- Open access
- Published: 28 January 2023

Forex market forecasting using machine learning: Systematic Literature Review and meta-analysis
- Michael Ayitey Junior 1 ,
- Peter Appiahene 1 ,
- Obed Appiah 1 &
- Christopher Ninfaakang Bombie 1
Journal of Big Data volume 10 , Article number: 9 ( 2023 ) Cite this article
33k Accesses
11 Citations
5 Altmetric
Metrics details
When you make a forex transaction, you sell one currency and buy another. If the currency you buy increases against the currency you sell, you profit, and you do this through a broker as a retail trader on the internet using a platform known as meta trader. Only 2% of retail traders can successfully predict currency movement in the forex market, making it one of the most challenging tasks. Machine learning and its derivatives or hybrid models are becoming increasingly popular in market forecasting, which is a rapidly developing field.
While the research community has looked into the methodologies used by researchers to forecast the forex market, there is still a need to look into how machine learning and artificial intelligence approaches have been used to predict the forex market and whether there are any areas that can be improved to allow for better predictions. Our objective is to give an overview of machine learning models and their application in the FX market.
This study provides a Systematic Literature Review (SLR) of machine learning algorithms for FX market forecasting. Our research looks at publications that were published between 2010 and 2021. A total of 60 papers are taken into consideration. We looked at them from two angles: I the design of the evaluation techniques, and (ii) a meta-analysis of the performance of machine learning models utilizing evaluation metrics thus far.
The results of the analysis suggest that the most commonly utilized assessment metrics are MAE, RMSE, MAPE, and MSE, with EURUSD being the most traded pair on the planet. LSTM and Artificial Neural Network are the most commonly used machine learning algorithms for FX market prediction. The findings also point to many unresolved concerns and difficulties that the scientific community should address in the future.
Based on our findings, we believe that machine learning approaches in the area of currency prediction still have room for development. Researchers interested in creating more advanced strategies might use the open concerns raised in this work as input.
Introduction
The foreign exchange or forex market is the largest financial market in the world where currencies are bought and sold simultaneously. It is even larger than the stock market; According to the 2019 Triennial Central Bank Survey of FX and Over-the-Counter (OTC) derivatives markets, it has a daily volume of $6.6 trillion [ 7 ]. It is a non-centralized market that operates 24 h a day except the weekend, which makes it unique from other financial markets. Because of its high volatility, nonlinearity, and irregularity, the forex market, unlike stocks, is one of the most complex markets [ 1 ]. The traits of Forex show differences compared to the stock, bond, and other financial markets. These differences make forex traders have more trading opportunities and advantages for profitable trades. Some of these advantages include no commissions, no middlemen, no fixed lot size, low transaction costs, high liquidity, almost instantaneous transactions, low margins/high leverage, 24-h operations, no insider trading, limited regulation, and online trading opportunities [ 73 ]. In the forex market, currency pairs are traded, with the base currency being the first listed currency and the quote currency being the second. Currency pairs compare the value of one currency to another (the base currency to the quote currency). When the prices depreciate, a quote currency is bought against the base currency, which leads to profit, and when the prices elevate, the base currency is bought against the quote currency [ 1 ]. Two main types of techniques are used to forecast future values for a typical financial time series, which are fundamental analysis and technical analysis. Fundamental analysis is a method of examining economic, social, and political issues that may influence currency prices in the forex market. In contrast, technical analysis involves using historical data price chart, which provides a roadmap for past price behavior. To forecast the future, a technical analyst looks to the past. Predicting the direction of a currency pair's movement is the most important choice in Forex. Predicting currency movement correctly can bring many benefits to traders and vice versa. In past and recent years, the research community has been highly active in predicting the forex market using machine-learning models. On one hand, many verifiable types of research have been conducted with the aim of understanding and predicting currency trends in the forex market using machine-learning models. According to Zhelev and Avresky [ 77 ], the cited literature in the field of deep learning is a basic foundation for solving the challenging problem of prediction of forex price. While the research community has spent a lot of time studying the methodologies used by researchers and practitioners in the context of predictive models in the forex market, there isn't much information on how to forecast currency pair movement in the forex market using machine-learning models and Meta-Analysis. To address this gap in knowledge, we conducted a Systematic Literature Review (SLR) on the use of machine learning (ML) techniques for forex market forecasting, with the goal of (i) understanding and summarizing current algorithms and models, and (ii) analyzing its evaluation metrics and open challenges to guide future research. Our SLR is to provide a complete examination of I machine learning as it has been considered in previous research, and (ii) the training processes used to train and assess machine learning algorithms. We also give a meta-analysis of the performance of the machine learning models that have been developed so far, as judged by their assessment criteria. In addition to examining the state of the art, we critically examined the approaches that have been applied thus far.
Research questions posed for our systematic literature review
Research Question | Motivation |
---|---|
1. For forecasting, what machine learning algorithm was used? | To look into the most up-to-date machine learning approaches for forecasting the FX market that has been considered so far |
2. What dataset was used to train the model, the period and timeframe considered in the literature | In terms of machine learning algorithms, examine the machine learning parameters employed in previous studies. The answers to these questions will help practitioners and researchers figure out the best machine learning configuration for FX market forecasting: which training technique will produce the greatest forecasting results |
3. Evaluation setup i. What types of validation techniques were exploited? ii. What evaluation measures were employed to get access to the prediction models? | |
3. Performance Meta-Analysis: using evaluation metrics of the selected studies | Examine the approaches studied to (i) validate and (ii) assess the proposed forex market forecasting models |
Related research
Our objective is to conduct a systematic literature review to comprehend and summarize studies on machine learning prediction models in the forex market. It's worth noting, however, that some secondary research on machine learning algorithms and deep learning has been proposed. [ 18 , 27 , 44 , 54 , 59 ].
According to Fletcher [ 18 ] when including advanced exogenous financial information to estimate daily FX carry basket returns, committees of discriminative techniques such as Support Vector Machines (SVM), Relevance Vector Machines (RVM), and Neural Networks) perform well.
Panda et al. [ 44 ] conducted a second SLR on Exchange Rate Prediction utilizing ANN and Deep Learning Methodologies, and offered novel approaches that were distinct according to them from 2000 to 2019, for predicted exchange rate projection the effects observed during the protected period within examined are displayed using newly proposed models such as Artificial Neural Network (ANN), Functional Link Artificial Neural Network (FLANN), Hidden Markov Model (HMM),
Support Vector Regression (SVR), an Auto-Regressive (AR) model. Some of the suggested novel neural network models for forecasting, on the other hand, took into account theoretical support and a methodical approach in model creation. This results in the transmission of new deep neural network models.
Islam et al. [ 27 ] conducted a SLR, which looked at recent advances in FOREX currency prediction using machine-learning algorithms. They utilized a keyword-based search approach to filter out popular and relevant research from papers published between 2017 and 2019. They also used a selection algorithm to decide which papers should be included in the review. They analyzed 39 research articles published on "Elsevier," "Springer," and "IEEE Xplore" that forecasted future FOREX prices within the specified time frame based on the selection criteria. According to their findings, in recent years, academics have been particularly interested in neural network models, pattern-based approaches, and optimization methodologies. Many deep learning algorithms, like the gated recurrent unit (GRU) and long short-term memory (LSTM), have been thoroughly investigated and show great promise in time series prediction.
Evaluating the Performance of Machine Learning Algorithms in Financial Market Forecasting was the subject of Ryll & Seidens [ 54 ] study, more than 150 related publications on utilizing machine learning in financial market forecasting were reviewed in this study. They created a table across seven primary factors outlining the experiments done in the studies based on a thorough literature review. They provide a simple, standardized syntax for textually describing machine-learning algorithms by listing and classifying distinct algorithms. They conducted rank analyses to analyze the comparative performance of different algorithm classes based on performance criteria acquired from publications included in the survey. In financial market forecasting, machine-learning algorithms beat most classic stochastic methods, according to their findings. They also discovered evidence that recurrent neural networks outperform feed-forward neural networks and support vector machines on average, implying that there are exploitable temporal relationships in financial time series across asset classes and countries. The same is true when comparing the benefits of different machine learning architectures.
Sezer et al. [ 59 ] did a thorough evaluation of DL studies for financial time series forecasting implementations. Convolutional Neural Networks (CNNs), Deep Belief Networks (DBNs), and Long-Short Term Memory (LSTM) were used to categorize the papers. Their findings show that, despite the fact that financial forecasting has a lengthy study history, overall interest in the DL community is increasing as a result of the use of new DL models,thus, there are numerous chances for researchers. They also attempted to predict the field's future by highlighting potential bottlenecks and opportunities in order to aid interested scholars.
Berradi et al. [ 6 ] suggested that giving the latest research of deep learning techniques applied to the financial market field can help investors to make an accurate decision. They gathered all the recent articles related to deep learning techniques applied to forecasting the financial market, which includes the stock market, stock index, commodity forecasting, and Forex. Their main goal was to find the most models used recently to solve the prediction problem using RNN, their characteristics, and their novelty. They gave all aspects that involve the process of forecasting beginning with preprocessing, the input features, the deep learning techniques, and the evaluation metrics employed. Their finding is that the hybrid model outperforms the traditional machine learning techniques, which leads to the conclusion that there is a very strong relationship between the combination of all the approaches and better prediction performance.
The goal of Henrique et al. [ 23 ] is to present methods for selecting the most important advances in machine learning applied to financial market prediction to present a review of the articles chosen, clarify the knowledge flow that the literature follows, and propose a classification for the articles. In addition, their study provides an overview of the best approaches for applying machine learning to financial time series forecasting as determined by the literature. The publications were then objectively assessed and categorized into the following categories: markets utilized as test data sources, predictive variables, predicted variables, methodologies or models, and performance metrics used in comparisons. In all, 57 papers from 1991 to 2017 were examined and categorized, spanning the specialist literature. Based on searches of connected article databases, no reviews employing such objective methodologies as main route analysis on the topic provided here were discovered according to them. The most cited articles, those with the highest bibliometric coupling and co-citation frequencies, the most recently published articles, and those that are part of the primary path of the literature studied knowledge flow were all discussed in the study. It should be highlighted that they were objective and straightforward survey methodologies, independent of the researcher's expertise, that could be used not just for preliminary research but also as knowledge validation for seasoned experts. In addition, the prediction algorithms and key performance measures for each article were presented. In addition to using neural and SVM networks, the authors used data from the North American market extensively. Similarly, the majority of the forecasts are based on stock indexes. New suggested models will likely be compared to neural and SVM network benchmarks, using data from the North American market, as one of the probable findings regarding the categorization presented in the research. The examination of the behavior of forecasts in developing markets, such as those of the BRICS, as well as the application of novel models in financial market prediction, continues to provide research opportunities.
Kaushik [ 32 ] presents a comprehensive review of contemporary research on Machine Learning and Deep Learning for exchange rate forecasting, based on peer-reviewed publications and books. The paper examines how Machine Learning and Deep Learning algorithms vary in projecting exchange rates in the FOREX market. SVM, Deep learning approaches such as Feedforward Neural networks, and hybrid ensembles have superior prediction accuracy than standard time series models, according to research. Future research should be conducted to assess the performance of these models, according to the authors,however, no single forecasting model consistently stands out as the best when evaluated using different criteria and on different currency pairs, and decisions based on the models' predictions should be used with caution.
Regarding the publications mentioned above, it's worth noting that none of them focused specifically on machine-learning methods for the FX market from 2010 to 2021. Fletcher [ 18 ] concentrated on discriminative approaches (Support Vector Machines (SVM), Relevance Vector Machines (RVM), and Neural Networks) without examining other machine learning algorithms critically.
Islam et al. [ 27 ] took into account machine learning in the context of forex trading, highlighting Regression Methods, Optimization Techniques, SVM Method, Neural Network Chaos Theory, Pattern-based Methods, and Other Methods, but the period under consideration was from 2017 to 2019, but there has been a lot of work in this area over the last two decades. Panda et al. [ 44 ] concentrated on deep learning and hybrid techniques, as well as a few other machine learning algorithms, but the number of publications chosen was insufficient for the period under evaluation.
The research published by Sezer et al. [ 59 ] focused on deep learning research by looking at Convolutional Neural Networks (CNNs), Deep Belief Networks (DBNs), and Long-Short Term Memory (LSTM): however, there are other deep learning models such as Radial Basis Function, Multi-layer Perceptron, and many more deep learning methods that have been used in forex market prediction that were not looked at by the article.
Contributions
The following are the contributions by this SLR:
We took a critical look at 60 primary articles or studies that present machine learning forecasting models in the forex market. Researchers can use them as a beginning stage to expand the knowledge on the topic.
We give a comprehensive summary of the primary studies found. This section is divided into three sections: I machine learning methodologies, (ii) evaluation strategies, and (iii) performance analysis of the presented models.
Based on our findings, we offer guidance and recommendations to help further research in the field.
Research methodology
In this study, a systematic literature review was employed as a research approach since it is a defined and methodical way of discovering, evaluating, and studying existing material in order to investigate a certain research issue or phenomenon Barbara [ 5 ].
We followed the SLR rules that were proposed by Barbara [ 5 ]. In addition, we incorporated the process of systematic reference inclusion, often known as "snowballing," as outlined by [ 71 ].
We adopted the SLR guidelines proposed by [ 71 ].
The following subsections describe the process followed.
Search strategy
A technique was proffered to retrieve all published articles/papers/literature associated with the topic. Our primary studies retrieval techniques involve search terms and phrase identification, databases to be searched, search and paper picking strategy adopted for the study.
Identifying search terms and phrases
To find the relevant search terms and phrases we followed five steps outlined by Barbara [ 5 ]:
We found alternative spellings and/or synonyms for all major terms and phrases;
We double-checked the keywords in any important paper;
In cases where a database supports it, we employed Boolean operators for conjunction, such as the OR, AND operator for concatenation of major phrases and operator for concatenation of alternative spellings and synonyms If necessary, we combined the search string into a summary form.
Results for a). To better tailor our search keywords, we first defined the population, intervention, and outcome. Specifically:
Results for b). The alternative spellings and synonyms identified are:
Forex: (“forex “OR “foreign exchange”).
Market: (“Market “OR “trading”).
forecasting: (“forecasting” OR “predicting”)
Machine Learning: (“machine learning” OR “supervised learning” OR “classification” OR “regression” OR “unsupervised learning”);
Results for c). We searched through the keywords in the relevant papers/articles and couldn't come up with any further spellings or synonyms to add to the list of terms to consider.
Results for d). The following search query was created using Boolean operators:

Results for e). We also defined the short search string reported below, due to the IEEE Xplore digital library's search term limitation:

Resources searched
In an SLR, selecting appropriate resources to search for germane material is critical. All available literature relevant to our study topics was selected and searched using the following resources:
Google Scholar ( https://scholar.google.com )
IEEE Xplore digital library ( https://ieeexplore.ieee.org )
ScienceDirect ( https://www.sciencedirect.com )
Microsoft Academic ( https://academic.microsoft.com )
ACM digital library ( https://www.acm.org )
Our decision to use these databases was based on our desire to collect as many publications as possible in order to adequately conduct our systematic literature review.
Because they contain a massive amount of literature related to our research questions, such as journal articles, conference proceedings, books, and other materials, the chosen sources or databases are recognized as one of the most representative for Forex market forecasting research and are used in many other SLRs.
Article selection process
Figures 1 , 2 and 3 depicts the article selection procedure used in this study. The selection procedure is described in depth in the subsections that follow.
We gathered the main research in the digital libraries specified in “ Resources searched ” section using the search strings given in “ Identifying search terms and phrases ” section. The search technique was restricted by a chronological range, therefore publications from 2010 to 2021 were examined. Table 1 second column displays the search results generated by the digital libraries: As you can see, we downloaded a total of 120 documents related to the query.
We started by removing non-relevant publications from the complete list of retrieved sources using the exclusion criteria (detailed reported in “ Resources searched ” section). The exclusion criteria were applied after reading the title, abstract, and keywords of the 120 publications discovered. Table 1 shows data source and search results with 120 initial papers and 20 removed, leaving 100 for the second step of screening, which included the inclusion criteria. This approach yields our final search string, which consists of 60 articles (60 percent of the papers discovered in the previous stage).
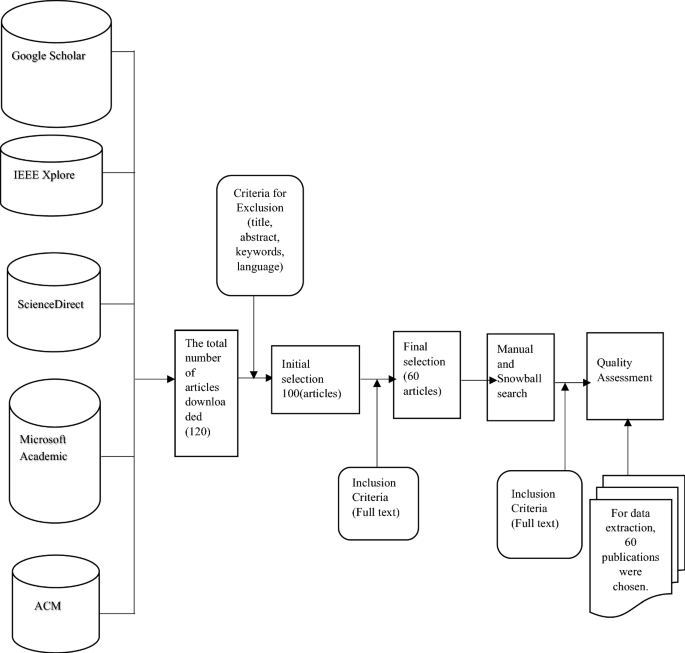
Showing conceptual framework for data extraction from the primary studies
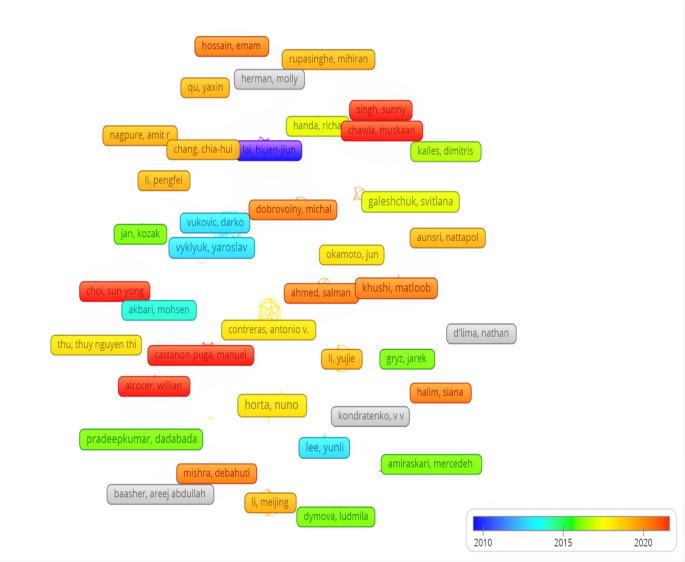
VOSviewer ( http://www.vosviewer.com/ ) was used to undertake a bibliographic coupling analysis. The number of references that are common across the publications evaluated is represented by the size and color of the nodes. The number of referenced references that two publications have in common is indicated by the link's strength
Point c should be critically looked at.
We employed a snowballing strategy to seek for possible missing papers [ 71 ]. The technique of identifying more sources using a paper's reference list or citations is known as snowballing [ 71 ]. In our context, we employed both forward and backward snowballing: with the former, we included articles that were referred to in the initially selected papers, and with the latter, we included publications that were referenced in the initially selected papers. We followed the same method as before, scanning the titles, abstracts, and keywords of the snowballed papers and applying the exclusion/inclusion criteria again: as a consequence, several publications were recognized as requiring further examination.
Given a collection of 60 sources identified using search string and then supplemented with those collected via snowballing. As a result, 60 papers made it through all of the stages.
We used the last filtering, i.e., the quality assessment step, after we had determined the set of final articles to evaluate in the SLR, to ensure that all of the final articles provided the data required to answer our research questions (see “ Evaluation of the study's quality ” section). As a result of this phase, we were able to compile the final list of articles for our study. Until this point, all 60 sources found had passed the quality check. As a result, our SLR is based on 60 studies. “Extraction of data” section details the data extraction procedure. The next subsections include (i) inclusion/exclusion criteria, (ii) the procedure's quality evaluation method, and (iii) the data extraction process.
Inclusion and exclusion criteria
An article has to meet the following criteria to be useful in answering our research questions.
A. Exclusion criteria: We omitted sources that satisfied the following limits from our research:
Articles about predicting methods other than machine learning.
Articles written in a language besides English.
Articles that aren't available in their whole.
B. Sources that complied with the following restrictions were considered for inclusion in our research:
All the articles, written in English, report machine learning techniques for forex market forecasting;
Articles that provide new strategies for improving the performance of existing machine learning algorithms for forecasting FX markets. It's worth noting that we included a wide range of publications (for example, journal, conference, workshop, and short papers) in order to compile a comprehensive collection of relevant resources.
Evaluation of the study's quality
After the final selection process, the quality of the publications was assessed. The legitimacy and completeness of the selected publications were assessed using the checklist below.
Q1: Does the machine learner classifier have a well-defined definition?
Q2: Can you tell me about the evaluation methodologies.
Consider the scenario in which research uses MAE, RMSE as an assessment statistic.
Extraction of data
We began extracting the data needed to address our study questions once we had chosen the final publications to be utilized for the SLR. We depended on the data in particular.
Table 2 shows the extraction form used in the selected publications, such as the machine learning algorithm.
Extraction of data form
Once we'd decided on the final papers for the SLR, we started extracting the data we'd need to answer our research questions. We used the data extraction form shown in Table 2 in particular. In addition to information on the specific aspects under examination, such as the machine learning approach employed in the selected publications, we have left aside a section for characterizing baseline models of the analyzed study. This made it easy to find a baseline model of prior research as well as future research direction.
Conceptual framework for data extraction
In this part, we offer a brief description of the demographics of the articles that passed the inclusion/exclusion criteria and the quality evaluation before providing the findings of the SLR with regard to the examined research topics.
Characteristics of the population
The final list of relevant primary studies evaluated in this SLR is presented in Tables 3 and 4 , with columns 'Year' and 'Book/Book Chapter/ Conf./Article/Thesis' indicating the year of publication and the periodicals in which the study was published. As can be seen, all of the articles evaluated were published between 2010 and 2021; 80% of this primary research were published after 2014, perhaps indicating a developing tendency that is now establishing itself as a more established subject. In conclusion We determined that the application of machine learning techniques for FX market prediction still poses open difficulties based on the quantity and types of papers released by the research community.
RQ1—Algorithm for machine learning
The first research question of our SLR was connected to the machine learners utilized in the literature, as identified in the primary papers. We wanted to know I whose machine learning method was used, (ii) what deep learning (Neural Network and related algorithms) was used, and (iii) what additional machine learning strategies were used. The outcomes of our analyses are detailed in the subsections below.
Beyond the 60 primary considered from 2010 to 2021, some papers published in 2022 as shown in Table 5 used machine learning algorithms including the authors publication were also looked at but did not affect the analysis of the initial 60 papers.
RQ2.3 Machine Learning algorithms
Our investigation revealed that a wide range of machine learning methods were applied. The bar chart in Fig. 4 displays the algorithms utilized in the primary investigations, as well as their frequency of presence. The machine learning method, the primary research in which it was utilized, and the number of primary studies that employed it are all listed in Table 6 . It's worth noting that many or hybrid algorithms may have been utilized in single primary research.
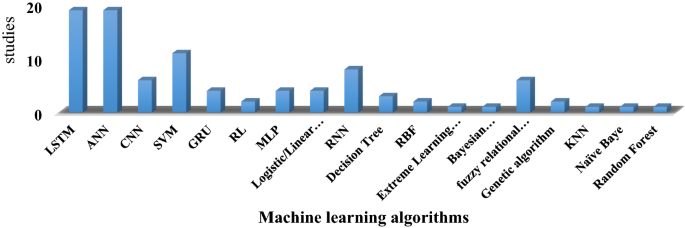
Bar chart reporting the number of primary studies and the machine learning algorithm adopted in each
LONG SHORT MEMORY NEURAL NETWORK (LSTM) as it is possible to these was investigated and used by 19 primary studies. A possible reason lies into the output of this type of models, which has shown a great performance and to properly analyze non-linear data, its ability to overcome the varnishing gradient problem pose by other neural networks.
ARTIFICIAL NEURAL NETWORK (ANN) as a deep learning model was also used by 19 primary studies indicating a growing trend in the use of it in the forex market prediction.
CONVOLUTIONAL NEURAL NETWORK is one of the deep learning algorithms that has not receive massive usage in the forex market, this model was use by 6 of our primary studies but can be further exploited in the foreign exchange market.
SUPPORT VECTOR MACHINE (SVA) was also used a number of times, 11 primary studies used this algorithm which is the third most used algorithm according to Figs. 4 , 5 , 6 and 7 . This classifier, as previously described by Farhat [ 17 ] can attain very high performance.
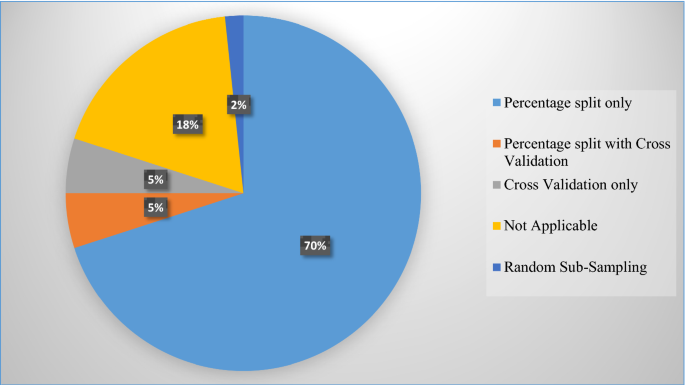
Validation approaches used in primary investigations are depicted in a pie chart
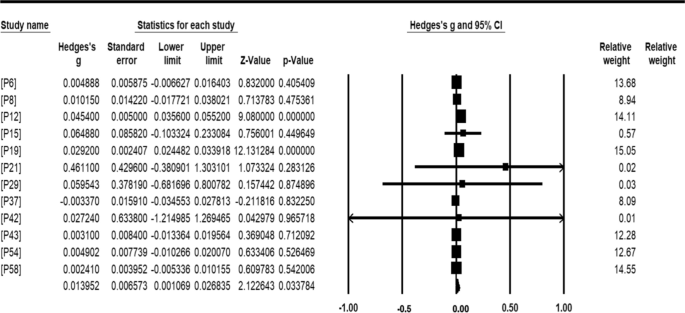
Results of meta-analysis based on MAE as Hedge's g and RMSE as Standard error
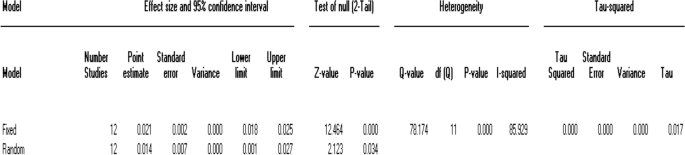
GRU stands for GATED RECURRENT UNIT and is a type of RNN with a gating mechanism. Compared to LSTMs, GRUs do not contain an output gate [ 57 ], four primary studies exploited this algorithm for their work.
REINFORCEMENT LEARNING (RL) this algorithm has not been exploited much as only two of our primary studies made use of it showing more opportunity in the use of this algorithm in the forex market.
MULTILAYER PERCEPTRON (MLP) is a feedforward artificial neural network (ANN) with three layers of nodes: input, hidden layer, and output layer. Except for the input nodes, each node in our selection is a neuron with a nonlinear activation function. Four key papers used this approach in their research.
LOGISTIC/LINEAR REGRESSION this machine learning algorithm was used by 4 of our primary studies, not popular in forex prediction.
RECURRENT NEURAL NETWORK Unlike traditional feedforward neural networks, RNNs have memory. Information fed into them persists, and the network can draw on this to make inferences. 8 papers from our primary studies used RNN for forex prediction.
DECISION TREE As may be seen, three key research studied Decision Trees. The output of these types of models, which consists of a rule describing the requirements for making a choice, could be one reason.
FUNCTION ON A RADIAL BASE NEURAL NETWORK (RBF) is a real-valued function whose value is solely determined by the distance between the input and a fixed point, such as the origin or another fixed point known as the center. This neural network was used by two of our key studies for their prediction models since any function that satisfies the property is a radial function.
EXTREME LEARNING MACHINES, BAYESIAN COMPRESSED VECTOR, K-NEAREST NEIGHBOR, NAÏVE BAYE AND RANDOM FOREST these are the least use algorithms according to our primary studies, from our studies we noticed that these algorithms were used in only one paper each showing the unpopularity in forex prediction.
FUZZY RELATIONAL MODEL was combined with other machine learning algorithms in predicting the forex market, 6 papers adopted this model in our primary studies.
The GENETIC ALGORITHM is a search heuristic based on Charles Darwin's natural selection hypothesis. This algorithm mimics the natural selection process, in which the most fit individuals are chosen for reproduction in order to create offspring for the following generation. It was combined with other machine learning models in predicting currency direction. 2 of our primary studies made us of this algorithm.
RQ2-dataset
Currency pairs are grouped into two thus the major and the minor, the major pairs are the four most heavily traded currency pairs in the forex (FX) market. The EUR/USD, USD/JPY, GBP/USD, and USD/CHF are the four major pairs at the moment. The EUR/USD is the most widely traded currency pair in the world, accounting for more than 20% of all forex transactions. The USD/JPY is a distant second, followed by the GBP/USD and, with a minor part of the global currency market, the USD/CHF. 75 percent of all forex trades are made on the big pairings. Minor currency pairs are ones which leave out the United States dollar, and they are normally less liquid. Examples include the euro and Swiss franc (EUR/CHF), Canadian dollar and Japanese yen (CAD/JPY), or pound sterling and Australian dollar (GBP/AUD etc.
Table 7 show the major currency pairs and corresponding primary papers/ articles that made use of dataset of them. From the table 42 papers made use of the EUR/USD currency pair and we can clearly say that according to our finding, it is the most traded and used currency pair. 18 papers made use of datasets of USD/GBP which is the next most traded pair after EUR/USD from our primary studies. USD/JPY came third as the next most used dataset from our study with 13 papers making use of its dataset. Last is USD/CHF dataset with 10 papers making use of it. From our study, we found out that some papers/articles made use of more than one dataset of the major currency pair. Some papers combined both minor and major pairs.
RQ3—evaluation setup
Our third research question revolves around the machine learning forecasting model evaluation strategies. It refers to:
validation techniques,
evaluation metrics adopted, and
datasets exploited.
RQ3.1—validation techniques
70% of the pie chart which is made up of 42 primary studies used percentage split only as a validation technique which is the most used validation technique in the 60 primary studies. 3 of the primary studies made use of percentage split and cross-validation representing 5% of the total primary studies. Articles that used cross-validation only represent 5% of the total primary studies which is just 3 articles. Some primary studies were designed as systems that did not make use of any validation techniques and that took 18% of the primary studies representing 11 papers or studies, one validation technique.
that came up only is Random sub-sampling validation that is just 2% of the total primary studies. Papers that used percentage split divided the dataset into two parts (Training and Test dataset) with the large part for training with the other part for the test. K-Fold cross-validation was used with K being set to 10. In this approach, the technique divides the original data set into ten equal-sized subsets at random. One of the ten subsets is kept as a test set, while the other nine are utilized as training sets. The cross-validation is then done ten times, with each of the ten subsets serving as the test set exactly once [ 26 ].
Table 8 shows evaluation metrics adopted by the primary studies, the most widely used are Mean Absolute Error (MAE), Mean Absolute Percentage Error (MAPE), Root Mean Square Error (RMSE), and Mean Square Error (MSE). Studies [P20], [P27], [P28], [P29], [P31], [P33], [P34], [36], [P37], [P43], [P44] adopted evaluations metrics that didn’t appear in any other primary paper aside them.
RQ4—meta-analysis of performance
The last research topic of our study was a statistical meta-analysis of machine learning algorithm performance in Forex market forecasting. A complete re-execution of the prediction models on a shared dataset with common assessment criteria would have been perfect for this sort of investigation, allowing them to be benchmarked. This, however, is not covered by a Systematic Literature Review; the purpose is to combine the findings of the major investigations. We looked at the effects of I the machine learning algorithm and (ii) the validation approach in this research. A statistical meta-analysis seeks to combine several studies to improve the estimates of impact sizes and clarify uncertainty when separate research disagrees. While individual studies are frequently insufficient to establish solid generalizable results, their combination may result in a lower random error and smaller confidence ranges. Meta-analyses have another significant flaw: they are unable to compensate for poor study design and bias in the individual research. If the studies are carried out and evaluated appropriately, the benefits vastly outweigh the minor drawbacks. We used the recommendations provided in the book to conduct the meta-analysis. In a meta-analysis, the first step is to calculate the result of interest and summary statistics from each of the individual studies. Not all prior research evaluated the performance of the presented models in the same way, as described in the evaluation metrics. To have a common baseline, we utilized Mean Square Error, Root Mean Square Error, and other metrics that were used in most of the other sources. To make a fair comparison, we looked at all of the publications and the metrics used. Papers that evaluated the effectiveness of machine learning model prediction in terms of Mean Absolute Error (MAE), Mean Absolute Percentage Error (MAPE), Root Mean Square Error (RMSE), and so on.
The analysis is based on twelve studies. The standardized difference in means (d) is the effect size index. The results of this analysis will be generalized to comparable studies. Therefore, the random-effects model was employed for the analysis. If we assume that the true effects are normally distributed (in d units), we can estimate the prediction interval. For all of the values in the primary studies, we utilized Comprehensive Meta-Analysis v3 to derive effect size estimates. It's worth mentioning that effect sizes must be normalized in order to be comparable across research. We used Hedges' g as the standardized measure of effect magnitude to achieve this goal. There are three main statistics recorded here which are the Q-value with its degrees and P-value, the I-square statistics, and finally Tau-square however these statistics don’t tell us how much the effect size varies. In our case the Q-value is 78.174, the degree of freedom is 12 and P-value is 0.0. The next statistic recorded is I-square which is 85.929 in our analysis. Finally, the last statistic recorded is Tau-square which is the variance of true effects in our case Tau-squared is 0.017. The prediction interval tells how much the test size varies here we will report that the true effect size is 0.05 and 0.95. In our meta-analysis, the mean effect size using the Hedge's g is 0.013952 with the Mean Absolute Error of each selected study using the Hedges g value. A 0.5 effect size indicates that the treatment group's mean is half a standard deviation higher than the control group's mean, the effect sizes imply that the mean of the treatment group is half a standard deviation higher than the mean of the control group (Table 9 ).
The application of machine learning techniques for forex market forecasting was the subject of a systematic literature review in this research. It focused on three particular features of how prior research performed experiments on forex forecasting models, namely I which machine learning model was used, (ii) which sorts of assessment procedures were used, and (iii) what validation approaches were used. Our research was based on publications that were published between 2010 and 2021. We looked at 60 publications out of a total of 120 that proposed machine learning algorithms for FX market forecasting. Existing studies were examined, as well as unresolved topics that need to be addressed in a future study. The research shows that deep learning models like ANN, LSTM, MLP-Neural Networks, and Radial Basis Function are more suited for forecasting the forex market than other machine learning algorithms like KNN and Nave Baye. The results are encouraging. To make the research field more precise, we have offered a list of steps to take. As a result, we conclude that the conclusions on the use of percentage split are suspect, and that the dataset should be divided into three parts: training, test, and validation test. In this regard, we hope that our findings may serve as a springboard for further investigation.
Availability of data and materials
The datasets used and/or analyzed which are the primary studies have been referenced in Table 2 column citation during the current study and are available from the corresponding author on reasonable request.
Acknowledgements
Grateful to the department of computer science and informatics university of energy and natural resources, to all individuals who assisted in one way or the other to see this work through to completion God bless you.
This research did not receive funding from any source.
Author information
Authors and affiliations.
University of Energy and Natural Resources, Sunyani, Ghana
Michael Ayitey Junior, Peter Appiahene, Obed Appiah & Christopher Ninfaakang Bombie
You can also search for this author in PubMed Google Scholar
MAJ searched through publication databases for the primary studies and also use inclusion and exclusion criteria to select the final studies for the research and assisted in performing the meta-analysis. PA played a supervisory role and assisted in designing data extraction form for retrieving interested information from the primary studies. OA analyzed and performed the bibliometric coupling for knowing the relationship that exists between the individual authors of each primary studies. CNB performed the meta-analysis of the twelve selected primary studies and reported the results. All authors read and approved the final manuscript.
Authors' information
MAJ is a master of philosophy in computer science student at the department of computer science and informatics at university of energy and natural resources at Sunyani Ghana. PA is the head of department for computer science and informatics at the university of energy and natural resources at Sunyani Ghana and direct supervisor to the corresponding author. OA co-supervisor to the main supervisor of the corresponding author and also coordinator for graduate studies of the department of computer science and informatics at university of energy and natural resources at Sunyani Ghana. CNB an assistant lecturer and a PHD candidate.
Corresponding author
Correspondence to Michael Ayitey Junior .
Ethics declarations
Ethics approval and consent to participate.
Not applicable.
Consent for publication
Competing interests.
The authors declare that they have no competing interests.
Additional information
Publisher's note.
Springer Nature remains neutral with regard to jurisdictional claims in published maps and institutional affiliations.

Rights and permissions
Open Access This article is licensed under a Creative Commons Attribution 4.0 International License, which permits use, sharing, adaptation, distribution and reproduction in any medium or format, as long as you give appropriate credit to the original author(s) and the source, provide a link to the Creative Commons licence, and indicate if changes were made. The images or other third party material in this article are included in the article's Creative Commons licence, unless indicated otherwise in a credit line to the material. If material is not included in the article's Creative Commons licence and your intended use is not permitted by statutory regulation or exceeds the permitted use, you will need to obtain permission directly from the copyright holder. To view a copy of this licence, visit http://creativecommons.org/licenses/by/4.0/ .
Reprints and permissions
About this article
Cite this article.
Ayitey Junior, M., Appiahene, P., Appiah, O. et al. Forex market forecasting using machine learning: Systematic Literature Review and meta-analysis. J Big Data 10 , 9 (2023). https://doi.org/10.1186/s40537-022-00676-2
Download citation
Received : 13 January 2022
Accepted : 25 December 2022
Published : 28 January 2023
DOI : https://doi.org/10.1186/s40537-022-00676-2
Share this article
Anyone you share the following link with will be able to read this content:
Sorry, a shareable link is not currently available for this article.
Provided by the Springer Nature SharedIt content-sharing initiative
- Systematic Literature Review
- Forex market
- Machine learning
- Meta-analysis
- Main navigation
- Main content
Government Intervention in the Foreign Exchange Market
- Owen F. Humpage
Working Papers of the Federal Reserve Bank of Cleveland are preliminary materials circulated to stimulate discussion and critical comment on research in progress. They may not have been subject to the formal editorial review accorded official Federal Reserve Bank of Cleveland publications. The views expressed in this paper are those of the authors and do not represent the views of the Federal Reserve Bank of Cleveland or the Federal Reserve System.
Suggested Citation
Humpage, Owen F. 2003. “Government Intervention in the Foreign Exchange Market.” Federal Reserve Bank of Cleveland, Working Paper No. 03-15. https://doi.org/10.26509/frbc-wp-200315
Foreign Exchange Swaps and Cross-Currency Swaps
Swiss Finance Institute Research Paper No. 22-51
A book chapter forthcoming in The Research Handbook of Financial Markets
31 Pages Posted: 16 Jun 2022
Angelo Ranaldo
University of Basel - Faculty of Business and Economics; Swiss Finance Institute; University of St. Gallen
Date Written: June 10, 2022
In the first part of this chapter, we explain the main characteristics of foreign exchange (FX) swap and cross-currency swap contracts. We emphasize the importance of the valuation adjustment (XVA) approach and then map the FX swap market in terms of currencies, parties, maturities, and size. The second part is devoted to the institutional framework of the FX swap market, specifically its over-the-counter (OTC) nature, recent technological trends, and policy actions such as the regulatory reform and process to oust the Libor rate. We conclude this chapter by pointing to future research directions to better understand the pricing and market functioning of FX swaps.
Suggested Citation: Suggested Citation
Angelo Ranaldo (Contact Author)
University of basel - faculty of business and economics ( email ).
Petersplatz 1 Basel, 4001 Switzerland
Swiss Finance Institute ( email )
c/o University of Geneva 40, Bd du Pont-d'Arve CH-1211 Geneva 4 Switzerland +41796637711 (Phone)
HOME PAGE: http://www.sfi.ch/de/about-us/news/hsg-faculty-members
University of St. Gallen ( email )
School of Finance Unterer Graben 21 St. Gallen, 9000 Switzerland +41712247010 (Phone)
HOME PAGE: http://fin-sr.unisg.ch
Do you have a job opening that you would like to promote on SSRN?
Paper statistics, related ejournals, swiss finance institute research paper series.
Subscribe to this free journal for more curated articles on this topic
Risk Management eJournal
Subscribe to this fee journal for more curated articles on this topic
Econometric Modeling: Derivatives eJournal
The global foreign exchange market in a higher-volatility environment
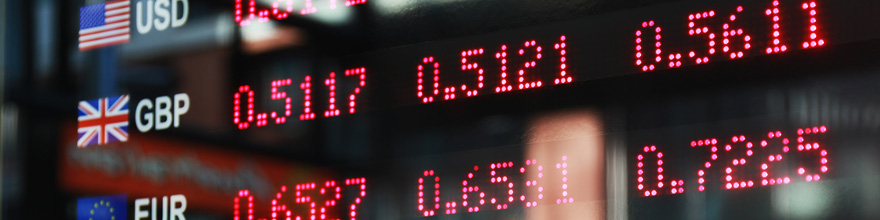
- Online appendix
- Data behind the graphs
Turnover in global foreign exchange (FX) averaged more than $7.5 trillion per day in April 2022 amid a volatile market environment. Compared with the previous BIS Triennial survey in 2019, trading volumes were higher because of greater activity in short-maturity FX derivatives and more inter-dealer trading. By contrast, trading with customers stagnated, mirroring a slowdown in international investment in 2022. A greater share of trading was executed via various bilateral methods, rather than via multilateral platforms that make prices available to all participants, implying that the transparency of the FX market may have decreased further. 1
JEL classification: C42, C82, F31, G12, G15.
Turnover in global foreign exchange (FX) markets reached $7.5 trillion per day in April 2022 ( Graph 1 , panel A), 2 a volume that is 30 times greater than daily global GDP. 3 The Triennial Central Bank Survey of over-the-counter (OTC) foreign exchange turnover ("Triennial Survey") offers a glimpse into this vast FX market. This year in April, data collection coincided with heightened FX volatility due to a confluence of factors, such as changing expectations about the paths of future interest rates in major advanced economies, rising commodity prices and geopolitical tensions after Russia's invasion of Ukraine.
Global FX volumes were higher compared with the previous Triennial Survey in 2019, owing to two main drivers. First, more trading in short maturity FX derivatives, which mechanically increases turnover, under the assumption that many contracts are rolled over. And the greater use of short maturity derivatives may reflect market participants' aversion to taking on term risk in a more volatile environment. Second, more inter-dealer trading, which tends to rise with volatility. In fact, the rise in inter-dealer turnover was big enough to reverse the long-term trend of a declining inter-dealer share in global FX trading. By contrast, dealers' trading with financial customers stagnated, mirroring the slowdown in international financial investment activity.
Key takeaways
- Turnover in global foreign exchange markets reached $7.5 trillion per day in April 2022, in a market that was more volatile than during the previous survey in 2019.
- Inter-dealer trading increased, reversing a long-run trend. By contrast, trading by dealers with customers stagnated, partly reflecting a slowdown in international investment activity.
- Trading moved further away from multilateral platforms towards bilateral methods, where information remains private, suggesting that the transparency of the FX market may have decreased further.
Trading with hedge funds and principal trading firms (PTFs), and the associated prime-brokered turnover, also declined, suggesting some reduction in activity by non-bank financial intermediaries in the FX market.
The share of FX trading using various bilateral methods, where information about the trade remains private, has increased. This reflects both inter-dealer and dealer-customer trading shifting away from multilateral platforms. In the inter-dealer market, trading volumes executed via electronic brokers, where trade attributes such as prices can be seen by all participants, have thus continued to decline. The notable shift towards bilateral forms of trading in 2022 implies a continued reduction of "visible" trading and increased market fragmentation, suggesting that the transparency of the FX market may have decreased further.
The remainder of the feature starts with a bird's eye view of long-run trends. This forms the backdrop for discussing the Triennial results obtained this year amid more volatile markets than during previous surveys. The last two sections delve deeper into the dealer-customer and inter-dealer market segments.
The global FX market – a bird's eye view of long-run trends
While more than 50 currencies trade globally, FX trading activity is concentrated in a few trading hubs and major currencies. The Triennial captures sales desk activity in 52 jurisdictions for 56 currencies. Yet, close to 80% of all FX trading takes place in the five FX trading hubs that are major financial centres. Furthermore, as the pre-eminent vehicle currency, the US dollar was on one side of around 90% of all FX trades in April 2022 (see Box A ), a share virtually unchanged for decades.
FX trading involves both spot and derivatives, with the share of spot having been on a gradual decline over the last 10 years ( Graph 1 , panel B). FX swaps are the most traded FX instrument and their share increased from around 40% in 2013 to more than 50% in 2022. They are typically used by market participants to take positions, manage funding liquidity in different currencies and hedge currency risk. 4 Forwards are the third most traded instrument, 5 used mainly to hedge currency risk or to bet on future currency movements. Their market share has edged up gradually over time.
The FX market can be broadly characterised as consisting of a dealer-customer and an inter-dealer segment. Such a two-tier structure is typical of OTC markets, where dealers warehouse risk and serve as counterparties, ie provide liquidity, to end users. Inter-dealer trading volumes used to exceed trading volumes with customers until about two decades ago due to inter-dealer trading of inventory imbalances. 6 Thereafter, various structural changes resulted in relatively less inter-dealer trading ( Graph 1 , panel C). Examples include more efficient inventory risk management and "internalisation", whereby dealers match customer flows on their own books.
But the distinction between the core inter-dealer and dealer-customer market segments has become somewhat blurred over time. This reflects a proliferation of multilateral trading venues, a growing variety of execution methods, and some non-bank actors emerging as liquidity providers alongside dealers. Especially in spot markets, principal trading firms (PTFs) have become important. PTFs rely mostly on speed and automated trading strategies rather than balance sheet capacity to support their market intermediation activities. As they have morphed into liquidity providers to customers, PTFs have become an integral part of FX intermediation and a key determinant of liquidity conditions.
Aggregate FX turnover in a higher volatility environment
Comparing the results of the 2022 Triennial with the 2019 Survey is complicated by very different market environments. The 2019 Triennial covered a month in a period of subdued and falling volatility in FX markets. And ultra-low interest rates supported intermediation and liquidity provision in FX markets. The 2022 environment looks very different. Especially important for the FX market this year was the high and uncertain inflation path, driving rapid but globally diverging monetary tightening. The Russian invasion of Ukraine led to further uncertainty and market turbulence. These developments had a profound effect on international capital flows and financial market volatility, including that in currency markets.
To get a better sense of the relationship of volatility and turnover in FX markets, we benchmark the Triennial with higher-frequency series. 7 This allows us to compare changes in turnover not only over three years but also on a month-by-month basis.
The higher-frequency benchmark shows that turnover increased in tandem with FX volatility in the early part of 2022 ( Graph 2 ). At close to $8.5 trillion per day, turnover in March 2022 is estimated to have exceeded even the prior peak during the Covid-19 financial market turmoil in March 2020. In July and August this year, some divergence between volatility and turnover emerged when exchange rate volatility continued to rise while turnover did not.
The positive relationship between turnover and volatility is in line with historical relationships and not too surprising ( Graph 2 , panel C). In a higher-risk environment, market participants tend to adjust positions, shift towards hedging currency risk, or take more speculative positions – activities that all drive up turnover. A dispersion of beliefs about the future among investors will also drive trading volumes higher. 8
That said, trading in spot did not outpace that in derivatives, as it did in previous high-volatility episodes. The monthly benchmark series spot and derivatives volumes show that, historically, it was spot trading that increased faster with volatility than derivatives trading. This is especially visible during the Covid-19 crisis in March 2020. Hence, the positive relationship of changes in volatility with changes in the ratio of spot to derivative turnover ( Graph 3 , panel A). This year, however, both spot and derivatives trading grew in tandem so that their ratio remained unchanged (red dots). This may reflect that in early 2022 market participants adjusted to changing expectations of a prolonged period of uncertainty.
The higher turnover in FX derivatives may reflect the increasing use of short maturities. A shift towards shorter maturities increases turnover mechanically, under the assumption that many contracts are rolled over. The more granular but lower-frequency data from the Triennial shows that the growth of turnover in FX swaps and forwards between 2019 and 2022 was due entirely to more trading in shorter tenors with maturities of one week or less ( Graph 3 , panels B and C). And the overnight segment, first split out in 2022, accounted for close to half of short-maturity swaps and almost a third of short-maturity forwards. As inter-dealer FX swap trading is predominantly in very short tenors, the higher inter-dealer share in 2022 is one factor behind the overall shortening of maturities.
Dealers' trading with customers stagnates
At the broadest level, customers to reporting dealers are categorised into two groups. First, non-financial customers, such as global corporates, which make up only a small and shrinking fraction of dealer-customer turnover. 9 Second, other financial institutions – a customer group that includes non-reporting banks, hedge funds and PTFs, institutional investors, and official sector financial institutions.
Further reading :
- FX trade execution: complex and highly fragmented (bis.org)
- FX execution algorithms and market functioning (bis.org)
- The Foreign Exchange Market (SSRN)
Dealers' trading with customers has stagnated in dollar terms compared with three years ago ( Graph 4 , panel A). Given the overall growth of the market, the share of dealer-customer turnover therefore fell from 62% to 54%. Stagnant customer trading may partly reflect the risk-off environment. Contraction in international investment in early 2022 likely played a role as gross external portfolio positions contracted sharply in the first quarter of 2022. 10 And whenever international investment positions have fallen historically, FX market turnover with other financial institutions has fallen too. 11
Across customer segments, there was a small shift away from trading with hedge funds and PTFs. Turnover with this segment declined both in spot and derivatives. Hence, the share of hedge funds and PTFs in total turnover with other financial institutions declined from 17% to 14% ( Graph 4 , panel B). The associated prime-brokered turnover declined as well ( Graph 4 , panel C). Given high volatility, market sources suggest that hedge funds have been very active in April this year. Hence, the decline is most likely to reflect less turnover with PTFs. The Euromoney magazine rankings also show that the PTF share in (direct electronic) spot liquidity provision to clients has declined, from 32% to 28% between 2019 and 2022. The relatively more muted PTF activity could reflect a range of factors such as a reported shift by some PTFs from highly competitive and efficient FX markets to less efficient asset classes with greater arbitrage opportunities, such as crypto, dealers catching up technologically or the factors underpinning the resurgent inter-dealer market as discussed next.
A resurgent inter-dealer market
Reversing the long-run trend decline, the share of inter-dealer trading has grown significantly over the last three years, to account for more than 45% of all FX trading volumes in April 2022 ( Graph 5 , panel A). Such a share was last seen in the mid-2000s (see Graph 1 , panel B). Inter-dealer activity gained relative ground across all three major instruments, to 40% in spot, 54% in FX swaps and 34% in outright forwards ( Graph 5 , panel B).
The relative rise in inter-dealer trading volumes may partly reflect the more volatile environment. Higher volatility means greater need to offset inventory imbalances with other dealers and to engage in other risk management trades.
Less inter-dealer trading is public
The inter-dealer market provides crucial information for ascertaining market conditions and obtaining reference prices for the wider FX market. The centralised structure of the electronic inter-dealer spot market has meant that this core activity and information was contained within two main inter-dealer electronic brokers (see Box B ). These are organised as central limit order books (CLOBs), where information such as quantities and prices is visible to traders on the platform. And analogous to an exchange, trading on these multilateral venues is anonymous, ie the counterparty is unknown (hence they are classified as "anonymous venues" in the Triennial). 12 This contrasts with many other venues in the FX market, which trade by "direct" means. In these cases, dealers, for instance, respond bilaterally to requests from customers or stream prices electronically to specific customers. Hence, conditions of trades executed by direct means are known only to the two counterparties (see Box C ).
The 2022 Triennial shows a further decline of trading on inter-dealer electronic brokers – a continuation of a long-run trend ( Graph 5 , panel C). Even as inter-dealer spot trading increased by 43% compared with 2019, the share of inter-dealer spot turnover traded via electronic brokers declined further, from 15% to 9%. Lower spot volumes on inter-dealer electronic brokers also go hand in hand with lower PTF trading activity, as PTFs account for a significant amount of trading activity on inter-dealer brokers. 13
Several drivers are behind the trend decline in inter-dealer electronic brokers. First, more inter-dealer trading via disclosed and direct means, as, for example, in the fact that smaller dealers increasingly source liquidity from big dealers for their own customers in this form. Second, internalisation, whereby dealers match customer flows on their own books, continues to substitute for inter-dealer trading (Butz and Oomen (2018)). While major FX dealers have been internalising most of their customer flows for a long time, the recent Triennial shows a further increase in internalisation ratios, particularly on the part of dealers in Asian financial centres ( Graph 6 , panel A). 14 Third, related-party trades, ie trades between different organisational units of a single dealer, which have continued to rise to 22% of total turnover ( Graph 6 , panel B). 15 Relatedly, the 2022 Triennial first broke out "non-market-facing" trades. These amounted to $895 billion, and their shares in the different instruments ranged between 20 and 35% of inter-dealer turnover ( Graph 6 , panel C). 16 While internalisation and non-market-facing trades seem rather technical factors, in many cases they represent alternative choices to "going to the market", where they would have affected market conditions.
While less "visible" trading and greater market fragmentation have so far not hampered market functioning, this development may not be without downsides. Market participants have successfully used technologies to navigate a more fragmented market. For example, customers rely on smart order routing and execution algorithms to spread large orders over time and across multiple electronic venues (Markets Committee (2020)). Yet, less visibility for trades could harm price discovery for the market as a whole. It also hinders policymakers from appropriately monitoring FX markets (see Markets Committee (2018)).
Bank for International Settlements (2022): " Triennial Central Bank Survey of Foreign Exchange and Over-the-counter (OTC) Derivatives Markets in 2022 ", October.
Bech, M (2012): " FX volume during the financial crisis and now ", BIS Quarterly Review , March.
Borio, C, R McCauley and P McGuire (2022): " Dollar debt in FX swaps and forwards: huge, missing and growing ", BIS Quarterly Review , December.
Butz, M and R Oomen (2019): "Internalisation by electronic FX spot dealers", Quantitative Finance, vol 19, no 1, pp 35–56.
Caballero, J, P Wooldridge and D Xia (2022): " The internationalisation of EME currency trading ", BIS Quarterly Review , December.
Chaboud, A, D Rime and V Sushko (2022): "The foreign exchange market", in R Gurkaynak and J Wright (eds), The Research Handbook of Financial Markets , available on SSRN.
Euromoney (2022): Foreign Exchange Survey 2022 .
Gopinath, G (2015): "The international price system", NBER Working Papers , no 21646.
Lyons, R (1996): "Foreign exchange volume: Sound and fury signifying nothing?", in J Frankel, G Galli and A Giovannini (eds), The Microstructure of Foreign Exchange Markets , University of Chicago Press.
Markets Committee (2018): " Monitoring fast-paced electronic markets ", report submitted by a Study Group, Markets Committee Papers , no 10.
---(2020): " FX execution algorithms and market functioning ", report submitted by a Study Group, Markets Committee Papers , no 13.
Moore, M, A Schrimpf and V Sushko (2016): " Downsized FX markets: causes and implications ", BIS Quarterly Review , December.
Ranaldo, A (2022): "Foreign exchange swaps and cross-currency swaps", Swiss Finance Institute Research Papers , no 22-51.
Schrimpf, A and V Sushko (2019): " Sizing up global foreign exchange markets ", BIS Quarterly Review , December.
Varian, H (1985): "Divergence of opinion in complete markets: a note", Journal of Finance , vol 40, no 1, pp 309–17.
Online Appendix
Technical annex.
Graph 1: Adjusted for local and cross-border inter-dealer double-counting, ie "net-net" basis; daily averages in April.
Graph 2.A: Triennial Survey data are adjusted for local and cross-border inter-dealer double-counting, ie "net-net" basis; daily averages in April. See Bech (2012) for a methodological description of the benchmarking technique. Vertical lines indicate the BIS Triennial Surveys in April of 2016, 2019 and 2022.
Graph 2.B: Options price based Deutsche Bank US dollar currency volatility index (DBCVIX) average mid-price. Vertical lines indicate the BIS Triennial Surveys in April 2016, 2019 and 2022.
Graph 2.C: Triennial Survey data are adjusted for local and cross-border inter-dealer double-counting, ie "net-net" basis; daily averages in April. See Bech (2012) for a methodological description of the benchmarking technique.
Graph 3A: FX spot/derivatives based on volumes settled via CLS.
Graph 3.B: Adjusted for local and cross-border inter-dealer double-counting, ie "net-net" basis. One-day data were not collected in previous Triennial surveys.
Graph 3.C: Adjusted for local and cross-border inter-dealer double-counting, ie "net-net" basis. One-day data were not collected in previous Triennial surveys.
Graph 4: FX turnover adjusted for local and cross-border inter-dealer double-counting, ie "net-net" basis; daily averages in April.
Graph 4.C: Prime-brokered turnover includes both (i) the transactions between prime brokers and their customers as well as (ii) the resulting "give-up" trades with reporting dealers. The associated customer turnover is anywhere between a half and two thirds of the total prime-brokered turnover. See Box A in Schrimpf and Sushko (2019a).
Graph 5: Adjusted for local and cross-border inter-dealer double-counting, ie "net-net" basis; daily averages in April.
Graph 6: Adjusted for local and cross-border inter-dealer double-counting.
Graph 6.A: Volume-weighted averages using total customer spot turnover (for countries that did not report the underlying customer spot turnover, their share in total spot turnover was applied). Asian centres include Hong Kong SAR, Japan and Singapore.
Graph 6.C: Based on back-to-back and compression trades.
1 We thank Ryan Banerjee, Claudio Borio, Alain Chaboud, Stijn Claessens, Philippe Lintern, Benoît Mojon, James O'Connor, Frank Packer, Andreas Schrimpf, Hyun Song Shin, Nikola Tarashev and Goetz von Peter for useful comments. Jose Maria Vidal Pastor provided excellent research assistance. All errors are our own. The views expressed in this article are those of the authors and not necessarily those of the Bank for International Settlements.
2 See Bank for International Settlements (2022a) for detailed survey results.
3 Global GDP amounted to $96.1 trillion in 2021 or $0.26 trillion per day; see World Bank, World Development Indicators database, 1 July 2022.
4 Borio et al (in this issue) discuss the use of FX swaps as funding instrument in greater detail.
5 Outright forwards include deliverable forwards and as well non-deliverable forwards (NDFs).
6 Lyons (1996) coined the term "hot potato trading" in reference to the repeated passing of inventory imbalances among dealers. A single customer trade could generate a cascade of inter-dealer trades until the inter-dealer market would settle at a new equilibrium price.
7 The benchmarking follows Bech (2012) and complements the Triennial mainly with semiannual surveys of local FX committees and monthly data from the Continuous Linked Settlement Group.
8 The impact of disagreement on trading volume has been appreciated at least since Varian (1985).
9 The share of non-financial customers in total FX turnover fell from 7% to 6% between 2019 and 2022.
10 Data from the IMF Coordinated Portfolio Investment Survey (CPIS) show that gross (assets plus liabilities) international portfolio positions of residents in a sample of 25 advanced and emerging market economies contracted by $7 trillion between Q4 2021 and Q1 2022.
11 Combining the IMF CPIS data with the BIS Triennial, we find a significant positive relationship between international investment portfolios and FX turnover, when regressing the three-year changes in FX turnover with other financial institutions in a currency of a specific jurisdiction on three-year changes in gross external portfolios (assets plus liabilities) of residents in that jurisdiction. Retrenchment of foreign portfolio investment also explains a lower level of turnover in EME currencies (Caballero et al (2022), in this issue).
12 The inter-dealer FX swap market is organised differently from spot. It has been highly fragmented for a long time; there is no anonymous trading in FX swaps, with electronic trading executed mainly via a "request-for-quote" trading protocol (see Ranaldo (2022)).
13 Chaboud et al (2022) show that non-bank (eg PTF) trading volumes, executed algorithmically, accounted for more than 40% of all trading on EBS in mid-2021.
14 Data on internalisation ratios are likely to be less precise than the turnover figures as they are collected as a supplementary question.
15 A variety of transactions can be related-party trades, such as an FX desk of a dealer bank conducting a risk transfer trade with its headquarters, which does consolidated risk management globally; a derivatives desk hedging risk resulting from a trade with a spot desk of the same institution; or a local affiliate raising foreign currency funding by entering an FX swaps transaction with its headquarters
16 The Survey collected back-to-back and compression trades as part of non-market-facing trades. Back-to-back trades are deals that automatically follow trades with customers to shift risk across the sales desks of a dealer. Compression trades refer to portfolio optimisation by replacing existing contracts with new ones to reduce notional amounts while keeping net exposures unchanged.
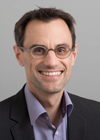
- Share this page
- Sign up to receive email alerts
- Translations
- Legal information
- Terms and conditions
- Copyright and permissions
- Privacy notice
- Cookies notice
- Email scam warning
A business journal from the Wharton School of the University of Pennsylvania
How Carry Trade Exposure Impacts Asset Price Movements
September 10, 2024 • 7 min read.
Research co-authored by Wharton’s Amy Wang Huber introduces a new metric to measure the risk-bearing capacity of foreign exchange market intermediaries and the implications for asset prices.
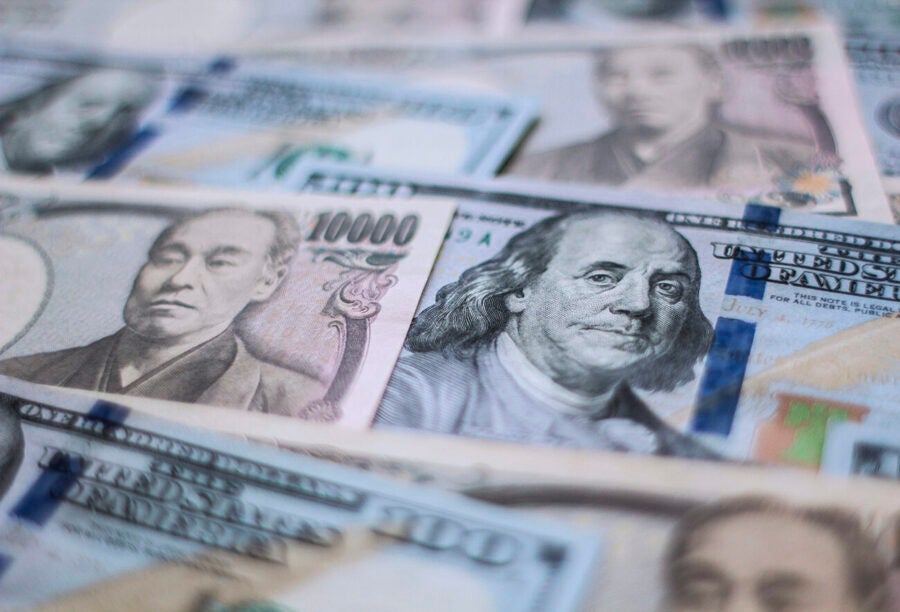
- Finance & Accounting
The spectacular unwinding of the Yen carry trade raises the question: How big is the carry trade ? This is a difficult question to answer. Foreign-exchange traders can simultaneously buy and sell multiple currencies. Exposures in one currency can be quickly hedged or diversified by trading other currencies. In other words, just tallying who traded which currency will not reveal the true extent of carry trades. Not all sales of Japanese yen indicate positions in a carry trade, for example.
Finance professors Amy Wang Huber at Wharton and Yu An at Johns Hopkins University propose to use risk to measure the extent of carry trade exposure in the financial system.
In their new research paper titled “ Intermediary Elasticity and Limited Risk-bearing Capacity ,” Huber and An developed a method to sort foreign exchange (FX) trades into those that cannot be further diversified and thus create risks to dealers and hedge funds that accommodate these trades. They identified “intermediary elasticity” as a way to measure the price compensation financial intermediaries require to bear such trading-induced risks. This intermediary elasticity, they argued, reflects financial intermediaries’ limited risk-taking capacity and shows why trading demand shocks in one currency propagate to other currencies and even other asset markets.
Measuring Risks and Their Price Response
In their paper, Huber and An showed that almost all FX trading-induced risks fall into three categories: the U.S. dollar portfolio, the carry portfolio, and the euro-yen portfolio. Together, these three risks account for 90% of the risk borne by intermediaries in FX trading. The U.S. dollar portfolio is one where investors buy the dollar and sell all other currencies. In the euro-yen portfolio, investors sell the Japanese yen and buy the euro. Finally, the carry portfolio buys all currencies of countries with high-interest rates and sells those of low-interest rate countries; essentially, it exploits arbitrage opportunities between currencies.
These risks capture what is non-diversifiable when dealers and hedge funds accommodate FX trades. Therefore, net flows into these risks measure the intermediaries’ trading-induced risk exposure. For example, the authors found that intermediaries accumulated $0.8 trillion in risk exposure to the carry trade between 2012 and 2023.
Why is it important to measure non-diversifiable risks in FX trading? Huber offered an example: The currencies of Australia and New Zealand tend to move similarly because these two countries share similar economic conditions. Therefore, if some investors buy Australian dollars and sell New Zealand dollars, the risks faced by intermediaries who accommodate these trades are largely diversified away.
“The amount of risk that the intermediary needs to absorb is not the same as the amount of securities traded.” — Amy Wang Huber
Huber stressed that because much of FX trading can be diversified, it is more meaningful to measure price movements in response to changes in the quantity of risk than changes in the quantity of currencies traded. This is the motivation behind intermediary elasticity, which offers an intuitive way to measure the intermediary’s risk-bearing capacity. If the price response to trading-induced risks is zero, then the intermediary is able to perfectly share risks from accommodating trading and the question of limited risk-bearing capacity does not arise. Conversely, the larger the intermediary elasticity, the more limited the risk-bearing capacity.
With intermediary elasticity, it is easy to uncover the impact of trading demand shock in one currency on other currencies or even other asset markets, Huber said. “It all comes down to common risk exposure,” she explained. “When a customer sells a large quantity of Japanese yen, the intermediary who accommodates this trade must hedge out the non-diversifiable risks induced by this trade. This hedge affects the risk price according to the intermediary elasticity. Then, all other currency prices are affected because each currency has some exposure to these non-diversifiable risks.” The same logic uncovers how trading demand shocks in FX propagate to other asset markets.
Highlights from the Paper
The study used a sample of foreign exchange transactions between intermediaries, such as dealers and hedge funds, and their customers from September 2012 to December 2023. The sample covered 17 currencies, including the U.S. dollar, the Australian dollar, the Canadian dollar, and the Korean won. There are three types of customers: funds, which include mutual funds, pension funds, and sovereign wealth funds; non-bank financial entities, including insurance companies and clearing houses; and corporations.
The study estimated the intermediary elasticity for each of the three risks — the dollar, the euro-yen, and the carry. Using sovereign bond auctions in the U.S., Australia, the U.K., Canada, Japan, Germany, and France, the study estimated that intermediaries raise the price by about 1% in response to a $20 billion trading demand shock to the dollar risk, a $10 billion trading demand shock to the carry risk, and a $3.5 billion trading demand shock to the euro-yen factor. Huber explained why the price responses are so large: “We are talking about shock to risks that are non-diversifiable risk. Price responses to trading demand shock in a single security could be much more muted because dealers can diversify across securities. In other words, the amount of risk that the intermediary needs to absorb is not the same as the amount of securities traded. This is the whole point of the paper.”
For the six commonly traded currencies of advanced economies — the Australian dollar, the Canadian dollar, the Swiss franc, the euro, the British pound, and the Japanese yen, the study determined that for every $1 billion in inflows, the price response ranges from 5 bps (basis points) to 16 bps.
“All asset markets are connected. Trading demand shocks originating from the FX market could spread to other asset markets, and vice versa.” — Amy Wang Huber
The study found large cross-elasticity between the Australian dollar, the Canadian dollar, and the British pound. That is, when there is a trading demand shock in the Australian dollar, the Canadian dollar’s price also moves. In contrast, the cross-elasticity is small between the Japanese yen and the Australian dollar, for example, because these two currencies hedge each other in terms of the carry risk exposure.
Finally, the paper showed that common exposure to non-diversifiable FX risks causes spillovers in asset markets such as CDS (credit default swap indices), commodities, corporate bonds, equities, options, and U.S. Treasury bonds. The study estimated that a $1 billion trading demand shock in corporate bonds raises its own price by 5 bps and that for CDS and commodities by similar magnitudes. In contrast, trading demand shocks to U.S. Treasury bonds reduce the price of CDS and corporate bonds.
Three Surprising Findings
The study produced surprises on three fronts, which Huber listed:
- The first is that the intermediary elasticity, or the price response to shocks to non-diversifiable risks, is very high.
- Secondly, the pattern of how currencies are related, particularly the relationship between the yen and the euro. “Both of them are low-interest rate currencies, but they are actually opposite each other in one of the key non-diversifiable risks that we identify,” Huber said.
- Thirdly, the study showed and quantified how trading in the forex market could affect prices in six other asset markets. “All asset markets are connected. Trading demand shocks originating from the FX market could spread to other asset markets, and vice versa.” Huber said.
For regulators, the big takeaway from the paper is that regulations are best designed vis-à-vis the exposure to the amount of risk, as opposed to simply counting the number of securities in a portfolio, Huber said.
More From Knowledge at Wharton
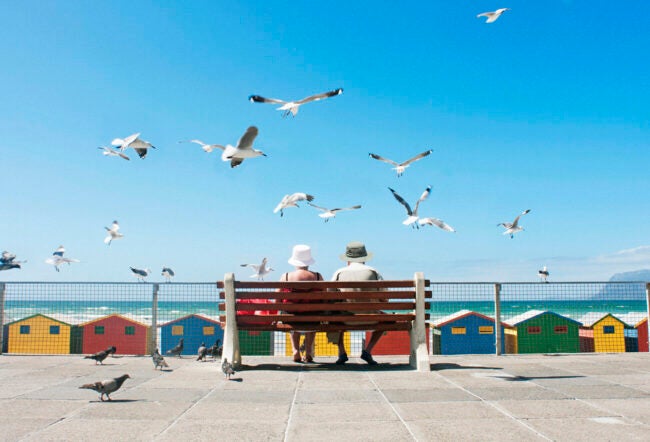
How Low-income Households Can Secure Their Retirement Finances
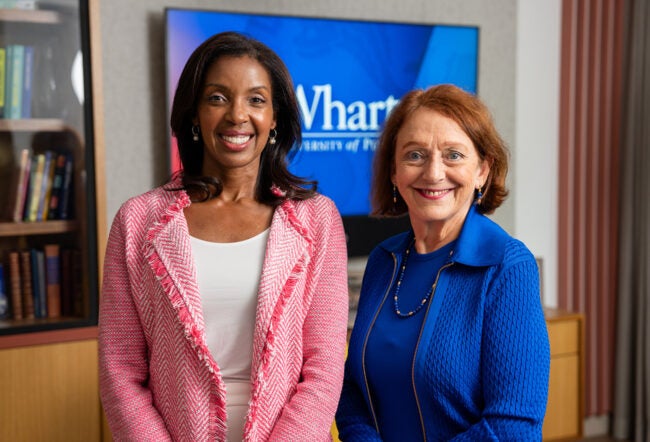
What I’ve Learned: Olivia S. Mitchell
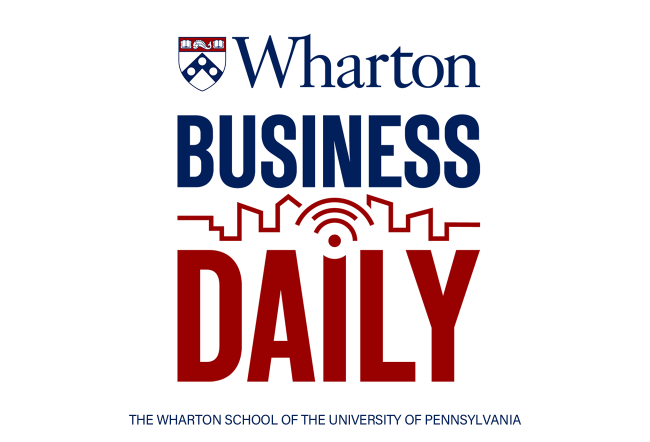
Understanding Climate Risk’s Impact on Homeowners Insurance Premiums
Looking for more insights.
Sign up to stay informed about our latest article releases.

IMAGES
VIDEO
COMMENTS
1. Introduction. The foreign exchange market, which is a part of the global financial market, is important in regulating international capital flows and promoting the sustainable development of international economy and trade This exchange market is an important mechanism that regulates the relationship between the supply and demand of foreign exchange and that determines exchange rates in the ...
Although modern technical analysis was originally developed in the context of the stock market, its advocates. argue that it applies in one form or another to all asset markets. Since the era of floating exchange rates began in the. early 1970s, foreign currency traders have widely adopted this approach to trading.
This chapter discusses the structure and functioning of the spot foreign exchange (FX) market. The market structure, which has become far more complex over the past three decades, has mostly evolved endogenously as the global FX market is subject to notably less regulatory oversight than equity and bond markets in most countries.
The foreign exchange or forex market is the largest financial market in the world where currencies are bought and sold simultaneously. It is even larger than the stock market; According to the 2019 Triennial Central Bank Survey of FX and Over-the-Counter (OTC) derivatives markets, it has a daily volume of $6.6 trillion [].It is a non-centralized market that operates 24 h a day except the ...
Abstract. Foreign exchange market is the largest financial market in the world and the value of activities in currency of one country is used to trade for the unit of currency of another country. There are four main participants in FX market such as 1) companies and individuals 2) capital market 3) Hedger 4) Speculators This research exploring ...
Research Department, Norges Bank, Oslo, Norway. Norwegian University of Science and Technology, Norway. Search for more papers by this author. Michael R. King, Michael R. King. Richard Ivey School of Business, University of Western Ontario, Canada ... This chapter describes the foreign exchange (FX) market and presents new evidence on recent ...
Abstract Nowadays foreign exchange interventions occur in emerging market economies, whereas empirical studies on interventions mainly refer to advanced economies. ... more research on interventions in emerging markets is needed analysing the influence of heterogeneous institutional circumstances, examining the role of central bank ...
Keywords: foreign exchange intervention, exchange rate, Chinese economy, inflation Author's E-Mail Address: [email protected]; [email protected]; [email protected] . IMF Working Papers. describe research in progress by the author(s) and are published to elicit comments and to encourage debate. The views expressed in IMF Working Papers are
Abstract. The paper documents the use of foreign exchange intervention (FXI) across countries and monetary regimes, with special attention to its use under inflation targeting (IT). We find significant differences between advanced and emerging market economies, with the former group conducting FXI limitedly and broadly symmetrically, while the ...
BIS Papers No 24 97 The effectiveness of foreign exchange intervention in emerging market countries1 Piti Disyatat and Gabriele Galati 1. Introduction This paper attempts to provide a comprehensive overview of what is known about the effectiveness of foreign exchange intervention in emerging market countries. This is done in two steps. First, an
The paper uses event study methodology given the clustered and non-stochastic nature of intervention. We find that direct intervention, through sale or purchase of foreign currency, is not successful in influencing the direction of exchange rate movement and does not have any statistically significant impact on volatility as corroborated by ...
The foreign exchange (forex) market is a dynamic and complex financial arena where the exchange rates of various currency pairs fluctuate continuously. ... The main aim of this research paper is ...
F. oreign exchange intervention is the practice of monetary authorities buying and selling currency in the foreign exchange market to influence exchange rates. Researchers have studied whether interven- tion is successful in influencing exchange rate movements and how it affects volatility.
Keywords: financial markets, foreign exchange, market microstructure, dealer intermediation, electronic trading, algorithmic trading. BIS Working Papers are written by members of the Monetary and Economic Department of the Bank for International Se ttlements, and from time to time by other economists, and are published by the Bank.
Foreign exchange markets are often the most active and important asset markets in developing and transition economies, yet few research papers on the subject have systematically documented their structures or main characteristics.2 To bridge this gap, this paper characterizes the foreign exchange market microstructures in these economies with
This article offers a survey of the literature on foreign exchange intervention, including sections on the theoretical channels through which intervention might affect exchange rates and a summary of the empirical findings. The survey emphasizes that intervention is intended to provide monetary authorities with an means of influencing their exchange rates independent from monetary policy, and ...
In the first part of this chapter, we explain the main characteristics of foreign exchange (FX) swap and cross-currency swap contracts. We emphasize the importance of the valuation adjustment (XVA) approach and then map the FX swap market in terms of currencies, parties, maturities, and size.
By way of currency devaluation and/or depreciation on the foreign exchange market, those countries using their domestic currencies increased the price competitiveness and exports of their national economies, while at the same time reducing imports. ... Complementary to the research findings the paper's scientific contribution is composed ...
Turnover in global foreign exchange markets reached $7.5 trillion per day in April 2022, in a market that was more volatile than during the previous survey in 2019. Inter-dealer trading increased, reversing a long-run trend. By contrast, trading by dealers with customers stagnated, partly reflecting a slowdown in international investment activity. Trading moved further away from multilateral ...
Abstract and Figures. This paper analyses the impact of foreign exchange rates on the foreign exchange reserves and the real Gross Domestic Product in India. These variables are essential for ...
In their new research paper titled "Intermediary Elasticity and Limited Risk-bearing Capacity," Huber and An developed a method to sort foreign exchange (FX) trades into those that cannot be ...