
An official website of the United States government
The .gov means it’s official. Federal government websites often end in .gov or .mil. Before sharing sensitive information, make sure you’re on a federal government site.
The site is secure. The https:// ensures that you are connecting to the official website and that any information you provide is encrypted and transmitted securely.
- Publications
- Account settings
Preview improvements coming to the PMC website in October 2024. Learn More or Try it out now .
- Advanced Search
- Journal List
- Ind Psychiatry J
- v.28(1); Jan-Jun 2019

Is obesity a risk to depression? A cross-sectional study
Department of Psychiatrist, Army College of Medical Sciences, New Delhi, India
Sachin Kumar Saxena
1 Department of Psychiatrist, Base Hospital Delhi Cantt, New Delhi, India
Sabreen Bashir
2 Department of Community Medicine, Army College of Medical Sciences, New Delhi, India
Background:
Depression and obesity are disorders of stress with a dose dependent relationship between the both. The adverse health and social consequences are significant, when depression and obesity co-exist. This study aimed to examine the prevalence of depression among overweight and obese patients in a large station of Armed Forces and associate other risk factors of depression.
This cross sectional descriptive study was conducted in the general OPD of large Station medicare centre (SMC) on overweight and obese personnel. Data was collected by self-administered Patient Health Questionnaire (PHQ-9) to assess the risk for depression over a period of one month.
In this study, out of 106 individuals, 71 (67%) were overweight and 35 (33%) were obese, as per WHO criteria. Of the individuals assessed, 13 (12%) individuals were found to have risk of moderate depression, 58 (54%) for mild depression and 35 (33%) individuals had no risk for clinically significant depression. The likelihood of depression was most strongly associated with BMI followed by age, status of living with family and habit of drinking alcohol.
Conclusion:
Obesity and depressive disorders are common comorbidities with overlapping pathophysiology whose co-existence leads to exponential adverse health outcomes. The outcome of depression and obesity is to be managed comprehensively by psychological counseling and life style modification.
Globally, the prevalence of depression and obesity are important public health concerns, with an estimated 350 and 500 million people suffering, respectively.[ 1 , 2 ] A recent meta-analysis of 17 community-based cross-sectional studies among adults showed a positive overall association between depression and obesity.[ 3 ] Depression and obesity are disorders of stress with dysregulation of the stress management system [ Figure 1 ].[ 4 ] Obesity is a systemic disorder, leading to increase in cortisol, leptin, insulin levels resulting in hypothalamic pituitary adrenal axis dysregulation, and insulin resistance, which can further induce inflammation and worsen depression.[ 5 ] There is a dose-dependent relationship between the both, that is, with higher body mass index (BMI) the risk of clinical depression increases.[ 3 ]

Pathoetiological connection between obesity and depression. ACTH – Adrenocorticotrophic hormone; CRH – Corticotropin-releasing hormone; CRP – C-reactive protein; HPA – Hypothalamic—pituitary—adrenal; IL – Interleukin; TNF – Tumour necrosis factor
Obesity and depression run in the vicious cycle [ Figure 2 ]. When an individual is in a depressed mood state, it is also associated with anhedonia and sedentary lifestyle along with dietary changes. On the other hand, obese patients have higher levels of leptin hormone, which is known to cause clinical depression. Depression and obesity are both associated with social stigma and low self-esteem.[ 6 ] The adverse health and social consequences are significant when depression and obesity cooccur. Symptoms of depression in obese patients are strongly associated with poor quality of life, particularly social functioning and mental health.[ 7 ] Further, obesity coupled with depression has significant economic implications, owing to reduced participation in the labor force and absenteeism.[ 8 ]

Vicious cycle of obesity and depression
There are limited data on the prevalence of comorbid obesity and depression in the general practice setting. The frequency with which comorbid depression and obesity occur is important because of the implications for its detection and management. For example, some somatic criteria for the diagnosis of depression, such as sleep problems, fatigue, or changes in appetite, could be confounded with manifestations of obesity.[ 9 ] Depressed people with overweight are difficult to manage; however, little decrease in their body weight had remarkably helped improving their depression.[ 10 ] While interventions for weight management, such as exercise and lifestyle modification may also be helpful in managing depression, there are no set protocols for providing integrated treatment of these health conditions.
This study aimed to examine the risk of depression among overweight and obese individuals in a large station of Armed Forces and associate depression with other risk factors.
MATERIALS AND METHODS
This is a cross-sectional (prospective) descriptive study conducted in the general outpatient department (OPD) of a large station of Armed Forces.
The study population was overweight and obese personnel registered in polyclinic (calculated according to the BMI) who were ≥18 years of age and who fulfilled both inclusion criteria. Inclusion criteria were all personnel whose BMI was ≥25 and who were willing to participate in the study. From a previous study, the prevalence of depression among obese participants was 23%,[ 11 ] which sample size was calculated to be 106 taking the absolute error of margin of 8% with 95% confidence interval (CI).
Instruments
After taking their informed consent, data were collected by self-administered/interviewer assisted (in case of insufficient ability) Patient Health Questionnaire (PHQ-9) to assess the risk of depression. The PHQ-9 has been extensively used by medical personnel in the primary care setting.[ 12 ] A total score of ≥10 to classify depression is considered the best balance between sensitivity (88%) and specificity (88%) compared to mental health professional assessment, and this cutoff value was used for this study.[ 13 ]
Social-demographic characteristics
Self-administered data were obtained for age category, rank, service years, education status, marital status, status of living with family, past field postings, and habit of drinking alcohol.
Body mass index
Participants were asked to report their weight to the nearest kilogram and their height to the nearest centimeter. BMI was calculated, and World Health Organization definitions applied to categories overweight (25–29.9 kg/m 2 ) and obese (>30 kg/m 2 ).[ 14 ] Overweight and obese individuals were included in the study.
The PHQ-9 was used to assess the risk of depression. It is scored on a 27-point scale. Severity of risk of depression was graded according to the following scores: (0–4) – no risk; score (5–10) – mild risk; score (10–14) – moderate risk; and score (>15) – severe risk of depression.[ 13 ]
Statistical analysis
Data entry and statistical analysis were conducted using the Statistical Package for the Social Sciences program version 21 (IBM Corporation, Chichago USA). Descriptive analysis was performed including frequencies, percentages, and confidence intervals. Binary logistic regression model was used to examine association between predictor variables (as captured by data capturing form) and risk of depression. The main model consists of the following predictor variables: demographic variables such as age, service years, rank, education, marital status, living status, and habit of drinking alcohol. Results were expressed as odds ratio (OR) and 95% CIs. In this study, the level of significance was set at P < 0.05 for all the analyses.
A total of 106 study population including 71 (67%) overweight, and 35 (33%) obese were evaluated. The mean age ± standard deviation of patients was 37.73 ± 9.3 years with a minimum age of 25 years and maximum age of 56 years. Among the study population, 82 (77.4%) were of the rank sergeant and below and 24 (22.6%) were warrant officers and above with mean service years of approximately 18 years. More than half of the study population was graduates (75.5%). Almost 80% of individuals were married, and 74.5% of them were living with family. A majority of the respondents (63%) had the habit of drinking alcohol. Among the study population, 67% of them were overweight, and 33% of them were obese with a mean BMI of 27.9 kg/m 2 [ Table 1 ].
Demographic characteristics of the study population
Characteristics | =106 | % |
---|---|---|
Age (yr) | ||
Upto 30 | 33 | 31.1 |
31 and above | 73 | 68.9 |
Rank | ||
Sgt & below | 82 | 774 |
WO & Above | 24 | 22.6 |
Service years | ||
Upto 20 | 65 | 61.3 |
21 & above | 41 | 38.7 |
Education | ||
Primary School | 5 | 4.7 |
Middle School | 3 | 2.8 |
High School | 18 | 17 |
Graduate | ||
Field postings | 80 | 75.5 |
Yes | 53 | 50 |
No | ||
Marital Status | 53 | 50 |
Married | 92 | 86.8 |
Unmarried | 14 | 13.2 |
Living Status | ||
With Family | 79 | 74.5 |
Alone | 27 | 25.5 |
Habit of Drinking Alcohol | ||
Yes | 67 | 63.2 |
No | 39 | 36.8 |
BMI | ||
Overweight | 71 | 67 |
Obese | 35 | 33 |
BMI- Body mass index (kg/m2); SGT - Sergeant; WO- Warrant officer
As per this study, of the individuals assessed, 13 (12%) individuals fulfilled the criteria for risk of moderate depression, 58 (54%) for mild depression, and 35 (33%) individuals had no risk for clinically significant depression. Thus almost half of individuals with overweight or obese were ailing from the risk of mild-to-moderate depression. There was no individual with risk of severe depression. This study is in concurrence with a previous study which found overweight increased the risk of onset of depression.[ 3 ] The prevalence of depression in overweight and obese individuals can be associated with factors such as stigmatization, low self-esteem, and body dissatisfaction[ 11 ] which can contribute to or exacerbate depressive illness. Conversely, features of depression may also cause or exacerbate weight problems and obesity.
On assessing the individuals whether they had any difficulty in job, about 53% of individuals had some difficulty in job, about 20% of them had moderate difficulty in job, and about 25% of them had no difficulty in job [ Table 2 ].
Risk of depression in Study population
Likelihood of depression | =106 | % |
---|---|---|
No risk | 35 | 33 |
Mild risk | 58 | 54.7 |
Moderate risk | 13 | 12.3 |
Severe risk | - | - |
PHQ Score (0-4) - No risk; (5-10)- Moderate risk; (10-14) moderate risk, (>15)- severe risk
The likelihood of depression was most strongly associated with BMI (OR: 6.33; 95% CI: 2.94–13.64; P < 0.001) followed by age (OR: 1.12; 95% CI: 1.08–3.7; P = 0.008), living single (OR: 1.31; 95% CI: 1.06–1.54; P = 0.004), and the habit of drinking alcohol (OR: 1.24; 95% CI: 1.04–1.64; P = 0.041). There was a positive association between weight gain and depression in a previous study also.[ 15 ] Advancing age was associated with depression which was also shown in earlier studies.[ 16 ]
Obesity and depressive disorders are common morbidities with overlapping pathophysiology whose coexistence is associated with adverse health outcomes. In fact, many overweight/obese patients exhibited weight loss after the treatment of depression.[ 10 ]
This study found that large number (54%) of obese patients had a risk of mild depression, and few (12%) had a risk of moderate depression. As per recent literature,[ 9 ] the individuals with risk of mild depression to be treated in community setting itself with lifestyle modification, dietary advice, and involvement of pleasure-seeking activities. Whereas individuals with risk of moderate depression need to be referred to psychological counselor for further investigation and counseling sessions.

The data regarding the prevalence of depression in patients with overweight and obese individuals from India are scarce. However, a previous study from the USA reported that obese were significantly more likely to be depressed than those who were either normal or underweight.[ 17 ]
Regression analysis from the previous studies identified more educated, unmarried group of individuals with worse mental health.[ 17 , 18 ] However, the likelihood of depression was not significant with education status and marital status in this study. This study also showed no significant association with service years, rank, and field postings. The most likely explanation for these disparate results relates to differences in the populations studied and the procedures used, particularly the measures of depression.
Exploration into the most effective ways of discovering the potential comorbidity of obesity and depression will help to assist in developing interventional approaches to effectively target these conditions simultaneously or sequentially.
The PHQ-9 has been shown to have high sensitivity and specificity in the general practice setting. However, it is a screening tool and does not provide a clinical diagnosis of depression. Therefore, there is likely to be some degree of measurement error in the rates of depression reported for each BMI category. Cross-sectional nature of this study precluded an examination of the alternate hypothesis that depression increases the risk for obesity.
RECOMMENDATIONS
It is recommended that every individual with overweight/obesity should once be screened by the PHQ-9 questionnaire by the authorized medical attendant at the OPD level and should be analyzed for the risk of depression. Individuals who are detected to have moderate-to-severe risk of depression should not only undergo lifestyle modifications but also be enrolled in a combined comprehensive obesity and depression control program, which will include the systemic series of counseling sessions by a trained psychological counselor and should be followed up till the individual is benefited. The benefit of interpersonal and psychotherapy may also be given by referring them to psychiatry centers. This will improve not only the overall functional status including mental, emotional, and social well-being but also their health perception and quality of life. It will also prevent cognitive dysfunction, increased days of work absence, early retirement, higher dependence on disability welfare and correspondingly, and improvement in depressive symptoms leading to increase in work productivity.
Financial support and sponsorship
Conflicts of interest.
There are no conflicts of interest.

An official website of the United States government
The .gov means it’s official. Federal government websites often end in .gov or .mil. Before sharing sensitive information, make sure you’re on a federal government site.
The site is secure. The https:// ensures that you are connecting to the official website and that any information you provide is encrypted and transmitted securely.
- Publications
- Account settings
- My Bibliography
- Collections
- Citation manager
Save citation to file
Email citation, add to collections.
- Create a new collection
- Add to an existing collection
Add to My Bibliography
Your saved search, create a file for external citation management software, your rss feed.
- Search in PubMed
- Search in NLM Catalog
- Add to Search
Depression and obesity: evidence of shared biological mechanisms
Affiliations.
- 1 Department of Psychiatry, Amsterdam Neuroscience and Amsterdam Public Health Research Institute, VU University Medical Center, Amsterdam, The Netherlands.
- 2 Laureate Institute for Brain Research, Tulsa, OK, USA.
- 3 School of Community Medicine, The University of Tulsa, Tulsa, OK, USA.
- 4 Department of Internal Medicine, Division of Endocrinology, Erasmus University Medical Center Rotterdam, and Obesity Center CGG, Rotterdam, The Netherlands.
- 5 Department of Psychiatry, Amsterdam Neuroscience and Amsterdam Public Health Research Institute, VU University Medical Center, Amsterdam, The Netherlands. [email protected].
- PMID: 29453413
- DOI: 10.1038/s41380-018-0017-5
Depression and obesity are common conditions with major public health implications that tend to co-occur within individuals. The relationship between these conditions is bidirectional: the presence of one increases the risk for developing the other. It has thus become crucial to gain a better understanding of the mechanisms responsible for the intertwined downward physiological spirals associated with both conditions. The present review focuses specifically on shared biological pathways that may mechanistically explain the depression-obesity link, including genetics, alterations in systems involved in homeostatic adjustments (HPA axis, immuno-inflammatory activation, neuroendocrine regulators of energy metabolism including leptin and insulin, and microbiome) and brain circuitries integrating homeostatic and mood regulatory responses. Furthermore, the review addresses interventional opportunities and questions to be answered by future research that will enable a comprehensive characterization and targeting of the biological links between depression and obesity.
PubMed Disclaimer
Similar articles
- Shared biological mechanisms of depression and obesity: focus on adipokines and lipokines. Fu X, Wang Y, Zhao F, Cui R, Xie W, Liu Q, Yang W. Fu X, et al. Aging (Albany NY). 2023 Jun 29;15(12):5917-5950. doi: 10.18632/aging.204847. Epub 2023 Jun 29. Aging (Albany NY). 2023. PMID: 37387537 Free PMC article. Review.
- Mechanisms linking depression co-morbid with obesity: An approach for serotonergic type 3 receptor antagonist as novel therapeutic intervention. Kurhe Y, Mahesh R. Kurhe Y, et al. Asian J Psychiatr. 2015 Oct;17:3-9. doi: 10.1016/j.ajp.2015.07.007. Epub 2015 Jul 18. Asian J Psychiatr. 2015. PMID: 26243683 Review.
- Metabolic syndrome and major depression. Marazziti D, Rutigliano G, Baroni S, Landi P, Dell'Osso L. Marazziti D, et al. CNS Spectr. 2014 Aug;19(4):293-304. doi: 10.1017/S1092852913000667. Epub 2013 Oct 8. CNS Spectr. 2014. PMID: 24103843 Review.
- HPA-axis stress reactivity in youth depression: evidence of impaired regulatory processes in depressed boys. Lopez-Duran NL, McGinnis E, Kuhlman K, Geiss E, Vargas I, Mayer S. Lopez-Duran NL, et al. Stress. 2015;18(5):545-53. doi: 10.3109/10253890.2015.1053455. Epub 2015 Jun 26. Stress. 2015. PMID: 26115161 Free PMC article.
- Pterostilbene alleviates cafeteria diet-induced obesity and underlying depression in adolescent male Swiss albino mice and affects insulin resistance, inflammation, HPA axis dysfunction and SIRT1 mediated leptin-ghrelin signaling. Patil R, Aswar U, Vyas N. Patil R, et al. Horm Behav. 2024 May;161:105504. doi: 10.1016/j.yhbeh.2024.105504. Epub 2024 Feb 14. Horm Behav. 2024. PMID: 38354494
- Clinical Factors and Biomarkers Associated with Depressive Disorders in Older Patients Affected by Chronic Kidney Disease (CKD): Does the Advanced Glycation End Products (AGEs)/RAGE (Receptor for AGEs) System Play Any Role? Buoli M, Dozio E, Caldiroli L, Armelloni S, Vianello E, Corsi Romanelli M, Castellano G, Vettoretti S. Buoli M, et al. Geriatrics (Basel). 2024 Jul 30;9(4):99. doi: 10.3390/geriatrics9040099. Geriatrics (Basel). 2024. PMID: 39195129
- Correlation of Adiponectin and Leptin with Anthropometrics and Behavioral and Physical Performance in Overweight and Obese Chinese College Students. Sun J, Chen J, Cicchella A. Sun J, et al. Biology (Basel). 2024 Jul 27;13(8):567. doi: 10.3390/biology13080567. Biology (Basel). 2024. PMID: 39194505
- Negative association between Body Roundness Index and bone mineral density: insights from NHANES. Ding Z, Zhuang Z, Tang R, Qu X, Huang Z, Sun M, Yuan F. Ding Z, et al. Front Nutr. 2024 Aug 8;11:1448938. doi: 10.3389/fnut.2024.1448938. eCollection 2024. Front Nutr. 2024. PMID: 39176032 Free PMC article.
- Exploring the possible therapeutic mechanism of Danzhixiaoyao pills in depression and MAFLD based on "Homotherapy for heteropathy": A network pharmacology and molecular docking. Chu Y, Pang B, Yang M, Wang S, Meng Q, Gong H, Kong Y, Leng Y. Chu Y, et al. Heliyon. 2024 Jul 26;10(15):e35309. doi: 10.1016/j.heliyon.2024.e35309. eCollection 2024 Aug 15. Heliyon. 2024. PMID: 39170292 Free PMC article.
- Impact of the microbiome on human, animal, and environmental health from a One Health perspective. Ma LC, Zhao HQ, Wu LB, Cheng ZL, Liu C. Ma LC, et al. Sci One Health. 2023 Aug 25;2:100037. doi: 10.1016/j.soh.2023.100037. eCollection 2023. Sci One Health. 2023. PMID: 39077043 Free PMC article. Review.
- Vos T, Flaxman AD, Naghavi M, Lozano R, Michaud C, Ezzati M, et al. Years lived with disability (YLDs) for 1160 sequelae of 289 diseases and injuries 1990-2010: a systematic analysis for the Global Burden of Disease Study 2010. Lancet. 2012;380:2163–96. - PubMed - PMC
- Effects H. Health effects of overweight and obesity in 195 countries over 25 years. N Engl J Med. 2017;377:13–27. http://www.nejm.org/doi/10.1056/NEJMoa1614362
- Heymsfield SB, Wadden TA. Mechanisms, pathophysiology, and management of obesity. N Engl J Med. 2017;376:254–66. http://www.nejm.org/doi/10.1056/NEJMra1514009 - PubMed
- de Wit L, Luppino F, van Straten A, Penninx B, Zitman F, Cuijpers P. Depression and obesity: a meta-analysis of community-based studies. Psychiatry Res. 2010;178:230–5. https://doi.org/10.1016/j.psychres.2009.04.015 . - DOI - PubMed
- Jung SJ, Woo H, Cho S, Park K, Jeong S, Lee YJ, et al. Association between body size, weight change and depression: systematic review and meta-analysis. Br J Psychiatry. 2017;211:14–21. http://www.ncbi.nlm.nih.gov/pubmed/28428339 [cited 4 Sep 2017] - PubMed
Publication types
- Search in MeSH
Related information
- Cited in Books
- PubChem Compound (MeSH Keyword)
LinkOut - more resources
Full text sources.
- Nature Publishing Group
Other Literature Sources
- scite Smart Citations
- Genetic Alliance
- MedlinePlus Health Information

- Citation Manager
NCBI Literature Resources
MeSH PMC Bookshelf Disclaimer
The PubMed wordmark and PubMed logo are registered trademarks of the U.S. Department of Health and Human Services (HHS). Unauthorized use of these marks is strictly prohibited.
Thank you for visiting nature.com. You are using a browser version with limited support for CSS. To obtain the best experience, we recommend you use a more up to date browser (or turn off compatibility mode in Internet Explorer). In the meantime, to ensure continued support, we are displaying the site without styles and JavaScript.
- View all journals
- Explore content
- About the journal
- Publish with us
- Sign up for alerts
- Open access
- Published: 05 August 2019

Investigating the association between body fat and depression via Mendelian randomization
- Maria S. Speed 1 , 2 , 3 , 4 , 5 ,
- Oskar H. Jefsen ORCID: orcid.org/0000-0002-5831-5158 3 ,
- Anders D. Børglum ORCID: orcid.org/0000-0001-8627-7219 4 , 5 , 6 ,
- Doug Speed 1 , 4 , 5 , 7 &
- Søren D. Østergaard ORCID: orcid.org/0000-0002-8032-6208 2 , 3 , 4 , 5 , 7
Translational Psychiatry volume 9 , Article number: 184 ( 2019 ) Cite this article
12k Accesses
83 Citations
193 Altmetric
Metrics details
- Personalized medicine
- Prognostic markers
Obesity and depression are major public health concerns that are both associated with substantial morbidity and mortality. There is a considerable body of literature linking obesity to the development of depression. Recent studies using Mendelian randomization indicate that this relationship is causal. Most studies of the obesity–depression association have used body mass index as a measure of obesity. Body mass index is defined as weight (measured in kilograms) divided by the square of height (meters) and therefore does not distinguish between the contributions of fat and nonfat to body weight. To better understand the obesity–depression association, we conduct a Mendelian randomization study of the relationship between fat mass, nonfat mass, height, and depression, using genome-wide association study results from the UK Biobank ( n = 332,000) and the Psychiatric Genomics Consortium ( n = 480,000). Our findings suggest that both fat mass and height (short stature) are causal risk factors for depression, while nonfat mass is not. These results represent important new knowledge on the role of anthropometric measures in the etiology of depression. They also suggest that reducing fat mass will decrease the risk of depression, which lends further support to public health measures aimed at reducing the obesity epidemic.
Similar content being viewed by others
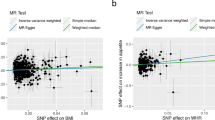
Obesity and atypical depression symptoms: findings from Mendelian randomization in two European cohorts
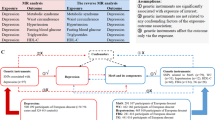
The association between depression and metabolic syndrome and its components: a bidirectional two-sample Mendelian randomization study
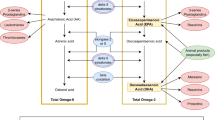
Omega-3 fatty acids and major depression: a Mendelian randomization study
Introduction.
Obesity, defined as abnormal or excessive accumulation of body fat, is a major public health concern 1 as an established risk factor for cardiovascular disease, type II diabetes, certain cancers, and overall decreased life expectancy 2 . Furthermore, observational studies have shown an association between obesity and depression. For example, Luppino et al. 3 found that obese individuals were 55% more likely to develop depression, while depressed individuals were 58% more likely to become obese.
To investigate the observed association between obesity and depression, prior studies have performed Mendelian randomization (MR), a method from genetic epidemiology which uses data from genome-wide association studies (GWAS) to determine whether a risk factor is causal for an outcome 4 . MR studies have indicated that there is a causal relationship going from obesity to depression, but not vice versa 5 , 6 , 7 , 8 . These MR studies measured obesity using body mass index (BMI), which is calculated as weight (measured in kilograms) divided by the square of height (meters).
Although BMI is the most common measure of obesity (for example, an obese individual is generally defined as someone with BMI ≥ 30), its use has been repeatedly criticized 9 , 10 , 11 . Most notably, BMI does not distinguish between fat mass and nonfat mass. This distinction is very important from a physiological perspective, due to the fact that adipose tissue has very different properties compared to muscle and bone, the other major tissues contributing to body weight. In particular, adipose tissue is an endocrine organ that produces a range of adipokines and inflammatory proteins, which have been associated with negative systemic effects that may also affect the brain 12 . Furthermore, BMI does not capture body fat location, and while upper-body and visceral fat, which are more common in men, contribute to the development of an unhealthy cardiometabolic profile 13 , lower body fat, which is more common in women, seems to be protective of this 14 . In addition, how much of an individual’s BMI is due to fat, and where this fat is located, might also be of importance, as these factors can influence body satisfaction and social stigma, with psychological consequences 15 .
The aim of this study was to increase the understanding of the obesity–depression association by assessing the relationship between specific and biologically informative components of BMI (fat mass and nonfat mass, stratified on limbs and trunk) and depression via a MR study using results from large GWAS.
Materials and methods
In total, we consider 21 anthropometric measures: the first six are BMI, weight, height, whole-body fat percentage, whole-body fat mass, and whole-body nonfat mass; the remaining 15 are fat percentage, fat mass and nonfat mass for each of trunk, right arm, left arm, right leg, and left leg. Supplementary Fig. 1 reports the genetic correlations between the 21 measures. We use MR to test whether each measure is a causal risk factor for depression, then to test whether depression is a causal risk factor for each measure.
In order to use MR to test whether one phenotype is a causal risk factor for another, we require GWAS summary statistics for both phenotypes. For the anthropometric traits, we use genome-wide summary statistics provided by the Neale Lab ( http://www.nealelab.is/uk-biobank/ ), who performed association analyses for over 2000 phenotypes from the UK Biobank ( http://www.ukbiobank.ac.uk/ ). The UK Biobank is a population-based cohort of approximately 500,000 individuals; 54% are female, the average age is 57 (range 37–73), while 94% report as being White British. For the anthropometric GWAS, the average sample size is 331,910 (see Table 1 for exact numbers); the analysis was performed using linear regression including ten principal components and sex as covariates.
For depression, we use summary statistics from the most recent GWAS of major depressive disorder (MDD) by the Psychiatric Genomics Consortium (PGC) 6 , available at ( https://www.med.unc.edu/pgc/results-and-downloads ). This study recruited individuals from seven cohorts; they used a relatively liberal definition of MDD, based on self-reporting, clinical assessment, and examination of medical records, with an estimated population prevalence of 15%. The association analysis was performed using logistic regression, including significantly-associated principal components as covariates. The PGC provides two sets of summary statistics: from their main GWAS of 480,359 samples (135,458 cases, 344,901 controls) they report results for 10,000 of the most strongly-associated SNPs; whereas from a “sub-GWAS” of 173,005 samples (59,851 cases and 113,154 controls), they report results for all SNPs. Therefore, when testing whether depression is a causal risk factor for one of the anthropometric measures (which requires only results for significantly-associated SNPs), we use summary statistics from the main GWAS, whereas when testing whether an anthropometric measure is a causal risk factor for depression (which requires genome-wide results), we use summary statistics from the sub-GWAS.
In total, there are 6,568,396 SNPs common to the UK Biobank and PGC GWAS (we excluded SNPs with alleles A & T or C & G, or with info score < 0.9). We test whether an anthropometric measure is causally associated with depression using inverse-weighted regression, for which we use the R package Mendelian randomization 16 . To decide which SNPs to use in this regression, we first identify which have P < 5e−8 for the measure, then thin these until no pair remains within 3 cM with correlation squared >0.05. We use the same strategy to test whether depression is a causal risk factor for an anthropometric measure, except now we identify a set of independent, genome-wide significant SNPs for depression.
A key assumption of MR is no pleiotropy 17 . For example, the SNPs we use when testing the relationship between BMI and depression should be causal for BMI, but not depression. This is difficult to test directly, so instead we perform three sensitivity analyses. Firstly, we repeat the inverse-weighted regression excluding SNPs showing evidence for pleiotropy ( P < 0.05/N, where N is the number of independent, genome-wide significant SNPs). Secondly, we instead assess causality using weighted-median regression, which gives unbiased estimates provided at least 50% of the information comes from non-pleiotropic SNPs. Thirdly, we estimate the intercept from Egger Regression; an intercept significantly different to zero ( P < 0.05) is an indication of directional pleiotropy.
Our primary analysis focuses on BMI, weight, height, whole-body fat mass, whole-body nonfat mass, and whole-body fat percentage; our secondary analysis considers the 15 location-specific measures of fat mass, nonfat mass and fat percentage. As we are performing a total of 42 tests, we set the (conservative) Bonferroni corrected significance threshold at P < 0.05/42 (this is satisfied when the regression slope is ≥3.0 SDs from zero).
Table 1 reports the number of independent, genome-wide significant SNPs for each of the 21 anthropometric measures, and how much of the phenotypic variation each set of SNPs explains. The results of our primary analysis are displayed in Figs. 1 and 2 and Table 2 . Our main conclusions are based on the results from inverse-variance regression using all SNPs (the red lines in each plot), while the remaining three regressions (the orange, green and blue lines) are sensitivity analyses. We note that each time the slope from inverse-variance regression using all SNPs is statistically significant, this finding is supported by the three sensitivity analyses (i.e., the slope remains significant when SNPs showing evidence for pleiotropy are excluded or when we use weighted-median regression, and Egger regression does not find significant directional pleiotropy).
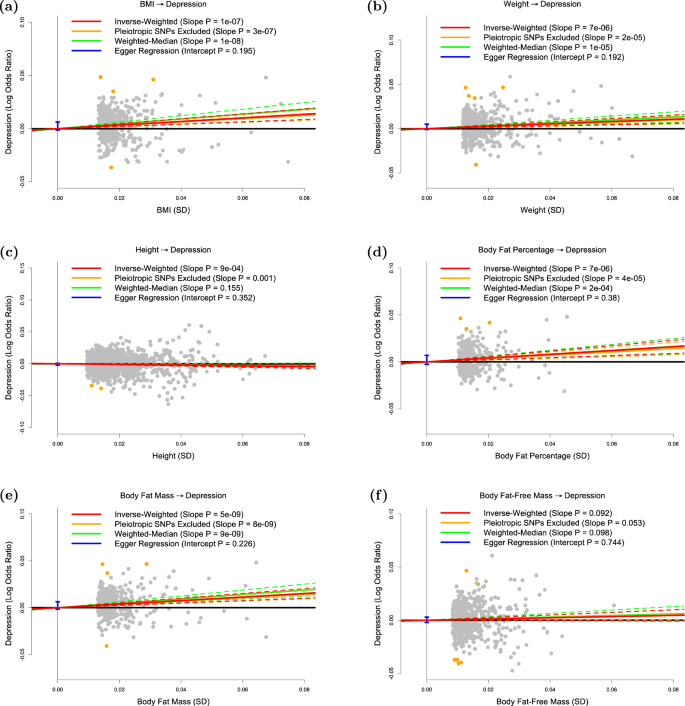
The panels plot per-allele effect sizes for a BMI, b weight, c height, d body fat percentage, e body fat mass and f body fat-free mass ( x -axes) against per-allele effect size for depression ( y -axis) For the anthropometric measures, effect sizes are measured in SDs, for depression the effect size is log-odds ratio. For each plot we estimate the slope using inverse-variance regression (red solid line), inverse-variance regression after excluding SNPs showing evidence for pleiotropy (orange solid line), weighted-median regression (green solid line) and Egger regression (blue solid line). The corresponding colored dashed lines represent the 95% confidence intervals for the slopes, while the vertical blue segment marks a 95% confidence interval for the intercept from Egger regression. The horizontal black solid line indicates no effect
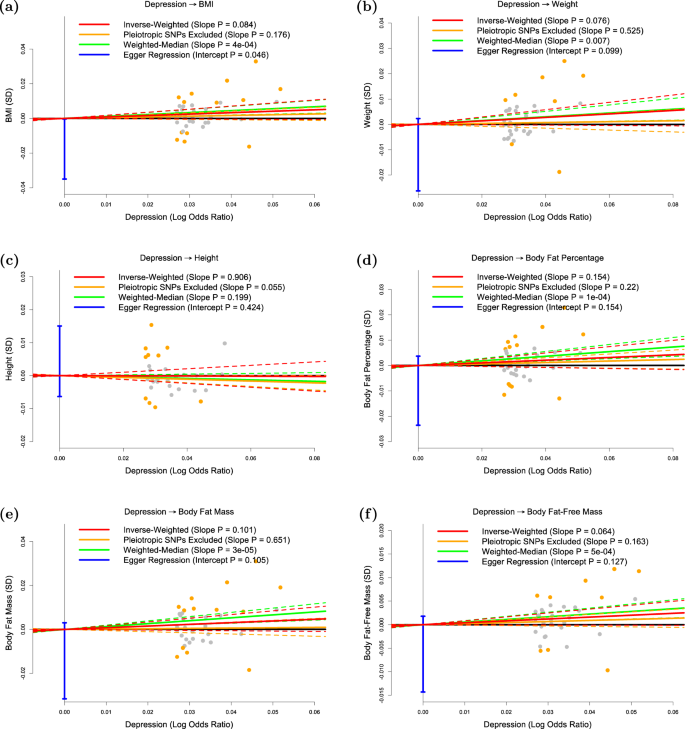
The panels plot per-allele effect size for depression ( x -axis) against per-allele effect sizes for a BMI, b weight, c height, d body fat percentage, e body fat mass and f body fat-free mass ( y -axes). For the anthropometric measures, effect sizes are measured in SDs, for depression the effect size is log-odds ratio. For each plot we estimate the slope using inverse-variance regression (red solid line), inverse-variance regression after excluding SNPs showing evidence for pleiotropy (orange solid line), weighted-median regression (green solid line) and Egger regression (blue solid line). The corresponding colored dashed lines represent the 95% confidence intervals for the slopes, while the vertical blue segment marks a 95% confidence interval for the intercept from Egger regression. The horizontal black solid line indicates no effect
Figure 1a confirms that BMI is a causal risk factor for depression. The estimated slope from inverse-weighted regression is 0.17 (SD 0.03), which is significantly greater than zero ( P = 1e−7) and indicates that a 1-SD increase in BMI corresponds to a 0.17 increase in the log-odds ratio (OR) for depression. Figure 1b indicates there is a causal relationship between weight and depression; however, the estimated slope (0.13, SD 0.03) is less than that for BMI, which supports the current preference to measure obesity using BMI rather than weight. Figure 1c suggests that height (short stature) is a causal risk factor for depression; the estimated slope is −0.06 (SD 0.02), indicating that a 1-SD increase in height corresponds to a 0.06 decrease in the log-odds ratio (OR) for depression.
Figure 1d and e shows that whole-body fat percentage and whole-body fat mass are both causal risk factors for depression; the estimated slopes are very similar (0.20, SD 0.04 and 0.19, SD 0.03, respectively) indicating that there is no advantage to normalizing (i.e., measuring fat percentage instead of fat mass). The estimated slope in Fig. 1f is 0.06 (SD 0.03), indicating that MR finds no evidence that whole-body nonfat is a causal risk factor for depression.
Figure 2 shows that, consistent with previous studies, there is no significant evidence that depression is a causal risk factor for any of the six anthropometric measures investigated here.
Table 3 reports results from our secondary analysis. For each of the 15 location-specific anthropometric measures, the results are consistent with those from the whole-body version; i.e., regardless of whether we consider trunk, right arm, left arm, right leg, or left arm, we again find that fat percentage and fat mass are causally associated with depression, but that nonfat mass is not. For fat percentage and fat mass, we are interested in comparing slope estimates, as significant differences would indicate that risk of developing depression depends on fat location. The largest difference is observed for fat percentage, where the estimated slope for left leg (0.34, SD 0.06) is approximately twice that for trunk (0.16, SD 0.04); however, although this difference is nominally significance ( P = 0.01), it is not significant after correction for multiple comparisons.
We have used MR to investigate the causal relationship between different anthropometric measures and depression. We first confirmed that BMI is a causal risk factor for depression, but found no significant evidence that depression causes increased BMI. These results are in line with recent MR studies reporting evidence that higher BMI causally increases the risk of depression, but not the reverse 5 , 6 , 7 , 8 .
Our main finding is that body fat mass is a causal risk factor for depression, but that body nonfat mass is not, therefore indicating that the BMI-depression causality is driven by fat. We note from Table 1 that the number of genome-wide associated SNPs for whole-body nonfat ( n = 667) and the proportion of variance they explain (11.9%) are higher than the corresponding values for fat percentage ( n = 336 and 4.9%) and for fat mass ( n = 387 and 5.8%), indicating that MR finding no evidence for a causal relationship was not simply a power issue.
We also investigated whether the strength of the causal relationship between body fat and depression depended on the location, but these results were inconclusive; while the point estimates suggested that leg fat more strongly affects risk of depression than either trunk fat or arm fat, the difference was not significant after correcting for multiple comparisons.
The causal relationship going from fat mass to depression is likely to have both psychological and biological components. Psychologically, perceived weight discrimination, stigmatization, and body image dissatisfaction may mediate the causality; 18 , 19 , 20 , 21 biologically, obesity is associated with several endocrine and metabolic changes that have been linked to depression, including altered glucocorticoid, adipokine, insulin, leptin, and inflammatory signaling 22 . Although our study was not aimed at providing insight into how fat increases the risk of depression, the finding that trunk fat mass was not more strongly associated with depression risk than fat mass on the limbs (rather we found a tendency towards the opposite), seems to be in favor of a psychological mechanism—since trunk fat is considered the more metabolically adverse 13 .
Observational studies have attempted to separate the psychological and physiological components in the relationship between obesity and depression. In support of a physiological component, Jokela et al. 23 found that the risk of depressive symptoms associated with obesity increased almost linearly with the number of metabolic risk factors; in particular, obese individuals who were metabolically unhealthy had a higher depression risk than obese individuals who were metabolically healthy (OR = 1.23; 95% CI: 1.05, 1.45). However, the same study also found that metabolically-healthy individuals who were obese had a higher depression risk than metabolically-healthy individuals who were not obese (OR = 1.29; 95% CI: 1.12, 1.50), indicating that metabolic factors only partially explain the increase in depression risk associated with obesity. Similar results were found by the longitudinal study of Hamer et al. 24 .
A recent study by Tyrrell et al. 8 also sought to separate the psychological component of obesity from its adverse metabolic consequences—and employed MR to do so. Specifically, Tyrrell et al. used two genetic instruments, that both represented BMI, but one with and one without its adverse metabolic consequences. They found that both instruments were associated with increased risk of depression - suggesting that the causal association between BMI and depression is primarily driven by psychological consequences of adiposity and not by its adverse metabolic effects 8 . This conclusion resonates well with the results of our study.
In addition to the results on the impact of body fat on the risk of depression, we also found evidence suggesting that height (short stature) is a causal risk factor for depression. Several large observational studies have found short stature to be associated with poorer mental health 25 , lower health-related quality of life 26 , depressive symptoms in adolescents 27 and adults 28 , 29 , and suicide in men 30 . Other studies have found no association between height and depression or suicide 31 or negligible effects of height on mental health 32 . The association between short stature and poor mental health may be explained by confounding factors, such as socioeconomic status, prenatal development, or childhood factors, or by a causal effect of height on depression risk 33 , 34 . Our results indicate that at least part of the association between short stature and depression is indeed due to a direct causal effect.
Finally, we note some limitations of our work. Firstly, the MR estimates rely on three key assumptions: (i) the SNPs used as genetic predictors for a trait are causal for that trait; (ii) these SNPs are not associated with confounders of the trait-outcome association; (iii) these SNPs only affect the outcome through the causal relationship (i.e., there is no pleiotropy). We can be confident of (i) because our genetic predictors only used SNPs robustly-associated ( P < 5e−8) with the trait, while (ii) should be true due to the fact an individual’s genotypes are randomly allocated during gamete formation. It is hard to explicitly test (iii), however, our sensitivity analyses indicate that our conclusions are not the consequence of pleiotropy.
Secondly, the UK Biobank measured fat and nonfat mass via bioelectrical impedance analysis (using a Tanita BC418MA body composition analyzer), which is considered less accurate than techniques such as dual-energy x-ray absorptiometry. However, we would expect measurement error to cause the estimates for fat and nonfat to become more similar, so the fact that we observed a significant difference indicates that the UK Biobank measurements were sufficiently accurate for our purpose.
Thirdly, we note that some samples were common to both the anthropometric and MDD GWAS (the PGC GWAS used approximately 30,000 individuals from the UK Biobank). Although this overlap is likely to have only a small impact 35 , ideally, our analysis would be repeated using summary statistics from completely independent GWAS.
Fourthly, while we found only suggestive evidence that location of fat affects risk of depression, we recognize that the high correlations between measurements taken in the trunk, arms, and legs (Supplementary Fig. 1 ) would have reduced our power to detect significant differences.
Fifthly, there may be sex-specific psychological and physiological factors affecting the obesity–depression relationship. While we were not able to test sex differences, because the PGC did not release results from male-only and female-only GWAS of depression, this is worthy of further study.
In conclusion, the present study provides evidence that the causal relationship between BMI and depression is driven by fat mass and height, and not by nonfat mass. These results represent important new knowledge on the role of anthropometric measures in the etiology of depression. They also suggest that reducing fat mass will decrease the risk of depression, which lends further support to public health measures aimed at reducing the obesity epidemic.
Swinburn, B. A. et al. The global obesity pandemic: shaped by global drivers and local environments. Lancet 378 , 804–814 (2011).
Article Google Scholar
Haslam, D. W. & James, W. P. Obesity. Lancet 366 , 1197–1209 (2005).
Luppino, F. S. et al. Overweight, obesity, and depression: a systematic review and meta-analysis of longitudinal studies. Arch. Gen. Psychiatry 67 , 220–229 (2010).
Lawlor, D. A., Harbord, R. M., Sterne, J. A., Timpson, N. & Davey Smith, G. Mendelian randomization: using genes as instruments for making causal inferences in epidemiology. Stat. Med. 27 , 1133–1163 (2008).
Hartwig, F. P. et al. Body mass index and psychiatric disorders: a Mendelian randomization study. Sci. Rep. 6 , 32730 (2016).
Article CAS Google Scholar
Wray, N. R. et al. Genome-wide association analyses identify 44 risk variants and refine the genetic architecture of major depression. Nat. Genet. 50 , 668–681 (2018).
van den Broek, N. et al. Causal associations between body mass index and mental health: a Mendelian randomisation study. J. Epidemiol. Community Health 72 , 708–710 (2018).
Tyrrell, J. et al. Using genetics to understand the causal influence of higher BMI on depression. Int. J. Epidemiol 48 , 834–848 (2019).
Kopelman, P. G. Obesity as a medical problem. Nature 404 , 635–643 (2000).
Nuttall, F. Q. Body mass index: obesity, BMI, and health: a critical review. Nutr. Today 50 , 117–128 (2015).
Wellens, R. I. et al. Relationships between the Body Mass Index and body composition. Obes. Res. 4 , 35–44 (1996).
Ouchi, N., Parker, J. L., Lugus, J. J. & Walsh, K. Adipokines in inflammation and metabolic disease. Nat. Rev. Immunol. 11 , 85–97 (2011).
Tchernof, A. & Despres, J. P. Pathophysiology of human visceral obesity: an update. Physiol. Rev. 93 , 359–404 (2013).
Karpe, F. & Pinnick, K. E. Biology of upper-body and lower-body adipose tissue-link to whole-body phenotypes. Nat. Rev. Endocrinol. 11 , 90–100 (2015).
Berner, L. A., Arigo, D., Mayer, L. E., Sarwer, D. B. & Lowe, M. R. Examination of central body fat deposition as a risk factor for loss-of-control eating. Am. J. Clin. Nutr. 102 , 736–744 (2015).
Yavorska, O. O. & Burgess, S. Mendelian Randomization: an R package for performing Mendelian randomization analyses using summarized data. Int. J. Epidemiol. 46 , 1734–1739 (2017).
Zheng, J. et al. Recent developments in Mendelian Randomization studies. Curr. Epidemiol. Rep. 4 , 330–345 (2017).
Levy, B. R. & Pilver, C. E. Residual stigma: psychological distress among the formerly overweight. Soc. Sci. Med. (1982) 75 , 297–299 (2012).
Hunger, J. M. & Major, B. Weight stigma mediates the association between BMI and self-reported health. Health Psychol. 34 , 172–175 (2015).
Robinson, E., Sutin, A. & Daly, M. Perceived weight discrimination mediates the prospective relation between obesity and depressive symptoms in U.S. and U.K. adults. Health Psychology 36 , 112–121 (2017).
Stevens, S. D., Herbozo, S., Morrell, H. E., Schaefer, L. M. & Thompson, J. K. Adult and childhood weight influence body image and depression through weight stigmatization. J. Health Psychol. 22 , 1084–1093 (2017).
Hryhorczuk, C., Sharma, S. & Fulton, S. E. Metabolic disturbances connecting obesity and depression. Front. Neurosci. 7 , 177 (2013).
Jokela, M., Hamer, M., Singh-Manoux, A., Batty, G. D. & Kivimaki, M. Association of metabolically healthy obesity with depressive symptoms: pooled analysis of eight studies. Mol. Psychiatry 19 , 910–914 (2014).
Hamer, M., Batty, G. D. & Kivimaki, M. Risk of future depression in people who are obese but metabolically healthy: the English longitudinal study of ageing. Mol. Psychiatry 17 , 940–945 (2012).
Cheung, Y. B. et al. Height and mental health and health utility among ethnic Chinese in a polyclinic sample in Singapore. Ann. Acad. Med. 42 , 73–79 (2013).
Google Scholar
Christensen, T. L., Djurhuus, C. B., Clayton, P. & Christiansen, J. S. An evaluation of the relationship between adult height and health-related quality of life in the general UK population. Clin. Endocrinol. 67 , 407–412 (2007).
Rees, D. I., Sabia, J. J. & Argys, L. M. A head above the rest: height and adolescent psychological well-being. Econ. Hum. Biol. 7 , 217–228 (2009).
Montgomery, S. M., Netuveli, G., Hildon, Z. & Blane, D. Does financial disadvantage at older ages eliminate the potential for better health? J. Epidemiol. Community Health 61 , 891–895 (2007).
Osika, W. & Montgomery, S. M. Economic disadvantage modifies the association of height with low mood in the US, 2004: the disappointment paradox. Econ. Hum. Biol. 6 , 95–107 (2008).
Magnusson, P. K., Gunnell, D., Tynelius, P., Davey Smith, G. & Rasmussen, F. Strong inverse association between height and suicide in a large cohort of Swedish men: evidence of early life origins of suicidal behavior? Am. J. Psychiatry 162 , 1373–1375 (2005).
Bjerkeset, O., Romundstad, P., Evans, J. & Gunnell, D. Association of adult body mass index and height with anxiety, depression, and suicide in the general population: the HUNT study. Am. J. Epidemiol. 167 , 193–202 (2008).
Coste, J., Pouchot, J. & Carel, J. C. Height and health-related quality of life: a nationwide population study. J. Clin. Endocrinol. Metab. 97 , 3231–3239 (2012).
Batty, G. D. et al. Height, wealth, and health: an overview with new data from three longitudinal studies. Econ. Hum. Biol. 7 , 137–152 (2009).
Perkins, J. M., Subramanian, S. V., Davey Smith, G. & Ozaltin, E. Adult height, nutrition, and population health. Nutr. Rev. 74 , 149–165 (2016).
Burgess, S., Davies, N. M. & Thompson, S. G. Bias due to participant overlap in two-sample Mendelian randomization. Genet. Epidemiol. 40 , 597–608 (2016).
Download references
Acknowledgements
D.S. is supported by the European Unions Horizon 2020 Research and Innovation Program under the Marie Skłodowska-Curie grant agreement number 754513, by Aarhus University Research Foundation (AUFF) and the Independent Research Fund Denmark (7025-00094B). A.D.B. is supported by grants from The Lundbeck Foundation (R102-A9118 and R155-2014-1724). Data handling and analysis on the GenomeDK HPC facility was supported by NIMH (1U01MH109514-01 to Michael O’Donovan and A.D.B.). HPC capacity at GenomeDK was provided by the iSEQ center and Center for Genomics and Personalized Medicine, Aarhus, Denmark (grants to A.D.B.). S.D.Ø. is supported by the Independent Research Fund Denmark (7016-00048).
Author information
Authors and affiliations.
Bioinformatics Research Centre, Aarhus University, Aarhus, Denmark
Maria S. Speed & Doug Speed
Department of Affective Disorders, Aarhus University, Aarhus, Denmark
Maria S. Speed & Søren D. Østergaard
Department of Clinical Medicine, Aarhus University, Aarhus, Denmark
Maria S. Speed, Oskar H. Jefsen & Søren D. Østergaard
The Lundbeck Foundation Initiative for Integrative Psychiatric Research, iPSYCH, Aarhus, Denmark
Maria S. Speed, Anders D. Børglum, Doug Speed & Søren D. Østergaard
Center for Genomics and Personalized Medicine, Aarhus, Denmark
Department of Biomedicine and Center for Integrative Sequencing, iSEQ, Aarhus University, Aarhus, Denmark
Anders D. Børglum
Aarhus Institute of Advanced Studies, Aarhus University, Aarhus, Denmark
Doug Speed & Søren D. Østergaard
You can also search for this author in PubMed Google Scholar
Corresponding author
Correspondence to Søren D. Østergaard .
Ethics declarations
Conflict of interest.
The authors declare that they have no conflict of interest.
Additional information
Publisher’s note: Springer Nature remains neutral with regard to jurisdictional claims in published maps and institutional affiliations.
Supplementary information
Supplementary figure 1, rights and permissions.
Open Access This article is licensed under a Creative Commons Attribution 4.0 International License, which permits use, sharing, adaptation, distribution and reproduction in any medium or format, as long as you give appropriate credit to the original author(s) and the source, provide a link to the Creative Commons license, and indicate if changes were made. The images or other third party material in this article are included in the article’s Creative Commons license, unless indicated otherwise in a credit line to the material. If material is not included in the article’s Creative Commons license and your intended use is not permitted by statutory regulation or exceeds the permitted use, you will need to obtain permission directly from the copyright holder. To view a copy of this license, visit http://creativecommons.org/licenses/by/4.0/ .
Reprints and permissions
About this article
Cite this article.
Speed, M.S., Jefsen, O.H., Børglum, A.D. et al. Investigating the association between body fat and depression via Mendelian randomization. Transl Psychiatry 9 , 184 (2019). https://doi.org/10.1038/s41398-019-0516-4
Download citation
Received : 13 February 2019
Revised : 14 May 2019
Accepted : 20 June 2019
Published : 05 August 2019
DOI : https://doi.org/10.1038/s41398-019-0516-4
Share this article
Anyone you share the following link with will be able to read this content:
Sorry, a shareable link is not currently available for this article.
Provided by the Springer Nature SharedIt content-sharing initiative
This article is cited by
Body composition, lifestyle, and depression: a prospective study in the uk biobank.
BMC Public Health (2024)
Brain-derived neurotrophic factor and C-reactive protein (CRP) biomarkers in suicide attempter and non-attempter major depression disorder (MDD) patients
- Seyed Hassan Saadat
- Mohammad Javanbakht
- Shima Shahyad
Annals of General Psychiatry (2024)
Familial confounding of internalising symptoms and obesity in adolescents and young adults; a co-twin analysis
- Alexander Charles Campbell
- Lucas Calais-Ferreira
- Jesse T. Young
International Journal of Obesity (2024)
Composite healthy lifestyle, socioeconomic deprivation, and mental well-being during the COVID-19 pandemic: a prospective analysis
Molecular Psychiatry (2024)
Childhood obesity and adolescent follow-up depressive symptoms: exploring a moderated mediation model of body esteem and gender
- Lucia Beltrán-Garrayo
- Junilla K. Larsen
- Ana Rosa Sepúlveda
European Child & Adolescent Psychiatry (2024)
Quick links
- Explore articles by subject
- Guide to authors
- Editorial policies


IMAGES
VIDEO
COMMENTS
Research has revealed an association between depression and obesity, with the prevalence of depression in obese individuals being twice as high as in those of normal weight [ 5 ].
Obesity and depression are disorders with a high prevalence and an extraordinary effect over global morbidity and mortality. The relationship between both disorders has been analysed in scientific literature, obtaining significant associations but also contradictory results.
Many recent lines of scientific evidence indicate that depression and obesity are not independent. In fact, there is strong reason to believe that these conditions are interconnected...
Globally, the prevalence of depression and obesity are important public health concerns, with an estimated 350 and 500 million people suffering, respectively. [ 1, 2] A recent meta-analysis of 17 community-based cross-sectional studies among adults showed a positive overall association between depression and obesity. [ 3] .
The present review focuses specifically on shared biological pathways that may mechanistically explain the depression-obesity link, including genetics, alterations in systems involved in homeostatic adjustments (HPA axis, immuno-inflammatory activation, neuroendocrine regulators of energy metabolism including leptin and insulin, and microbiome ...
Most studies of the obesity–depression association have used body mass index as a measure of obesity. Body mass index is defined as weight (measured in kilograms) divided by the square of...