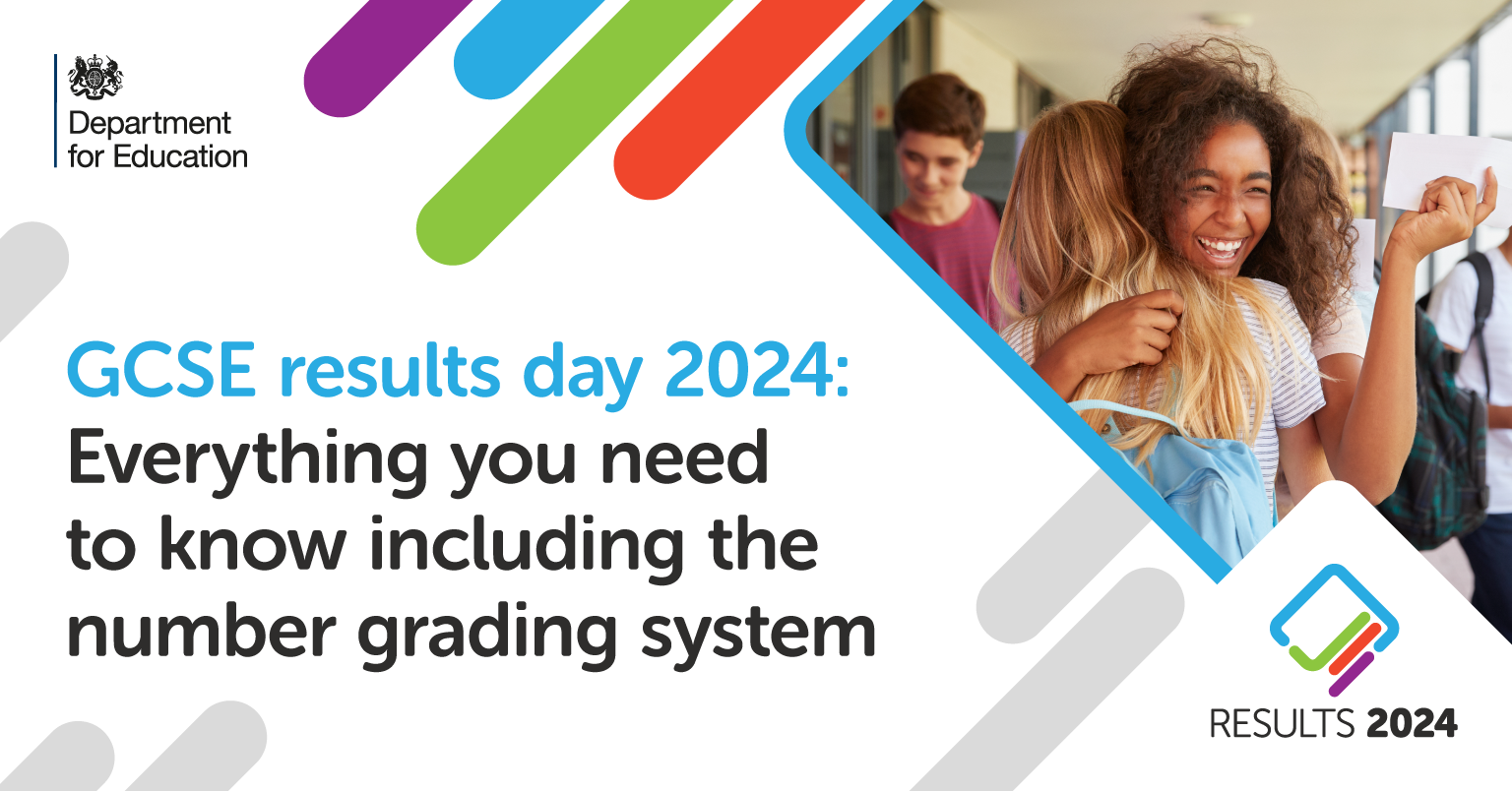
Thousands of students across the country will soon be finding out their GCSE results and thinking about the next steps in their education.
Here we explain everything you need to know about the big day, from when results day is, to the current 9-1 grading scale, to what your options are if your results aren’t what you’re expecting.
When is GCSE results day 2024?
GCSE results day will be taking place on Thursday the 22 August.
The results will be made available to schools on Wednesday and available to pick up from your school by 8am on Thursday morning.
Schools will issue their own instructions on how and when to collect your results.
When did we change to a number grading scale?
The shift to the numerical grading system was introduced in England in 2017 firstly in English language, English literature, and maths.
By 2020 all subjects were shifted to number grades. This means anyone with GCSE results from 2017-2020 will have a combination of both letters and numbers.
The numerical grading system was to signal more challenging GCSEs and to better differentiate between students’ abilities - particularly at higher grades between the A *-C grades. There only used to be 4 grades between A* and C, now with the numerical grading scale there are 6.
What do the number grades mean?
The grades are ranked from 1, the lowest, to 9, the highest.
The grades don’t exactly translate, but the two grading scales meet at three points as illustrated below.
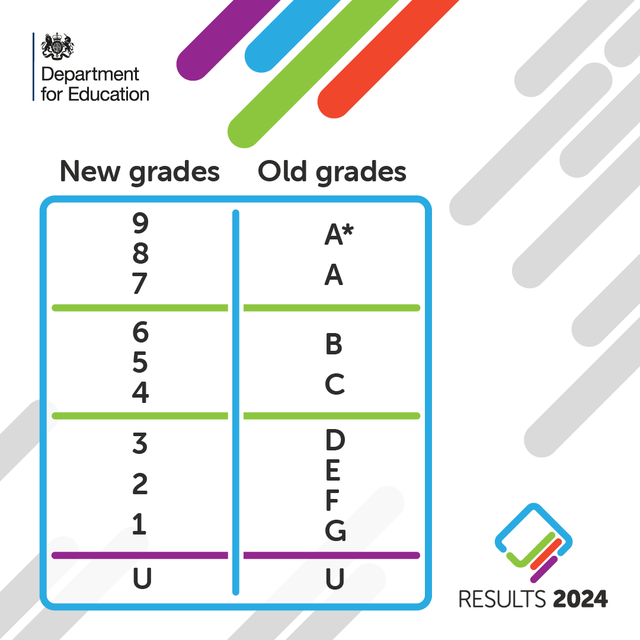
The bottom of grade 7 is aligned with the bottom of grade A, while the bottom of grade 4 is aligned to the bottom of grade C.
Meanwhile, the bottom of grade 1 is aligned to the bottom of grade G.
What to do if your results weren’t what you were expecting?
If your results weren’t what you were expecting, firstly don’t panic. You have options.
First things first, speak to your school or college – they could be flexible on entry requirements if you’ve just missed your grades.
They’ll also be able to give you the best tailored advice on whether re-sitting while studying for your next qualifications is a possibility.
If you’re really unhappy with your results you can enter to resit all GCSE subjects in summer 2025. You can also take autumn exams in GCSE English language and maths.
Speak to your sixth form or college to decide when it’s the best time for you to resit a GCSE exam.
Look for other courses with different grade requirements
Entry requirements vary depending on the college and course. Ask your school for advice, and call your college or another one in your area to see if there’s a space on a course you’re interested in.
Consider an apprenticeship
Apprenticeships combine a practical training job with study too. They’re open to you if you’re 16 or over, living in England, and not in full time education.
As an apprentice you’ll be a paid employee, have the opportunity to work alongside experienced staff, gain job-specific skills, and get time set aside for training and study related to your role.
You can find out more about how to apply here .
Talk to a National Careers Service (NCS) adviser
The National Career Service is a free resource that can help you with your career planning. Give them a call to discuss potential routes into higher education, further education, or the workplace.
Whatever your results, if you want to find out more about all your education and training options, as well as get practical advice about your exam results, visit the National Careers Service page and Skills for Careers to explore your study and work choices.
You may also be interested in:
- Results day 2024: What's next after picking up your A level, T level and VTQ results?
- When is results day 2024? GCSEs, A levels, T Levels and VTQs
Tags: GCSE grade equivalent , gcse number grades , GCSE results , gcse results day 2024 , gsce grades old and new , new gcse grades
Sharing and comments
Share this page, related content and links, about the education hub.
The Education Hub is a site for parents, pupils, education professionals and the media that captures all you need to know about the education system. You’ll find accessible, straightforward information on popular topics, Q&As, interviews, case studies, and more.
Please note that for media enquiries, journalists should call our central Newsdesk on 020 7783 8300. This media-only line operates from Monday to Friday, 8am to 7pm. Outside of these hours the number will divert to the duty media officer.
Members of the public should call our general enquiries line on 0370 000 2288.
Sign up and manage updates
Follow us on social media, search by date.
M | T | W | T | F | S | S |
---|---|---|---|---|---|---|
1 | 2 | 3 | 4 | |||
5 | 7 | 8 | 9 | 10 | 11 | |
13 | 14 | 15 | 16 | 17 | 18 | |
21 | 22 | 23 | 24 | 25 | ||
26 | 27 | 29 | 31 |
Comments and moderation policy

IMAGES
VIDEO
COMMENTS
The Nature and Values of Physical Education Essay. Physical educators have been recognized as contributors to school curriculum after a long and hard fight. Physical education proponents have claimed alliances with psychology, morality, science and medicine; these are the things that have validated physical education in the educational milieu ...
In conclusion, physical education is of utmost importance in schools. It not only promotes physical fitness but also contributes to mental well-being and academic performance. Physical education teaches valuable life skills and promotes social interaction and inclusivity. By incorporating physical education into the curriculum, schools can help ...
Introduction Physical education is an integral component of a well-rounded education, and its importance cannot be overstated. In this essay, we will explore the myriad benefits of physical education for students, focusing on its role in improving physical fitness, developing motor skills, and promoting regular physical activity.
To define physical education, we need to say that it is an educational process that aims to improve human development and performance through physical activity. It tends to take place at school through formal lessons, but it also includes informal activity such as play. Physical education is a process of learning, the context being mainly physical.
Physical education in schools has long been a topic of debate, with some arguing that it is a vital part of a well-rounded education, while others claim that it is unnecessary and takes away from valuable instructional time. However, the evidence overwhelmingly supports the idea that physical education is a crucial component of a student's education, offering a wide range of benefits that ...
Read chapter 5 Approaches to Physical Education in Schools: Physical inactivity is a key determinant of health across the lifespan. A lack of activity inc...
nature and values of physical education. On the one hand, physical education teachers are. apt to claim that theirs is essentially a practical vocation; a calling to the teaching of physical ...
High-quality essay on the topic of "Physical Education" for students in schools and colleges.
High-quality essay on the topic of "Why Physical Education Is Important" for students in schools and colleges.
Get a custom essay on Physical Education and Its Benefits. Physical exercise promotes blood circulation in the body especially in the brain and such activity works to reduce stress and improve on mood and attitude of the student as work without play makes Jack a dull boy. Moreover studies have proved that physically active children achieve more ...
What is the impact of physical education on students' well-being and academic success? Decreasing time for quality phys-ed to allow more instructional time for core curricular subjects - including math, science, social studies and English - is counterproductive, given its positive benefits on health outcomes and school achievement.
Looking for a good essay, research or speech topic on Physical Education? Check our list of 70 interesting Physical Education title ideas to write about!
The Philosophy of Physical Education: A New Perspective is a new member of the Routledge Studies in Physical Education and Youth Sport series. This series, which examines current social issues, pedagogical models, and in some cases research methods, has as its principal goal, 'to inform academic debate, and to have a high impact on both policy and practice.' To that extent, Steven A. Stolz ...
Key Messages Evidence suggests that increasing physical activity and physical fitness may improve academic performance and that time in the school day dedicated to recess, physical education class, and physical activity in the classroom may also facilitate academic performance.
Physical education contributes not only to physical health but also to mental well-being. Achieving success in sports and physical activities fosters a sense of accomplishment and positively impacts a student's confidence and overall well-being. The enjoyment derived from such achievements not only enhances the primary curriculum experience but ...
Physical education has been an integral part of school curriculum for centuries, with a rich history and evolving significance in promoting overall well-being. In this essay, the importance of physical education in schools will be explored, including its history, benefits, role in promoting social skills, as well as the challenges and controversies it faces.
Introduction Physical education (PE) is a vital component of a comprehensive educational curriculum that promotes the physical, mental, and social development of students. This essay explores the significance of physical education in schools, discussing its benefits, challenges, and the role it plays in fostering lifelong healthy habits.
Five themes were identified as central influences to young people's meaningful experiences in physical education and sport: social interaction, fun, challenge, motor competence, and personally relevant learning. These themes provide future direction for the design and implementation of meaningful physical education and youth sport experiences.
Physical education is a socially constructed activity that forms one component of a wider physical culture that includes sport and health/physical activity . The terms sport and physical education are often used interchangeably in school contexts, where sport and health continue to shape what is understood by the term physical education.
Conceptual physical education (CPE) courses (classes), based on physical education standards (physical literacy) and fitness-education benchmarks, use text materials and classroom sessions to teach kinesiology concepts, principles, and self-management skills. CPE is a mature innovation at the college level and is now widely used in secondary ...
Part III: Physical Activity During School Hours; Promotion of Physical Activity and Health in the School Setting makes an important contribution to the youth physical activity field for scholars and practitioners. The book is essential reading for advanced students, researchers, practitioners, and policy-makers with an interest in physical ...
Why is physical education important? This essay discusses the benefits of physical education and its importance for children in schools.
Nature provides a place and an opportunity for play and physical activity. Play is critical for children, as it aids in the development of cognitive thinking and reasoning skills. Nature encourages exploration and building, which improves problem solving, decision making, and the ability to respond to changing contexts.
The growing interest in sustainable urban development is driven by challenges posed by urbanization, socioeconomic activities and environmental issues 1.Urban areas contribute 80% of the world's ...
A hybrid model that combines a differentiable solver for atmospheric dynamics with machine-learning components is capable of weather forecasts and climate simulations on par with the best ...
Read the best free essays on Physical Education obstacles and get inspiration for your essays. Thanks to our essays you can expand your knowledge on this topic.
Thousands of students across the country will soon be finding out their GCSE results and thinking about the next steps in their education.. Here we explain everything you need to know about the big day, from when results day is, to the current 9-1 grading scale, to what your options are if your results aren't what you're expecting.